Papers by Azzeddine Bakdi
A class of PSO-tuned controllers in Lorenz chaotic system
Mathematics and Computers in Simulation
A Novel Semi-Supervised Learning Approach for State of Health Monitoring of Maritime Lithium-Ion Batteries
SSRN Electronic Journal
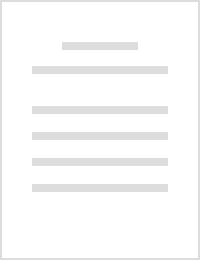
A Generalized Control Strategy for DC-DC Converters used in DC Microgrid with SWT, PV and FC
2020 2nd Global Power, Energy and Communication Conference (GPECOM)
A generalized control approach for DC-DC converter is proposed in this paper for optimal DC volta... more A generalized control approach for DC-DC converter is proposed in this paper for optimal DC voltage tracking control. The developed controller is used for both DC input voltage control of a buck converter and for output voltage control of an interleaved boost converter (IBC). These converters are widely used in DC microgrid systems that consist of fuel cell and other renewable energy sources. The DC microgrid in this paper contains a Small-scale Wind Turbine (SWT), a photovoltaic (PV) source and a fuel cell (FC) source which is used to stabilize the DC bus that feeds a load. The parameters of the optimal controller are optimized using the particle swarm optimization (PSO) algorithm and the stability of the controller is demonstrated using Lyapunov analysis. In addition, the designed controller regulates the PV system and SWT input voltages ensuring the two systems operate at their maximum power point (MPP). Furthermore, the control maintains the DC output voltage of the FC source at a desired constant value despite the current fluctuations due to the other sources.

Journal of Energy Storage
Longevity and safety of lithium-ion batteries are facilitated by efficient monitoring and adjustm... more Longevity and safety of lithium-ion batteries are facilitated by efficient monitoring and adjustment of the battery operating conditions. Hence, it is crucial to implement fast and accurate algorithms for State of Health (SoH) monitoring on the Battery Management System. The task is challenging due to the complexity and multitude of the factors contributing to the battery capacity degradation, especially because the different degradation processes occur at various timescales and their interactions play an important role. Data-driven methods bypass this issue by approximating the complex processes with statistical or machine learning models: they rely solely on the available cycling data, while remaining agnostic to the underlying real physical processes. This paper proposes a data-driven approach which is understudied in the context of battery degradation, despite being characterised by simplicity and ease of computation: the Multivariable Fractional Polynomial (MFP) regression. Models are trained from historical data of one exhausted cell and used to
Fullest COLREGs Evaluation Using Fuzzy Logic for Collaborative Decision-Making Analysis of Autonomous Ships in Complex Situations
IEEE Transactions on Intelligent Transportation Systems, 2022
Multiple Instance Learning With Random Forest for Event Logs Analysis and Predictive Maintenance in Ship Electric Propulsion System
IEEE Transactions on Industrial Informatics, 2022

Journal of Navigation, 2021
Economic and technological development has increased the amount, density and complexity of mariti... more Economic and technological development has increased the amount, density and complexity of maritime traffic, which has resulted in new challenges. One challenge is conforming to the distinct evasion manoeuvres required by vessels entering into near-collision situations (NCSs). Existing rules are vague and do not precisely dictate which, when and how collision avoidance manoeuvres (CAMs) should be executed. The automatic identification system (AIS) is widely used for vessel monitoring and traffic control. This paper presents an efficient, scalable method for processing large-scale raw AIS data using the closest point of approach (CPA) framework. NCSs are identified to create a database of historical traffic data. Important features describing CAMs are defined, estimated and analysed. Applications on a high-quality real-world data set show promising results for a subset of the identified situations. Future applications may play a significant role in the maritime regulatory framework, ...
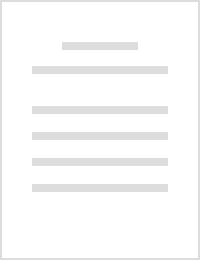
Improved process monitoring using PCA methods and adaptive threshold scheme
2017 5th International Conference on Electrical Engineering - Boumerdes (ICEE-B), 2017
Principal component analysis (PCA) is well-known as a multivariate statistical analysis method, i... more Principal component analysis (PCA) is well-known as a multivariate statistical analysis method, it has been widely and effectively applied for process monitoring. Furthermore, dynamic PCA (DPCA) methods consider serial correlation in addition to cross correlation between variables, and thus reflects the process dynamics. In this paper, we investigate static and dynamic monitoring, where different DPCA methods are used with uniform and different numbers of lags for each variable. Moreover, resulting statistics of these modelling methods are evaluated though our developed adaptive threshold, aimed at improving the monitoring scheme's robustness and sensitivity. Those constructed schemes are tested and evaluated though the eminent Tennessee Eastman Process (TEP) benchmark. The obtained results demonstrate superior performance and promising capabilities of some detection schemes.
Improvement of an adaptive thresholding scheme for fault detection in complex systems

