Papers by Wisal Hashim Abdulsalam

Engineering, Technology & Applied Science Research, 2025
Significant advances in the automated glaucoma detection techniques have been made through the em... more Significant advances in the automated glaucoma detection techniques have been made through the employment of the Machine Learning (ML) and Deep Learning (DL) methods, an overview of which will be provided in this paper. What sets the current literature review apart is its exclusive focus on the aforementioned techniques for glaucoma detection using the Preferred Reporting Items for Systematic Reviews and Meta-Analysis (PRISMA) guidelines for filtering the selected papers. To achieve this, an advanced search was conducted in the Scopus database, specifically looking for research papers published in 2023, with the keywords "glaucoma detection", "machine learning", and "deep learning". Among the multiple found papers, the ones focusing on ML and DL techniques were selected. The best performance metrics obtained using ML recorded in the reviewed papers, were for the SVM, which achieved accuracies of 98.31%, 98.61%, 96.43%, 96.67%, 95.24%, and 98.60% in the ACRIMA, REFUGE, RIM-ONE, ORIGA-light, DRISHTI-GS, and sjchoi86-HRF databases, respectively, employing the REFUGE-trained model, while when deploying the ACRIMA-trained model, it attained accuracies of 98.92%, 99.06%, 98.27%, 97.10%, 96.97%, and 96.36%, in the same databases, respectively. The best performance metrics obtained utilizing DL recorded in the reviewed papers, were for the lightweight CNN, with an accuracy of 99.67% in the Diabetic Retinopathy (DR) and 96.5% in the Glaucoma (GL) databases. In the context of non-healthy screening, CNN achieved an accuracy of 99.03% when distinguishing between GL and DR cases. Finally, the best performance metrics were obtained using ensemble learning methods, which achieved an accuracy of 100%, specificity of 100%, and sensitivity of 100%. The current review offers valuable insights for clinicians and summarizes the recent techniques used by the ML and DL for glaucoma detection, including algorithms, databases, and evaluation criteria.

Mesopotamian journal of Cybersecurity, 2025
The Internet of Things (IoT) is an expanding domain that can revolutionize different industries. ... more The Internet of Things (IoT) is an expanding domain that can revolutionize different industries. Nevertheless, security is among the multiple challenges that it encounters. A major threat in the IoT environment is spoofing attacks, a type of cyber threat in which malicious actors masquerade as legitimate entities. This research aims to develop an effective technique for detecting spoofing attacks for IoT security by utilizing feature-importance methods. The suggested methodology involves three stages: preprocessing, selection of important features, and classification. The feature importance determines the most significant characteristics that play a role in detecting spoofing attacks. This is achieved via two techniques: decision tree (DT) and mutual information (MI). For classification, adaptive boosting (AdaBoost), XGBoost and categorical boosting (CatBoosting) are used to categorize incoming data as normal or spoofing. The experimental results indicate the efficiency of the suggested approach for correctly identifying spoofing attacks with high accuracy, fewer false positives, and reduced time needed. By utilizing feature importance and robust classification algorithms, the system can accurately differentiate between legitimate and malicious IoT traffic, thereby improving the overall security of IoT networks. The CatBoost classifier outperformed the AdaBoost and XGBoost classifiers in terms of accuracy.
Research Square (Research Square), Mar 13, 2024
Zenodo (CERN European Organization for Nuclear Research), Nov 1, 2018

Wasit Journal of Pure sciences
The COVID-19 pandemic has necessitated new methods for controlling the spread of the virus, and m... more The COVID-19 pandemic has necessitated new methods for controlling the spread of the virus, and machine learning (ML) holds promise in this regard. Our study aims to explore the latest ML algorithms utilized for COVID-19 prediction, with a focus on their potential to optimize decision-making and resource allocation during peak periods of the pandemic. Our review stands out from others as it concentrates primarily on ML methods for disease prediction.To conduct this scoping review, we performed a Google Scholar literature search using "COVID-19," "prediction," and "machine learning" as keywords, with a custom range from 2020 to 2022. Of the 99 articles that were screened for eligibility, we selected 20 for the final review.Our systematic literature review demonstrates that ML-powered tools can alleviate the burden on healthcare systems. These tools can analyze significant amounts of medical data and potentially improve predictive and preventive healthcare.

