Papers by Thomas Wettergren

arXiv (Cornell University), Sep 9, 2019
This paper presents a rapid (real time) solution to the minimum-time path planning problem for Du... more This paper presents a rapid (real time) solution to the minimum-time path planning problem for Dubins vehicles under environmental currents (wind or ocean currents). Realtime solutions are essential in time-critical situations (such as replanning under dynamically changing environments or tracking fast moving targets). Typically, Dubins problem requires to solve for six path types; however, due to the presence of currents, four of these path types require to solve the root-finding problem involving transcendental functions. Thus, the existing methods result in high computation times and their applicability for realtime applications is limited. In this regard, in order to obtain a real-time solution, this paper proposes a novel approach where only a subset of two Dubins path types (LSL and RSR) are used which have direct analytical solutions in the presence of currents. However, these two path types do not provide full reachability. We show that by extending the feasible range of circular arcs in the LSL and RSR path types from 2π to 4π: 1) full reachability of any goal pose is guaranteed, and 2) paths with lower time costs as compared to the corresponding 2π-arc paths can be produced. Theoretical properties are rigorously established, supported by several examples, and evaluated in comparison to the Dubins solutions by extensive Monte-Carlo simulations. Index Terms-Dubins paths, path planning, environmental currents, curvature-constrained vehicles.
In a multi-objective optimization scenario (e.g., optimal sensor deployment and sensor field reco... more In a multi-objective optimization scenario (e.g., optimal sensor deployment and sensor field reconfiguration for detection of moving targets), the non-dominated points are usually concentrated within a small region of the largedimensional decision space. This paper attempts to capture the low-dimensional behavior across the Pareto front by statistical mechanics-inspired optimization tools. A location-dependent energy function has been constructed and evaluated in terms of intensive temperature-like parameters in the sense of statistical mechanics. This low-order representation has been shown to permit rapid optimization of sensor field distribution on a simulation model of undersea operations.

Proceedings of SPIE, Apr 27, 2007
In a sparse sensor network, the sensor detection regions are often not overlapped. The traditiona... more In a sparse sensor network, the sensor detection regions are often not overlapped. The traditional instantaneous detection scheme is less effective due to the fact that targets may not be detected by any sensors at certain sampling instances. To detect the moving targets in a sparse sensor network, we have developed a new system suitable for multiple targets detection and tracking. An optimization based random field estimation method has been developed to characterize spatially distributed sensor reports without making any assumptions of their underlying statistical distributions. FBMM (Forward & Backward Mapping Mitigation) technology is developed to reduce the false detections resulted from the random field estimation. To further reduce the false detections, the refined random field is clustered using gap statistics. STLD (Spatial & Temporal Layering Discrimination) method is developed to individual clusters and true sensor detections are determined based on both spatial and temporal patterns. Simulation results have shown that our system can effectively detect multiple target tracks in a large surveillance region.

IEEE transactions on cybernetics, Jun 1, 2018
This paper presents information-theoretic performance analysis of passive sensor networks for det... more This paper presents information-theoretic performance analysis of passive sensor networks for detection of moving targets. The proposed method falls largely under the category of data-level information fusion in sensor networks. To this end, a measure of information contribution for sensors is formulated in a symbolic dynamics framework. The network information state is approximately represented as the largest principal component of the time series collected across the network. To quantify each sensor's contribution for generation of the information content, Markov machine models as well as x-Markov (pronounced as cross-Markov) machine models, conditioned on the network information state, are constructed; the difference between the conditional entropies of these machines is then treated as an approximate measure of information contribution by the respective sensors. The x-Markov models represent the conditional temporal statistics given the network information state. The proposed method has been validated on experimental data collected from a local area network of passive sensors for target detection, where the statistical characteristics of environmental disturbances are similar to those of the target signal in the sense of time scale and texture. A distinctive feature of the proposed algorithm is that the network decisions are independent of the behavior and identity of the individual sensors, which is desirable from computational perspectives. Results are presented to demonstrate the proposed method's efficacy to correctly identify the presence of a target with very low false-alarm rates. The performance of the underlying algorithm is compared with that of a recent data-driven, feature-level information fusion algorithm. It is shown that the proposed algorithm outperforms the other algorithm.
Replicator dynamics of evolutionary games with different delays on costs and benefits
Applied Mathematics and Computation, Dec 1, 2023
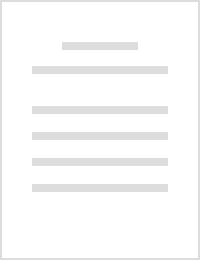
Optimization of waterspace management and scheduling for multiple unmanned vehicles
Institution of Engineering and Technology eBooks, Jul 8, 2020
We describe an approach for accomplishing the high-level mission planning required for a heteroge... more We describe an approach for accomplishing the high-level mission planning required for a heterogeneous team of autonomous vehicles performing surveys in multiple areas, such as required for mine countermeasure (MCM) missions. The high-level mission scheduling and waterspace management require sequencing the order and location of lower-level tasks to be completed by each vehicle in the heterogeneous team: unmanned surface vessels (USVs) and unmanned underwater vehicles (UUVs). In this context, the USVs serve as transport vehicles while the UUVs perform the actual surveys and execute any other local actions. We develop a solution to this complex sequencing operation by leveraging unique information processing, communication, refueling, and planning windows that form constraints within the system within a formal scheduling optimization framework. We use mixed-integer linear programming (MILP) as a solution method for the associated optimization problem. By using such a standard optimization approach, we both take advantage of optimality guarantees on the solution and extensive commercial numerical solvers. In addition to developing the numerical optimization problem, we also include methods to account for risks due to schedule slip for the individual tasks. We conclude with an example of joint USV/UUV planning using the optimization algorithm