Energy, 2019
In parallel to sustainable growth in solar fraction, continuous reductions in Photovoltaic (PV) m... more In parallel to sustainable growth in solar fraction, continuous reductions in Photovoltaic (PV) module and installation costs fuelled a profound adoption of residential Rooftop Mounted PV (RMPV) installations already reaching grid parity. RMPVs are promoted for economic, social, and environmental factors where they not only improve energy performance and reduce greenhouse effects but also contribute to bill savings. RMPV modules and energy conversion units are frequently subject to various types of anomalies which compromise power quality and promote fire risk and safety hazards for the personnel for which reliable protection is crucial. This article analyses historical data and presents a novel design that easily integrates with data storage units of RMPV systems to automatically process real-time data streams for reliable supervision. Dominant Transformed Components (TCs) are online extracted through multiblock Principal Component Analysis (PCA), most sensitive components are selected and their time-varying characteristics are recursively estimated in a moving window using smooth Kernel Density Estimation (KDE). Novel monitoring indices are developed as preventive alarms using Kullback-Leibler Divergence (KLD). This work exploits data records during 2015-2017 from thin-film, monocrystalline, and polycrystalline RMPV energy conversion systems. Fourteen test scenarios include array faults (line-to-line, line-to-ground, transient arc faults); DC-side mismatches (shadings, open circuits); grid-side anomalies (voltage sags, frequency variations); in addition to inverter anomalies and sensor faults.

Journal of Chemometrics, 2017
An improved process monitoring scheme is presented in this paper, it is based on the integration ... more An improved process monitoring scheme is presented in this paper, it is based on the integration of multivariate and univariate statistical analysis methods. Instead of conventional fixed control limits, adaptive thresholds are developed for common fault detection indices used with principal component analysis, including the Hotelling T 2 statistic and the sum of squared prediction error known as the Q statistic. The thresholds are updated based on a modified exponentially weighted moving average chart with a limited window length. The primary goal of this strategy is to enhance the performance of principal component analysis-based process monitoring method and overcome its shortcomings, by increasing fault detection rate to improve monitoring sensitivity and eliminating false alarms to ensure higher robustness and reliability. Fault detection in the revised model of Tennessee Eastman process benchmark is also investigated. The developed monitoring scheme is tested and compared with conventional fixed threshold technique, and its performance is evaluated across various types of process faults. The obtained results demonstrate the promising capabilities of the developed scheme. KEYWORDS adaptive threshold, fault detection, modified exponentially weighted moving average, principal component analysis, revised model of Tennessee Eastman process

Chemical Engineering Science, 2018
Principal Component Analysis (PCA) is the most common Multivariate Statistical Process Control (M... more Principal Component Analysis (PCA) is the most common Multivariate Statistical Process Control (MSPC) method that is widely used for Fault Detection and Diagnosis (FDD). Since early abnormality detection with high accuracy is required for safe and reliable process operation, False Alarms Rate (FAR), Missed Detection Rate (MDR) and the detection time delay are the major factors that must be taken into consideration when developing any process monitoring scheme. Unfortunately, the PCA performance, with fixed limits, is weak in terms of the stated factors. In contrast, conventional Moving Window PCA (MWPCA) is an adaptive technique which updates both the PCA model and the thresholds once a new normal observation is available. Yet, MWPCA methodology still does not reduce the MDR and the detection delay. In this paper, a Modified MWPCA (MMWPCA) with Fuzzy Logic Filter (FLF) is proposed to enhance the monitoring performance of PCA. It is an adaptive approach with a fixed model that combines both aforementioned techniques. The aim of using FLF is to ensure robustness to false alarms without affecting the Fault Detection (FD) 2 performance. The application of the proposed method has been carried out on the Tennessee Eastman Process (TEP). Hold-one and hold-five MMWPCA with FLF are applied and compared to recent FDD work in the literature. The obtained results demonstrate the superiority of the proposed technique in detecting different types of faults with high accuracy and with shorter time delay.