2018 1st Annual International Conference on Information and Sciences (AiCIS), 2018
Recognizing speech emotions is an important subject in pattern recognition. This work is about st... more Recognizing speech emotions is an important subject in pattern recognition. This work is about studying the effect of extracting the minimum possible number of features on the speech emotion recognition (SER) system. In this paper, three experiments performed to reach the best way that gives good accuracy. The first one extracting only three features: zero crossing rate (ZCR), mean, and standard deviation (SD) from emotional speech samples, the second one extracting only the first 12 Mel frequency cepstral coefficient (MFCC) features, and the last experiment applying feature fusion between the mentioned features. In all experiments, the features are classified using five types of classification techniques, which are the Random Forest (RF), k-Nearest Neighbor (k-NN), Sequential Minimal Optimization (SMO), NaĂŻve Bayes (NB), and Decision Tree (DT). The performance of the system validated over Surrey Audio-Visual Expressed Emotion (SAVEE) dataset for seven emotions. The results of the experiments showed given good accuracy compared with the previous studies using a fusion of a few numbers of features with the RF classifier.
International Journal of Machine Learning and Computing, 2019

Iraqi Journal of Science, 2024
Attention-Deficit Hyperactivity Disorder (ADHD), a neurodevelopmental disorder affecting millions... more Attention-Deficit Hyperactivity Disorder (ADHD), a neurodevelopmental disorder affecting millions of people globally, is defined by symptoms of hyperactivity, impulsivity, and inattention that can significantly affect an individual's daily life. The diagnostic process for ADHD is complex, requiring a combination of clinical assessments and subjective evaluations. However, recent advances in artificial intelligence (AI) techniques have shown promise in predicting ADHD and providing an early diagnosis. In this study, we will explore the application of two AI techniques, K-Nearest Neighbors (KNN) and Adaptive Boosting (AdaBoost), in predicting ADHD using the Python programming language. The classification accuracies obtained were 96.5% and 93.47%, respectively, before applying balancing to the data. In addition, 98.59% and 97.18%, respectively, after applying the balancing technique The extreme gradient boosting (XGBoost) technique had been applied to selecting the important features and the Pearson correlation for finding the correlation between features.

Signature verification involves vague situations in which a signature could resemble many referen... more Signature verification involves vague situations in which a signature could resemble many reference samples or might differ because of handwriting variances. By presenting the features and similarity score of signatures from the matching algorithm as fuzzy sets and capturing the degrees of membership, non-membership, and indeterminacy, a neutrosophic engine can significantly contribute to signature verification by addressing the inherent uncertainties and ambiguities present in signatures. But type-1 neutrosophic logic gives these membership functions fixed values, which could not adequately capture the various degrees of uncertainty in the characteristics of signatures. Type-1 neutrosophic representation is also unable to adjust to various degrees of uncertainty. The proposed work explores the type-2 neutrosophic logic to enable additional flexibility and granularity in handling ambiguity, indeterminacy, and uncertainty, hence improving the accuracy of signature verification systems. Because type-2 neutrosophic logic allows the assessment of many sources of ambiguity and conflicting information, decision-making is more flexible. These experimental results show the possible benefits of using a type-2 neutrosophic engine for signature verification by demonstrating its superior handling of uncertainty and variability over type-1, which eventually results in more accurate False Rejection Rate (FRR) and False Acceptance Rate (FAR) verification results. In a comparison analysis using a benchmark dataset of handwritten signatures, the type-2 neutrosophic similarity measure yields a better accuracy rate of 98% than the type-1 95%.

Artificial Intelligence Techniques to Identify Individuals through Palm Image Recognition, 2024
Artificial intelligence (AI) is entering many fields of life nowadays.
One of these fields is bio... more Artificial intelligence (AI) is entering many fields of life nowadays.
One of these fields is biometric authentication. Palm print recognition
is considered a fundamental aspect of biometric identification systems
due to the inherent stability, reliability, and uniqueness of palm print
features, coupled with their non-invasive nature. In this paper, we
develop an approach to identify individuals from palm print image
recognition using Orange software in which a hybrid of AI methods:
Deep Learning (DL) and traditional Machine Learning (ML) methods are used to enhance the overall performance metrics. The system
comprises of three stages: pre-processing, feature extraction, and feature classification or matching. The SqueezeNet deep learning model
was utilized to resize images and feature extraction. Finally, different
ML classifiers have been tested for recognition based on the extracted
features. The effectiveness of each classifier was assessed using various performance metrics. The results show that the proposed system
works well, and all the methods achieved good results; however, the
best results obtained were for the Support Vector Machine (SVM)
with a linear kernel.

