Int. Journal & Conference by Md Mehedi Hasan, PhD
FaceEngine: A Tracking-Based Framework for Real-Time Face Recognition in Video Surveillance System.

MDPI Applied Sciences, 2022
Provisioning the stereoscopic 3D (S3D) video transmission services of admissible quality in a wir... more Provisioning the stereoscopic 3D (S3D) video transmission services of admissible quality in a wireless environment is an immense challenge for video service providers. Unlike for 2D videos, a widely accepted No-reference objective model for assessing transmitted 3D videos that explores the Human Visual System (HVS) appropriately has not been developed yet. Distortions perceived in 2D and 3D videos are significantly different due to the sophisticated manner in which the HVS handles the dissimilarities between the two different views. In real-time video transmission, viewers only have the distorted or receiver end content of the original video acquired through the communication medium. In this paper, we propose a No-reference quality assessment method that can estimate the quality of a stereoscopic 3D video based on HVS. By evaluating perceptual aspects and correlations of visual binocular impacts in a stereoscopic movie, the approach creates a way for the objective quality measure to assess impairments similarly to a human observer who would experience the similar material. Firstly, the disparity is measured and quantified by the region-based similarity matching algorithm, and then, the magnitude of the edge difference is calculated to delimit the visually perceptible areas of an image. Finally, an objective metric is approximated by extracting these significant perceptual image features. Experimental analysis with standard S3D video datasets demonstrates the lower computational complexity for the video decoder and comparison with the state-of-the-art algorithms shows the efficiency of the proposed approach for 3D video transmission at different quantization (QP 26 and QP 32) and loss rate (1% and 3% packet loss) parameters along with the perceptual distortion features.

Plant Methods, 2018
Background
Field phenotyping by remote sensing has received increased interest in recent years w... more Background
Field phenotyping by remote sensing has received increased interest in recent years with the possibility of achieving high-throughput analysis of crop fields. Along with the various technological developments, the application of machine learning methods for image analysis has enhanced the potential for quantitative assessment of a multitude of crop traits. For wheat breeding purposes, assessing the production of wheat spikes, as the grain-bearing organ, is a useful proxy measure of grain production. Thus, being able to detect and characterize spikes from images of wheat fields is an essential component in a wheat breeding pipeline for the selection of high yielding varieties.
Results
We have applied a deep learning approach to accurately detect, count and analyze wheat spikes for yield estimation. We have tested the approach on a set of images of wheat field trial comprising 10 varieties subjected to three fertilizer treatments. The images have been captured over one season, using high definition RGB cameras mounted on a land-based imaging platform, and viewing the wheat plots from an oblique angle. A subset of in-field images has been accurately labeled by manually annotating all the spike regions. This annotated dataset, called SPIKE, is then used to train four region-based Convolutional Neural Networks (R-CNN) which take, as input, images of wheat plots, and accurately detect and count spike regions in each plot. The CNNs also output the spike density and a classification probability for each plot. Using the same R-CNN architecture, four different models were generated based on four different datasets of training and testing images captured at various growth stages. Despite the challenging field imaging conditions, e.g., variable illumination conditions, high spike occlusion, and complex background, the four R-CNN models achieve an average detection accuracy ranging from 88 to 94% across different sets of test images. The most robust R-CNN model, which achieved the highest accuracy, is then selected to study the variation in spike production over 10 wheat varieties and three treatments. The SPIKE dataset and the trained CNN are the main contributions of this paper.
Conclusion
With the availability of good training datasets such us the SPIKE dataset proposed in this article, deep learning techniques can achieve high accuracy in detecting and counting spikes from complex wheat field images. The proposed robust R-CNN model, which has been trained on spike images captured during different growth stages, is optimized for application to a wider variety of field scenarios. It accurately quantifies the differences in yield produced by the 10 varieties we have studied, and their respective responses to fertilizer treatment. We have also observed that the other R-CNN models exhibit more specialized performances. The data set and the R-CNN model, which we make publicly available, have the potential to greatly benefit plant breeders by facilitating the high throughput selection of high yielding varieties.