Renewable and Sustainable Energy Reviews, 2019
Advanced Fault Detection (FD), diagnosis, and isolation schemes are necessary to realize the requ... more Advanced Fault Detection (FD), diagnosis, and isolation schemes are necessary to realize the required levels of reliability and availability and to minimize financial losses against failures, explicitly in modern Wind Turbine Systems (WTSs) which are designed to generate electrical energy as efficiently and reliably as possible. This paper presents the design of a novel and inclusive FD framework on the basis of data-driven methods, it is intended for the online early detection of involuntary anomalies of various types and locations. Conventional methods are based on the exact model and/or signal patterns or hardware redundancy, and generally fail to address this issue. On the contrary, the presented algorithm is motivated by the availability of fast sensors and powerful computers yielding big data which can be explored to extract and exploit useful information. In a typical WTS, FD procedures face particular challenges attributed to high levels of measurement noise and sparse changes due to the fast dynamics as well as the switching control strategy along with transient phases. In this scope, a minimum and informative set of measured variables is proposed to accurately and completely describe the system behavior under its entire operation range; datasets are hence defined for appropriate dimensions and sampling time to satisfactorily model the measured data patterns and variability. Among data-based strategies, multivariate and univariate statistical analysis tools are recommended for this approach. A method based on Principal Component Analysis (PCA) is used in this study for its capabilities of dimensionality reduction, information de-correlation, and noise rejection. Multi-PCA-models are consequently used to train parallel statistical models through the proposed set of fault-relevant variables and used for online FD. Moreover, this method is reinforced by an adaptive threshold scheme based on an efficient modified EWMA control chart, the overall algorithm is robust to outliers and highly sensitive to small abnormalities and abrupt changes. Static and dynamic applications are investigated for modern wind turbine systems under different operating zones, and faults having different severity levels are studied ranging from sensors to actuators and up to system faults. The overall constructed algorithm shows significant potential applications compared to several methods recently reported in the literature in terms of applicability potential, robustness, and detection sensitivity.
Control Engineering Practice, 2017
This paper presents main results of fault detection and diagnosis in a cement manufacturing plant... more This paper presents main results of fault detection and diagnosis in a cement manufacturing plant using a new monitoring scheme. The scheme is based on multivariate statistical analysis and an adaptive threshold strategy. The process is statistically modeled using Principle Component Analysis (PCA). Instead of the conventional fixed control limits, adaptive thresholds are used to evaluate the common 2 and statistics as faults indicators. The adaptive thresholds are computed and updated using a modified Exponentially Weighted Moving Average (EWMA) chart. These techniques are merged together to construct a novel monitoring scheme whose effectiveness is demonstrated using involuntary real fault of a cement plant process and some simulated faulty cases.

Chemometrics and Intelligent Laboratory Systems, 2017
For large scale and complex processes, data-driven analysis methods are receiving increasing atte... more For large scale and complex processes, data-driven analysis methods are receiving increasing attention for fault detection and diagnosis to improve process operation by detecting when abnormal process operations exist and diagnosing the sources of the abnormalities. Common methods based on multivariate statistical analysis are widely used and particularly principal component analysis (PCA), fault detection indices used along with PCA including the Hotelling T² statistic and the sum of squared prediction error (SPE) known as the Q statistic can be used to identify faults. This paper develops a new adaptive thresholding scheme based on a modified exponentially weighted moving average (EWMA) control chart statistic, which is effective in detecting small changes and abrupt shifts in the process operation. The aim is to enhance the performance of PCA methods for process monitoring, while maintaining a low false alarm rate with good sensitivity of anomalies. The performance of the developed scheme is compared to a conventional fixed thresholding technique by evaluating the detection performance across various types of faults that occurred in the Tennessee Eastman Process, The results demonstrate the promising capabilities of our proposed scheme.

