Papers by Roozbeh Razavi-Far
The performance of diagnostic systems based on empirical models may vary in different zones of th... more The performance of diagnostic systems based on empirical models may vary in different zones of the training space. It is, thus, important to a-priori verify whether the model is working in a zone where the performance is expected to be satisfactory. In this respect, the objective of this work is to estimate the degree of confidence in the identification of nuclear transients by a diagnostic system based on a bagged ensemble of Supervised Fuzzy C-Means (FCM) classifiers. The method has been applied for classifying simulated transients in the feedwater system of a nuclear Boiling Water Reactor (BWR). The obtained results indicate that the bagging ensemble permits to achieve satisfactory performance, with a reliable estimation of the degree of confidence in the classification.
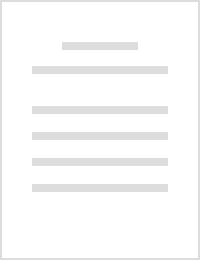
Neural Networks for Fault Detection and Isolation of a Nonlinear Dynamic System
The proper and timely fault detection and isolation of industrial plant is of premier importance ... more The proper and timely fault detection and isolation of industrial plant is of premier importance to guarantee the safe and reliable operation of industrial plants. The paper presents application of a neural networks-based scheme for fault detection and isolation, for the pressurizer of a PWR nuclear power plant. The scheme is constituted by two components: residual generation and fault isolation. The first component generates residuals via the discrepancy between measurements coming from the plant and a nominal model. The neural network estimator is trained with healthy data collected from a full-scale simulator. For the second component detection thresholds are used to encode the residuals as bipolar vectors which represent fault patterns. These patterns are stored in an associative memory based on a recurrent neural network. The proposed fault diagnosis tool is evaluated on-line via a full-scale simulator to detect and isolate the main faults appearing in the pressurizer of a PWR.
Ensemble of neural networks for detecting and classifying faults in nuclear power systems
Optimal Detection of New Classes of Faults by an Invasive Weed Optimization Method
International Joint Conference on Neural Networks (IJCNN), Jul 2014
Invasive Weed Classification
Efficient residuals pre-processing for diagnosing multi-class faults in a doubly fed induction generator, under missing data scenarios
Dynamic learning of latent residuals for diagnosing new class drifts in wind turbines
Transactions of the American Nuclear Society, Nov 2013
Smart Innovation, Systems and Technologies
This paper presents a study and the obtained results on the performance optimization of a large r... more This paper presents a study and the obtained results on the performance optimization of a large refrigeration system in steady state conditions. It is shown that, by using adequate knowledge on plant operation, the plant wide performance can be optimized with respect to a small set of variables. For this purpose, an appropriate performance function is defined. A derivative free optimization technique based on the invasive weed optimization (IWO) algorithm has been used to optimize the parameters of the local controllers in the system. The performance of the IWO algorithm, both in terms of optimality of the results and speed of convergence, is compared with particle swarm optimization (PSO) algorithm. Simulation results have been used to validate the proposed approach.
Plant-wide performance optimisation - The refrigeration system case
IEEE International Conference on Control Applications, CCA 2012, Oct 2012
Plant-wide dynamic and static optimisation of supermarket refrigeration systems
Optimising performance in steady state for a supermarket refrigeration system
20th Mediterranean Conference on Control and Automation, MED 2012, Jul 2012
A multiple observers and dynamic weighting ensembles scheme for diagnosing new class faults in wind turbines
Incremental design of a decision system for residual evaluation: A wind turbine application
8th IFAC Symposium on Fault Detection, Supervision and Safety of Technical Processes, SAFEPROCESS 2012, Aug 2012
Dynamic weighting ensembles for incremental learning and diagnosing new concept class faults in power systems
Ensemble of neural networks for detection and classification of faults in power systems
World Scientific Proc. Series on Computer Engineering and Information Science 7; Uncertainty Modeling in Knowledge Engineering and Decision Making, Aug 2012
Reliability Engineering & System Safety, Apr 2011
An important requirement for the practical implementation of empirical diagnostic systems is the ... more An important requirement for the practical implementation of empirical diagnostic systems is the capability of classifying transients in all plant operational conditions. The present paper proposes an approach based on an ensemble of classifiers for incrementally learning transients under different operational conditions. New classifiers are added to the ensemble where transients occurring in new operational conditions are not satisfactorily classified. The construction of the ensemble is made by bagging; the base classifier is a supervised Fuzzy C Means (FCM) classifier whose outcomes are combined by majority-voting. The incremental learning procedure is applied to the identification of simulated transients in the feedwater system of a Boiling Water Reactor (BWR) under different reactor power levels.
Annals of Nuclear Energy, 2011
This paper presents an ensemble-based scheme for nuclear transient identification. The approach a... more This paper presents an ensemble-based scheme for nuclear transient identification. The approach adopted to construct the ensemble of classifiers is bagging; the novelty consists in using supervised Fuzzy C-Means (FCM) classifiers as base classifiers of the ensemble. The performance of the proposed classification scheme has been verified by comparison with a single supervised, evolutionary-optimized FCM classifier with respect of the task of classifying artificial datasets. The results obtained indicate that in the cases of datasets of large or very small sizes and/or complex decision boundaries, the bagging ensembles can improve classification accuracy. Then, the approach has been applied to the identification of simulated transients in the feedwater system of a Boiling Water Reactor (BWR).

Neurocomputing, 2009
This paper presents a neuro-fuzzy (NF) networks based scheme for fault detection and isolation (F... more This paper presents a neuro-fuzzy (NF) networks based scheme for fault detection and isolation (FDI) of a U-tube steam generator (UTSG) in a nuclear power plant. Two types of NF networks are used. A NF based learning and adaptation of Takagi-Sugeno (TS) fuzzy models is used for residual generation, while for residual evaluation a NF network for Mamdani models is used. The NF network for Takagi-Sugeno models is trained with data collected from a full scale UTSG simulator and is used for generating residuals in the fault detection step. A locally linear neuro-fuzzy (LLNF) model is used in the identification of the steam generator. This model is trained using the locally linear model tree (LOLIMOT) algorithm. In the fault isolation part, genetic algorithms are employed to train a Mamdani type NF network, which is used to classify the residuals and take the appropriate decision regarding the actual behavior of the process. Furthermore, a qualitative description of faults is then extracted from the fuzzy rules obtained from the Mamdani NF network. Experimental results presented in the final part of the paper confirm the effectiveness of this approach.
Neuro-fuzzy based fault diagnosis of a Steam Generator
7th IFAC Symposium on Fault Detection, Supervision and Safety of Technical Processes, SAFEPROCESS 2009, Jul 2009
Uploads
Papers by Roozbeh Razavi-Far