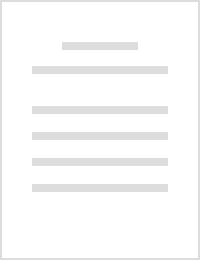
Detection and analysis of wheat spikes using Convolutional Neural Networks
Plant Methods, 2018
Background
Field phenotyping by remote sensing has received increased interest in recent years w... more Background
Field phenotyping by remote sensing has received increased interest in recent years with the possibility of achieving high-throughput analysis of crop fields. Along with the various technological developments, the application of machine learning methods for image analysis has enhanced the potential for quantitative assessment of a multitude of crop traits. For wheat breeding purposes, assessing the production of wheat spikes, as the grain-bearing organ, is a useful proxy measure of grain production. Thus, being able to detect and characterize spikes from images of wheat fields is an essential component in a wheat breeding pipeline for the selection of high yielding varieties.
Results
We have applied a deep learning approach to accurately detect, count and analyze wheat spikes for yield estimation. We have tested the approach on a set of images of wheat field trial comprising 10 varieties subjected to three fertilizer treatments. The images have been captured over one season, using high definition RGB cameras mounted on a land-based imaging platform, and viewing the wheat plots from an oblique angle. A subset of in-field images has been accurately labeled by manually annotating all the spike regions. This annotated dataset, called SPIKE, is then used to train four region-based Convolutional Neural Networks (R-CNN) which take, as input, images of wheat plots, and accurately detect and count spike regions in each plot. The CNNs also output the spike density and a classification probability for each plot. Using the same R-CNN architecture, four different models were generated based on four different datasets of training and testing images captured at various growth stages. Despite the challenging field imaging conditions, e.g., variable illumination conditions, high spike occlusion, and complex background, the four R-CNN models achieve an average detection accuracy ranging from 88 to 94% across different sets of test images. The most robust R-CNN model, which achieved the highest accuracy, is then selected to study the variation in spike production over 10 wheat varieties and three treatments. The SPIKE dataset and the trained CNN are the main contributions of this paper.
Conclusion
With the availability of good training datasets such us the SPIKE dataset proposed in this article, deep learning techniques can achieve high accuracy in detecting and counting spikes from complex wheat field images. The proposed robust R-CNN model, which has been trained on spike images captured during different growth stages, is optimized for application to a wider variety of field scenarios. It accurately quantifies the differences in yield produced by the 10 varieties we have studied, and their respective responses to fertilizer treatment. We have also observed that the other R-CNN models exhibit more specialized performances. The data set and the R-CNN model, which we make publicly available, have the potential to greatly benefit plant breeders by facilitating the high throughput selection of high yielding varieties.

The detection and tracking of a hand is an emerging research issue now-a-days to control the devi... more The detection and tracking of a hand is an emerging research issue now-a-days to control the devices by hand motion. Conventional hand detection methods use color and shape information from a RGB camera. With the recent advent of the depth camera, some researchers show that they can improve the performance of hand detection by combining the color (or intensity) information with the information from the depth camera. In this paper, we propose a novel method for hand detection using both color and depth information from Microsoft’s Kinect device. The proposed method extract the candidate hand regions from the depth image and select the best candidate based on the color and shape feature of each candidate regions. Then the contour of the selected candidate is determined in the higher resolution RGB image to improve the positional accuracy. For the tracking of the detected hand, we propose the boundary tracking method based on Generalized Hough Transform (GHT). The experimental results show that proposed method can improve the accuracy of hand motion detection over conventional methods.
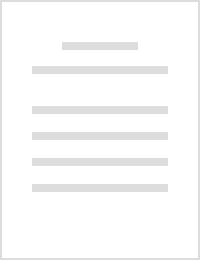
A Novel Quality Assessment of Transmitted 3D Videos Based on Binocular Rivalry Impact
Picture Coding Symposium, 2015
Binocular rivalry, a challenging visual phenomenon occurs frequently for dissimilar monocular sti... more Binocular rivalry, a challenging visual phenomenon occurs frequently for dissimilar monocular stimuli in 3D video communication particularly in error concealment. The quality assessment of distorted 3D video is a crucial element in designing and arranging advanced immersive media distribution platforms. In this paper we propose a quality assessment (QA) criterion that can be measured by considering the correlation of binocular rivalry impact. At first, subjective assessment is done for different frame freezing stereoscopic error concealment techniques and is compared with 2D to approximate the binocular rivalry characteristics. Secondly, a QA approach is proposed based on the perceptual characteristics of the Human Visual System (HVS). Finally, by incorporating the approximation with the perceptual measure, an assessment metric is generated for the transmitted 3D videos. Experimental analysis with common video datasets and comparison with different algorithms shows the efficiency of the proposed algorithm for video that is affected by asymmetric distortion and so is subject to binocular rivalry for stereoscopic views.