The research problem arose from the researchers’ sense of the importance of Digital Intelligence ... more The research problem arose from the researchers’ sense of the importance of Digital Intelligence (DI), as it is a basic requirement to help students engage in the digital world and be disciplined in using technology and digital techniques, as students’ ideas are sufficiently susceptible to influence at this stage in light of modern technology. The research aims to know the level of DI among university students using Artificial Intelligence (AI) techniques. To verify this, the researchers built a measure of DI. The measure in its final form consisted of (24) items distributed among (8) main skills, and the validity and reliability of the tool was confirmed. It was applied to a sample of 139 male and female students who were chosen in a random stratified manner from students at the University of Baghdad; College of Education for Pure Sciences/Ibn Al-Haitham - Department of Computer Science. The proposed AI model used two artificial intelligence techniques: Decision tree (DT) and Random Forest (RF). The classification accuracy using DT was 92.85. The RF technique was applied to find the essential features and the Pearson correlation to find the correlation between the features. The results showed that they possess DI, and this can contribute to developing plans and programs to develop it and improve their skills.
Zenodo (CERN European Organization for Nuclear Research), Feb 28, 2015
Electronic Commerce (EC) is an important field due to the many advantages it involves. This study... more Electronic Commerce (EC) is an important field due to the many advantages it involves. This study aims to discuss the constraints surrounding educated Iraqi women which results overall lack of entering the EC and to give us additional insight into why they are avoiding entering this world. Results reveal that lack of awareness, lack of government policy and support, language, security and trust are the most important factors that contributes to EC adoption in addition to many other factors.

Zenodo (CERN European Organization for Nuclear Research), Dec 30, 2014
Virtual organization is similar to traditional organization in principles, but is different in th... more Virtual organization is similar to traditional organization in principles, but is different in the ways it operates. It requires small creation costs compared to the traditional and it uses electronic commerce as the market place and distribution channel for its products and services.The aim of this article is to applying electronic commerce for a proposed virtual organization. The tools used to build an effective web application for virtual organization to provide virtual environment to the customers to do the transaction activities online include PHP, MySQL and Apache. HTML is used for displaying forms and tables and JavaScript is used for verification in client side. Finally, connecting it to 2Checkout.com company as a third party to perform the financial transactions in a secure manner. The system has a protected database by encryption and password with simplified administration to manage all activities without the need for any experience in programming and designing. Using a trusted international electronic money transfer company can be an essential assuring step.
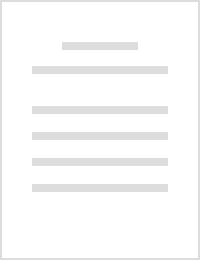
The Prediction of COVID 19 Disease Using Feature Selection Techniques
Journal of Physics: Conference Series
COVID 19 has spread rapidly around the world due to the lack of a suitable vaccine; therefore the... more COVID 19 has spread rapidly around the world due to the lack of a suitable vaccine; therefore the early prediction of those infected with this virus is extremely important attempting to control it by quarantining the infected people and giving them possible medical attention to limit its spread. This work suggests a model for predicting the COVID 19 virus using feature selection techniques. The proposed model consists of three stages which include the preprocessing stage, the features selection stage, and the classification stage. This work uses a data set consists of 8571 records, with forty features for patients from different countries. Two feature selection techniques are used in order to select the best features that affect the prediction of the proposed model. These are the Recursive Feature Elimination (RFE) as wrapper feature selection and the Extra Tree Classifier (ETC) as embedded feature selection. Two classification methods are applied for classifying the features vector...
International Journal of Machine Learning and Computing, 2019
Uploads
Papers by Wisal Hashim Abdulsalam
One of these fields is biometric authentication. Palm print recognition
is considered a fundamental aspect of biometric identification systems
due to the inherent stability, reliability, and uniqueness of palm print
features, coupled with their non-invasive nature. In this paper, we
develop an approach to identify individuals from palm print image
recognition using Orange software in which a hybrid of AI methods:
Deep Learning (DL) and traditional Machine Learning (ML) methods are used to enhance the overall performance metrics. The system
comprises of three stages: pre-processing, feature extraction, and feature classification or matching. The SqueezeNet deep learning model
was utilized to resize images and feature extraction. Finally, different
ML classifiers have been tested for recognition based on the extracted
features. The effectiveness of each classifier was assessed using various performance metrics. The results show that the proposed system
works well, and all the methods achieved good results; however, the
best results obtained were for the Support Vector Machine (SVM)
with a linear kernel.
One of these fields is biometric authentication. Palm print recognition
is considered a fundamental aspect of biometric identification systems
due to the inherent stability, reliability, and uniqueness of palm print
features, coupled with their non-invasive nature. In this paper, we
develop an approach to identify individuals from palm print image
recognition using Orange software in which a hybrid of AI methods:
Deep Learning (DL) and traditional Machine Learning (ML) methods are used to enhance the overall performance metrics. The system
comprises of three stages: pre-processing, feature extraction, and feature classification or matching. The SqueezeNet deep learning model
was utilized to resize images and feature extraction. Finally, different
ML classifiers have been tested for recognition based on the extracted
features. The effectiveness of each classifier was assessed using various performance metrics. The results show that the proposed system
works well, and all the methods achieved good results; however, the
best results obtained were for the Support Vector Machine (SVM)
with a linear kernel.