Journal of Marine Science and Engineering, 2019
The continuous growth in maritime traffic and recent developments towards autonomous navigation h... more The continuous growth in maritime traffic and recent developments towards autonomous navigation have directed increasing attention to navigational safety in which new tools are required to identify real-time risk and complex navigation situations. These tools are of paramount importance to avoid potentially disastrous consequences of accidents and promote safe navigation at sea. In this study, an adaptive ship-safety-domain is proposed with spatial risk functions to identify both collision and grounding risk based on motion and maneuverability conditions for all vessels. The algorithm is designed and validated through extensive amounts of Automatic Identification System (AIS) data for decision support over a large area, while the integration of the algorithm with other navigational systems will increase effectiveness and ensure reliability. Since a successful evacuation of a potential vessel-to-vessel collision, or a vessel grounding situation, is highly dependent on the nearby mane...
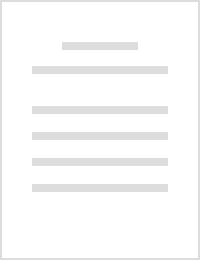
GPVS-Faults: Experimental Data for fault scenarios in grid-connected PV systems under MPPT and IPPT modes
Overview The Grid-connected PV System Faults (GPVS-Faults) data are collected from lab experiment... more Overview The Grid-connected PV System Faults (GPVS-Faults) data are collected from lab experiments of faults in a PV microgrid system. There are 16 data files in '.mat' and also '.csv', each for one experiment scenario, including photovoltaic array faults; inverter faults; grid anomalies; feedback sensor fault; and MPPT controller faults of various severity. GPVS-Faults data can be used to design/ validate/ compare various algorithms of fault detection/ diagnosis/ classification for PV system protection and reactive maintenance. Description The faults were introduced manually halfway during the experiments. The high-frequency measurements are noisy; with disturbances and variations of temperature and insolation during and between the experiments; MPPT/IPPT modes have adverse effects on the detection of low-magnitude faults. After critical faults, the operation is interrupted and the system may shut-down; the challenge is to detect the faults before a total failure. We refer interested researchers to read and cite the following references for detailed descriptions of: - GPVS-Faults scenarios, experiments, and data collection procedures are described in [1] with results for comparisons. - System description, energy management system, control/ communication of the PV system are detailed in [2]. -The description of the static multiblock version of the fault detection algorithm was presented in [3]. References [1]. A. Bakdi, W. Bounoua, A. Guichi, S. Mekhilef, (2020). Real-time fault detection in PV systems under MPPT using PMU and high-frequency multi-sensor data through online PCA-KDE-based multivariate KL Divergence. International Journal of Electrical Power & Energy Systems. [2]. A. Guichi, A. Talha, E. M. Berkouk, S. Mekhilef, S. Gassab, (2018). A new method for intermediate power point tracking for PV generator under partially shaded conditions in hybrid system. Sol. Energy. 170, 974-987. https://doi.org/10.1016/j.solener.2018.06.027. [3]. A. Bakdi, W. Bounoua, S. Mekhilef, L. M. Halabi, (2019). Nonparametri [...]
Robotics and Autonomous Systems, 2017
Please cite this article as: A. Bakdi, et al., Optimal path planning and execution for mobile rob... more Please cite this article as: A. Bakdi, et al., Optimal path planning and execution for mobile robots using genetic algorithm and adaptive fuzzy-logic control, Robotics and Autonomous Systems (2016),

ArXiv, 2021
Longevity and safety of Lithium-ion batteries are facilitated by efficient monitoring and adjustm... more Longevity and safety of Lithium-ion batteries are facilitated by efficient monitoring and adjustment of the battery operating conditions: hence, it is crucial to implement fast and accurate algorithms for State of Health (SoH) monitoring on the Battery Management System. The task is challenging due to the complexity and multitude of the factors contributing to the battery capacity degradation, especially because the different degradation processes occur at various timescales and their interactions play an important role. This paper proposes and compares two data-driven approaches: a Long Short-Term Memory neural network, from the field of deep learning, and a Multivariable Fractional Polynomial regression, from classical statistics. Models from both classes are trained from historical data of one exhausted cell and used to predict the SoH of other cells. This work uses data provided by the NASA Ames Prognostics Center of Excellence, characterised by varying loads which simulate dyna...
Testbed Scenario Design Exploiting Traffic Big Data for Autonomous Ship Trials Under Multiple Conflicts With Collision/Grounding Risks and Spatio-Temporal Dependencies
IEEE Transactions on Intelligent Transportation Systems
Uploads
Papers by Azzeddine Bakdi