No-reference quality assessment of 3D videos based on human visual perception
ABSTRACT Broadcasting of high definition stereoscopic 3D videos is growing rapidly because of gre... more ABSTRACT Broadcasting of high definition stereoscopic 3D videos is growing rapidly because of greater demand in the mass consumer market. In spite of increasing consumer
interest, poor quality, crosstalk or side effects and visual quality degradation due to packet loss during transmission has hampered the advancement of 3D visualization. The quality
assessment of distorted 3D video is a crucial element in designing and arranging advanced immersive media distribution platforms. A widely accepted no-reference quality metric to ...
Subjective evaluation and statistical analysis for improved frame-loss error concealment of 3D videos
Abstract—Broadcasting of high definition stereoscopic 3D videos is growing rapidly because of inc... more Abstract—Broadcasting of high definition stereoscopic 3D videos is growing rapidly because of increased demand in the mass consumer market. In spite of increasing consumer interest, poor quality, crosstalk or side effects and lack of defined broadcast standards has hampered the advancement of 3D displays. Real time transmission of 3DTV sequences over packet based networks may result in visual quality degradation due to packet loss and delay. In conventional 2D videos, different extrapolation and directional interpolation strategies ...
Edge-based spatial concealment of digital dropout error in degraded archived media
A spatial concealment technique, based on the concept of the strength of an edge, is proposed fo... more A spatial concealment technique, based on the concept of the strength of an edge, is proposed for 'digital dropout'error, evident in old archived media. As long as the presence of pathological motion is observed in video sequences where current state-of-the-art methods fail to reproduce correct information, such spatial restoration offers a much better solution in terms of quality and complexity. Furthermore, relevant edge-based weighted interpolation is able to restore complicated edges and complex textures from the available ...
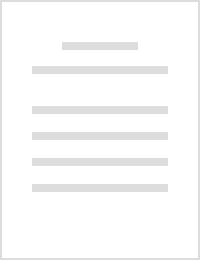
Hawkeye: Cloud Computing Based Automated Video Error Detection in Real-time
Journal of Internet Technology (SCIE, IF: .5), Jan 1, 2017
With the rapid development of video surveillance and broadcast systems monitoring the video quali... more With the rapid development of video surveillance and broadcast systems monitoring the video quality becomes an important aspect to assure better quality of service. Error detection is an important technique to measure the quality of videos transmitted over unreliable network. With the advent of HDTV previously subtle errors in videos are becoming more prominent. In this paper we want to propose a next generation cloud based video error detection system using video processing technology for making the detection process in real-time. At first, we introduce an automatic video error detection method and then propose a cloud computing platform. Finally we integrate error detection method with cloud computing platform named as Hawkeye to achieve a real time video error detection system which ensures contents integrity and minimizes testing time and efforts required to keep ahead of other conventional quality check system. Extensive experiments on prominent datasets and telecasted videos show that the proposed algorithm is very much efficient to detect errors for video broadcast and surveillance applications in terms of computation time and the detection of distorted frames

Fast and Reliable Structure-Oriented Distortion Measure for Video Processing, Jan 1, 2013
Structure-Oriented video distortions detection significantly impacts the effectiveness of video p... more Structure-Oriented video distortions detection significantly impacts the effectiveness of video processing algorithms. To transmit high quality videos and images with low bit rate, an effective compression algorithm removes the redundancy of statistical correlation and insignificant component of image signal. In video broadcasting and surveillance systems, not only the compression based but also content based distortion occurs during its transmission through wired or over wireless channel. To overcome these issues we first propose a measurement of these artifacts of videos by analyzing the distribution of local properties of image signals like dominant edge magnitude and direction to assure better Quality of Service. We also propose a metric to detect damaged frame by considering the contextual information, such as their consistency and edge continuity. According to the statistical information the distorted frames are then estimated based on the characteristics of their surrounding frames. Extensive experiments on prominent datasets and telecasted videos show that the proposed algorithm is very much efficient to detect errors for video broadcast and surveillance applications in terms of computation time and the detection of distorted frames.