arXiv (Cornell University), Sep 9, 2019
This paper presents a rapid (real time) solution to the minimum-time path planning problem for Du... more This paper presents a rapid (real time) solution to the minimum-time path planning problem for Dubins vehicles under environmental currents (wind or ocean currents). Realtime solutions are essential in time-critical situations (such as replanning under dynamically changing environments or tracking fast moving targets). Typically, Dubins problem requires to solve for six path types; however, due to the presence of currents, four of these path types require to solve the root-finding problem involving transcendental functions. Thus, the existing methods result in high computation times and their applicability for realtime applications is limited. In this regard, in order to obtain a real-time solution, this paper proposes a novel approach where only a subset of two Dubins path types (LSL and RSR) are used which have direct analytical solutions in the presence of currents. However, these two path types do not provide full reachability. We show that by extending the feasible range of circular arcs in the LSL and RSR path types from 2π to 4π: 1) full reachability of any goal pose is guaranteed, and 2) paths with lower time costs as compared to the corresponding 2π-arc paths can be produced. Theoretical properties are rigorously established, supported by several examples, and evaluated in comparison to the Dubins solutions by extensive Monte-Carlo simulations. Index Terms-Dubins paths, path planning, environmental currents, curvature-constrained vehicles.
Sensors, Jun 28, 2023
This article is an open access article distributed under the terms and conditions of the Creative... more This article is an open access article distributed under the terms and conditions of the Creative Commons Attribution (CC BY
OCEANS 2022, Hampton Roads, Oct 17, 2022
We propose a novel method to generate underwater object imagery that is acoustically compliant wi... more We propose a novel method to generate underwater object imagery that is acoustically compliant with that generated by side-scan sonar using the Unreal Engine. We describe the process to develop, tune, and generate imagery to provide representative images for use in training automated target recognition (ATR) and machine learning algorithms. The methods provide visual approximations for acoustic effects such as back-scatter noise and acoustic shadow, while allowing fast rendering with C++ actor in UE for maximizing the size of potential ATR training datasets. Additionally, we provide analysis of its utility as a replacement for actual sonar imagery or physics-based sonar data.