Journal Of Electrical Bioimpedance (IF: 2.11 )
Electrical Impedance Tomography (EIT) has successive wide range in impedance imaging, but still i... more Electrical Impedance Tomography (EIT) has successive wide range in impedance imaging, but still it is difficult to extract cardiac-related conductivity changes and respiratory-related conductivity changes in spontaneous breathing subjects. Quite a few methods are attempted to extract these two signals such as electrocardiogram gated averaging, frequency domain filtering and principal component analysis. However, such methods are not able to take apart these components properly or put some effort in real time imaging and have their own limitations. The purpose of this paper is to introduce a new method in the EIT clinical application field, Independent Component Analysis (ICA) to extract cardiac and respiratory related signals in electrical impedance tomography. Independent component analysis has been introduced to use in electrical impedance tomography but this is the first attempt ever to implement this method to separate these two signals and image those independent conductivity distribution of respiration and cardiac changes independently. Data has been collected from a spontaneous breathing subject. Filtration technique has been used to remove random noise and multi level spatial ICA has been applied to obtain independent component signals which has been later used in reconstruction algorithm for imaging.
Measuring Blockiness of Videos using Edge Enhancement Filtering
To represent high quality videos or images with low bit rate, an effective compression algorithm ... more To represent high quality videos or images with low bit rate, an effective compression algorithm removes the redundancy because of statistical correlation and also the insignificant component of image signal. This paper represents a new algorithm to measure the blocking artifacts of videos by analyzing the distortions of local properties of image signals like dominant edge magnitude and direction. Extensive experiments on various videos show that the new algorithm is very much efficient and faster to measure the blocking artifacts in real time video error detection applications.
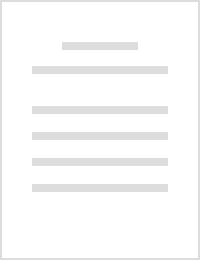
Blocking Artifact Detection by Analyzing the Distortions of Local Properties in Images
Now-a-days, recent trend is to represent high quality images or videos by using less bit represen... more Now-a-days, recent trend is to represent high quality images or videos by using less bit representation. To represent high quality videos or images with low bit rate, an effective compression algorithm removes the redundancy because of statistical correlation and also the insignificant component of image signal. This paper represents a new algorithm to measure the blocking artifacts of videos by analyzing the distortions of local properties of image signals like dominant edge magnitude and direction. For this purpose sobel convolution mask is used rather than kirsch mask to make the detection process faster and to model video noises that occur in broadcasting systems. Extensive experiments on various videos show that the new algorithm is very much efficient to measure the blocking artifacts in real time video error detection applications.

Cloud computing platform provides enormous opportunity to the users for fast computation, sharing... more Cloud computing platform provides enormous opportunity to the users for fast computation, sharing and transmission. Satellite image, geographical image, and aerial photograph have high resolutions with many different colors. Generally, image compression algorithms reduce data redundancy by encoding spatial dependencies. To bring out spatial dependencies
between these large volumes of data is very difficult and time consuming process. Moreover, the compression of these images has to be lossless to ensure faithful reproduction. Bit-plane coding technique allows us to take advantages from neighborhood similarity, often found in image plane which is more useful than accounting on gray level similarity. Moreover, bit-planes allow parallel processing of each plane in the clouds. In this paper, we present an approach to use the quadtree structures for efficient and progressive image compression using the cloud architecture which shows high compression ratios without any loss. Our proposed algorithm has simple structure and can compress/decompress any image very fast in the clouds while necessary.

An effecting compression algorithm removes the redundancy of image signal, when we want to repres... more An effecting compression algorithm removes the redundancy of image signal, when we want to represent high quality videos and images with lower bit rate. Removal of statistical correlation and insignificant components of image signal make the corresponding videos and images highly compressed. This paper represents a new algorithm to measure the blocking artifacts of videos by analyzing the distortions of local properties of image signals like dominant edge magnitude and direction. We also want to incorporate light weighted human vision measurement system like edge information to measure video artifacts in real time. Then simple bucket filling approach is applied, where the particular bucket contains the maximum value also indicating the block boundaries that are passed to the report module. After computing distortion measure a proposed detection approach is used to capture the distorted frames. Extensive experiments on various videos show that the new algorithm is very much efficient and faster to measure the independent lossy compressed block errors in real time video error detection applications.
Cloud computing is an emerging model of business computing that delivers computing as a service r... more Cloud computing is an emerging model of business computing that delivers computing as a service rather than a product, whereby shared resources, software and information are provided to computers and other devices as a utility over network. In recent years cloud computing has been put forward. It connects millions of computers to a super cloud. With the advent of HDTV previously subtle errors in videos are becoming more prominent, previously an extensive research field. In this paper we want to propose a next generation cloud based video error detection system using image/video processing technology. Hawkeye is an automatic error detection system which ensures contents integrity and minimizes testing time and efforts required to keep ahead of other conventional quality check system.
Proceedings of the 2012 International Conference on Information Technology
Now-a-days, recent trend is to represent high quality images or videos by using less bit represen... more Now-a-days, recent trend is to represent high quality images or videos by using less bit representation in broadcasting and surveillance systems.
In this paper, we have presented a new feature descriptor, the Local Transitional Pattern Varianc... more In this paper, we have presented a new feature descriptor, the Local Transitional Pattern Variance (LTPv), to represent facial images. A LTP feature is computed by encoding the transition of intensity changes around a local neighborhood in eight directions, and then, the LTPv descriptor of a facial image is generated from the integral projection of each LTP code weighted by its corresponding variance. Proposed LTPv descriptor used for face recognition using images from FERET database. Experimental results show that the LTPv descriptor yields improved recognition rate against other existing appearance-based feature descriptors.
Book Chapter by Md Mehedi Hasan, PhD
Measuring Blockiness of Videos using Edge Enhancement Filtering
To represent high quality videos or images with low bit rate, an effective compression algorithm ... more To represent high quality videos or images with low bit rate, an effective compression algorithm removes the redundancy because of statistical correlation and also the insignificant component of image signal. This paper represents a new algorithm to measure the blocking artifacts of videos by analyzing the distortions of local properties of image signals like dominant edge magnitude and direction. Extensive experiments on various videos show that the new algorithm is very much efficient and faster to measure the blocking artifacts in real time video error detection applications.
Uploads
Int. Journal & Conference by Md Mehedi Hasan, PhD
Field phenotyping by remote sensing has received increased interest in recent years with the possibility of achieving high-throughput analysis of crop fields. Along with the various technological developments, the application of machine learning methods for image analysis has enhanced the potential for quantitative assessment of a multitude of crop traits. For wheat breeding purposes, assessing the production of wheat spikes, as the grain-bearing organ, is a useful proxy measure of grain production. Thus, being able to detect and characterize spikes from images of wheat fields is an essential component in a wheat breeding pipeline for the selection of high yielding varieties.
Results
We have applied a deep learning approach to accurately detect, count and analyze wheat spikes for yield estimation. We have tested the approach on a set of images of wheat field trial comprising 10 varieties subjected to three fertilizer treatments. The images have been captured over one season, using high definition RGB cameras mounted on a land-based imaging platform, and viewing the wheat plots from an oblique angle. A subset of in-field images has been accurately labeled by manually annotating all the spike regions. This annotated dataset, called SPIKE, is then used to train four region-based Convolutional Neural Networks (R-CNN) which take, as input, images of wheat plots, and accurately detect and count spike regions in each plot. The CNNs also output the spike density and a classification probability for each plot. Using the same R-CNN architecture, four different models were generated based on four different datasets of training and testing images captured at various growth stages. Despite the challenging field imaging conditions, e.g., variable illumination conditions, high spike occlusion, and complex background, the four R-CNN models achieve an average detection accuracy ranging from 88 to 94% across different sets of test images. The most robust R-CNN model, which achieved the highest accuracy, is then selected to study the variation in spike production over 10 wheat varieties and three treatments. The SPIKE dataset and the trained CNN are the main contributions of this paper.