ACM Transactions on Sensor Networks, Nov 25, 2020
The paper presents a distributed algorithm, called Prediction-based Opportunistic Sensing for Res... more The paper presents a distributed algorithm, called Prediction-based Opportunistic Sensing for Resilient and Efficient Sensor Networks (POSE.R), where the sensor nodes utilize predictions of the target's positions to probabilistically control their multi-modal operating states to track the target. There are two desired features of the algorithm: energy-efficiency and resilience. If the target is traveling through a high node density area, then an optimal sensor selection approach is employed that maximizes a joint cost function of remaining energy and geometric diversity around the target's position. This provides energy-efficiency and increases the network lifetime while preventing redundant nodes from tracking the target. On the other hand, if the target is traveling through a low node density area or in a coverage gap (e.g., formed by node failures or non-uniform node deployment), then a potential game is played amongst the surrounding nodes to optimally expand their sensing ranges via minimizing energy consumption and maximizing target coverage. This provides resilience, that is the self-healing capability to track the target in the presence of low node densities and coverage gaps. The algorithm is comparatively evaluated against existing approaches through Monte Carlo simulations which demonstrate its superiority in terms of tracking performance, network-resilience and network-lifetime.
Planning Searches Using an Orienteering-based Vehicle Routing Approach
OCEANS 2022, Hampton Roads
Estimation of Multi-objective Optimal Solutions for Hypothetical Systems: A Probabilistic Step Towards the Verification of Autonomous Systems
OCEANS 2022, Hampton Roads
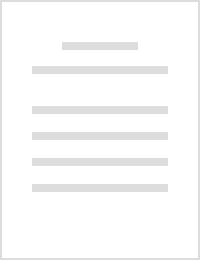
A search game for optimizing information collection in UUV mission planning
OCEANS 2015 - MTS/IEEE Washington, 2015
This paper addresses search planning to find hidden objects in undersea environments where the se... more This paper addresses search planning to find hidden objects in undersea environments where the sensor detection process is subject to false alarms with a geographically varying likelihood. We develop a game-theoretic approach for maximizing the information flow that occurs as a multi-agent collaborative search is conducted over a bounded region. To accomplish this, we apply 1) a search channel formalism to the discrete search paradigm to formulate information measures as a function of searcher regional visitation and 2) a Receiver Operator Characteristic (ROC) analysis to map search strategies to ROC operating points which, in turn, define the properties of the search channel. This enables us to formulate a search game where the cost is driven by expended search effort and the payout is in terms of the information collected. We describe a new algorithmic capability for solving the search allocation problem of multiple unmanned undersea vehicles that can collaborate in their efforts to resolve false alarm outcomes. We discuss the properties of the information measure developed for a repeated look over the search channel and examine the impact of setting ROC operating points to their game equilibrium values. Doing so enables the regional visitation to be determined via a greedy scheduling solution. Results are presented to show the dominance of this approach over alternative ROC strategies and to demonstrate the capability to take advantage of known non-homogeneity in the search environment.
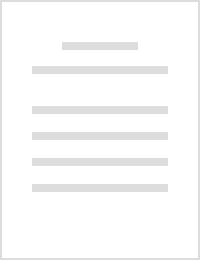
Guest Editorial Special Issue on Control of Very-Large-Scale Robotic (VLSR) Networks
IEEE Transactions on Control of Network Systems, 2021
Future applications of autonomous systems promise to involve increasingly large numbers of collab... more Future applications of autonomous systems promise to involve increasingly large numbers of collaborative robots individually equipped with onboard sensors, actuators, and wireless communications. By sharing and coordinating information, plans, and decisions, these very-large-scale robotic (VLSR) networks can dramatically improve their performance and operate over long periods of time with little or no human intervention. Controlling many collaborative agents to this day presents significant technical challenges. Besides requiring satisfactory communications, the amount of computation associated with most coordinated control algorithms increases with the number of agents. It is well-known, for example, that the optimal control of N collaborative agents for path planning and obstacle avoidance is a PSPACE-hard problem. Also, while necessary for performing basic tasks such as localization and mapping, many sensing and estimation approaches suffer from the curse of dimensionality, and their performance may degrade as uncertainties from disparate sources propagate through the network.
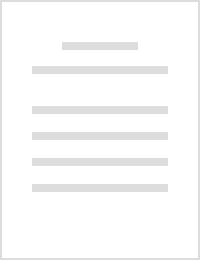
Extended search games for UUV mission planning
OCEANS 2017 – Anchorage, 2017
This paper discusses the construction of search games as a planning method to optimize the effect... more This paper discusses the construction of search games as a planning method to optimize the effectiveness of UUV search agents. In our previous work, we applied a receiver operator characteristic (or ROC curve) to model the detection performance of search agents operating in adverse environments where false alarms are prevalent. The area search game was developed to maximize the information collected during the search operation. This was achieved for fixed search horizon planning cycles by jointly optimizing the distribution of search effort over the region and the setting of ROC operating points affecting the characteristics of the local search channels. In this paper, we discuss extensions to this game to address the determination of search horizon. We articulate the expanded strategy space that occurs in multi-agent problems where the fusion of information is explicitly included within the search paradigm. The information content of search outcomes are examined and an information ...
Classification induced distributed sensor scheduling for energy-efficiency in underwater target tracking sensor networks
OCEANS 2017 – Anchorage, 2017
This paper develops a distributed sensor scheduling methodology that utilizes target classificati... more This paper develops a distributed sensor scheduling methodology that utilizes target classification decisions to govern the number of active sensors selected around the target in the deployment region. This approach utilizes a distributed supervisor on each sensor node to control the multi-modal operating state of the node. A distributed sensor selection method is proposed that dynamically adjusts the number of active sensor nodes based on the classification decision. The proposed method is simulated and validated to show that incorporating classification into the control loop significantly conserves energy reserves while still allowing for accurate target estimation.
Replicator dynamics of an N-player snowdrift game with delayed payoffs
Applied Mathematics and Computation, 2021
Abstract We consider a version of the N -player snowdrift game in which the payoffs obtained by t... more Abstract We consider a version of the N -player snowdrift game in which the payoffs obtained by the players are delayed. The delay in payoffs is shown to lead to a Hopf bifurcation with an associated critical value of the time delay in the replicator dynamics. For time delays larger than the critical time delay, the replicator dynamics oscillate around the equilibrium instead of asymptotically approaching it. The dependence of this critical time delay on the parameters of the game is determined. After developing the analysis for the 2-player game, the same methodology is applied to the N -player version of the game to show how the results change as a function of N , concluding with an analysis of the limiting case of large numbers of players.
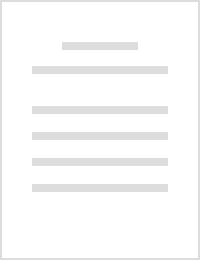
Risk-based scheduling of multiple search passes for UUVs
Detection and Sensing of Mines, Explosive Objects, and Obscured Targets XXI, 2016
This paper addresses selected computational aspects of collaborative search planning when multipl... more This paper addresses selected computational aspects of collaborative search planning when multiple search agents seek to find hidden objects (i.e. mines) in operating environments where the detection process is prone to false alarms. A Receiver Operator Characteristic (ROC) analysis is applied to construct a Bayesian cost objective function that weighs and combines missed detection and false alarm probabilities. It is shown that for fixed ROC operating points and a validation criterion consisting of a prerequisite number of detection outcomes, an interval exists in the number of conducted search passes over which the risk objective function is supermodular. We show that this property is not retained beyond validation criterion boundaries. We investigate the use of greedy algorithms for distributing search effort and, in particular, examine the double greedy algorithm for its applicability under conditions of varying criteria. Numerical results are provided to demonstrate the effectiveness of the approach.

IEEE Transactions on Control of Network Systems, 2019
This paper presents a distributed algorithm, called Prediction-based Opportunistic Sensing using ... more This paper presents a distributed algorithm, called Prediction-based Opportunistic Sensing using Distributed Classification, Clustering and Control (POSE.3C), for self adaptation of sensor networks for energy management. The underlying 3C network autonomy concept enables utilization of the target classification information to form dynamic clusters around the predicted target position via selection of sensor nodes with the highest energies and maximum geometric diversity. Further, the nodes can probabilistically control their heterogeneous devices to track targets of interest and minimize energy consumption in a completely distributed manner. Theoretical properties of the POSE.3C network are established and derived in terms of the network lifetime and missed detection characteristics. The algorithm is validated through extensive simulations which demonstrate a significant increase in the network lifetime as compared to other network control approaches, while providing high tracking accuracy and low missed detection rates.

Global Oceans 2020: Singapore – U.S. Gulf Coast
In this paper, we develop a new algorithm, called T-Lite, that enables fast time-risk optimal mot... more In this paper, we develop a new algorithm, called T-Lite, that enables fast time-risk optimal motion planning for variable-speed autonomous vehicles. The T-Lite algorithm is a significantly faster version of the previously developed T algorithm. T-Lite uses the novel time-risk cost function of T ; however, instead of a grid-based approach, it uses an asymptotically optimal sampling-based motion planner. Furthermore, it utilizes the recently developed Generalized Multi-speed Dubins Motion-model (GMDM) for sample-to-sample kinodynamic motion planning. The sample-based approach and GMDM significantly reduce the computational burden of T while providing reasonable solution quality. The sample points are drawn from a four-dimensional configuration space consisting of two position coordinates plus vehicle heading and speed. Specifically, T-Lite enables the motion planner to select the vehicle speed and direction based on its proximity to the obstacle to generate faster and safer paths. In this paper, T-Lite is developed using the RRT * motion planner, but adaptation to other motion planners is straightforward and depends on the needs of the planner.
Uploads
Papers by Thomas Wettergren