Conclusion
With the availability of good training datasets such us the SPIKE dataset proposed in this article, deep learning techniques can achieve high accuracy in detecting and counting spikes from complex wheat field images. The proposed robust R-CNN model, which has been trained on spike images captured during different growth stages, is optimized for application to a wider variety of field scenarios. It accurately quantifies the differences in yield produced by the 10 varieties we have studied, and their respective responses to fertilizer treatment. We have also observed that the other R-CNN models exhibit more specialized performances. The data set and the R-CNN model, which we make publicly available, have the potential to greatly benefit plant breeders by facilitating the high throughput selection of high yielding varieties.
Field phenotyping by remote sensing has received increased interest in recent years with the possibility of achieving high-throughput analysis of crop fields. Along with the various technological developments, the application of machine learning methods for image analysis has enhanced the potential for quantitative assessment of a multitude of crop traits. For wheat breeding purposes, assessing the production of wheat spikes, as the grain-bearing organ, is a useful proxy measure of grain production. Thus, being able to detect and characterize spikes from images of wheat fields is an essential component in a wheat breeding pipeline for the selection of high yielding varieties.
Results
We have applied a deep learning approach to accurately detect, count and analyze wheat spikes for yield estimation. We have tested the approach on a set of images of wheat field trial comprising 10 varieties subjected to three fertilizer treatments. The images have been captured over one season, using high definition RGB cameras mounted on a land-based imaging platform, and viewing the wheat plots from an oblique angle. A subset of in-field images has been accurately labeled by manually annotating all the spike regions. This annotated dataset, called SPIKE, is then used to train four region-based Convolutional Neural Networks (R-CNN) which take, as input, images of wheat plots, and accurately detect and count spike regions in each plot. The CNNs also output the spike density and a classification probability for each plot. Using the same R-CNN architecture, four different models were generated based on four different datasets of training and testing images captured at various growth stages. Despite the challenging field imaging conditions, e.g., variable illumination conditions, high spike occlusion, and complex background, the four R-CNN models achieve an average detection accuracy ranging from 88 to 94% across different sets of test images. The most robust R-CNN model, which achieved the highest accuracy, is then selected to study the variation in spike production over 10 wheat varieties and three treatments. The SPIKE dataset and the trained CNN are the main contributions of this paper.
Conclusion
With the availability of good training datasets such us the SPIKE dataset proposed in this article, deep learning techniques can achieve high accuracy in detecting and counting spikes from complex wheat field images. The proposed robust R-CNN model, which has been trained on spike images captured during different growth stages, is optimized for application to a wider variety of field scenarios. It accurately quantifies the differences in yield produced by the 10 varieties we have studied, and their respective responses to fertilizer treatment. We have also observed that the other R-CNN models exhibit more specialized performances. The data set and the R-CNN model, which we make publicly available, have the potential to greatly benefit plant breeders by facilitating the high throughput selection of high yielding varieties.
interest, poor quality, crosstalk or side effects and visual quality degradation due to packet loss during transmission has hampered the advancement of 3D visualization. The quality
assessment of distorted 3D video is a crucial element in designing and arranging advanced immersive media distribution platforms. A widely accepted no-reference quality metric to ...
between these large volumes of data is very difficult and time consuming process. Moreover, the compression of these images has to be lossless to ensure faithful reproduction. Bit-plane coding technique allows us to take advantages from neighborhood similarity, often found in image plane which is more useful than accounting on gray level similarity. Moreover, bit-planes allow parallel processing of each plane in the clouds. In this paper, we present an approach to use the quadtree structures for efficient and progressive image compression using the cloud architecture which shows high compression ratios without any loss. Our proposed algorithm has simple structure and can compress/decompress any image very fast in the clouds while necessary.
Book Chapter by Md Mehedi Hasan, PhD
Field phenotyping by remote sensing has received increased interest in recent years with the possibility of achieving high-throughput analysis of crop fields. Along with the various technological developments, the application of machine learning methods for image analysis has enhanced the potential for quantitative assessment of a multitude of crop traits. For wheat breeding purposes, assessing the production of wheat spikes, as the grain-bearing organ, is a useful proxy measure of grain production. Thus, being able to detect and characterize spikes from images of wheat fields is an essential component in a wheat breeding pipeline for the selection of high yielding varieties.
Results
We have applied a deep learning approach to accurately detect, count and analyze wheat spikes for yield estimation. We have tested the approach on a set of images of wheat field trial comprising 10 varieties subjected to three fertilizer treatments. The images have been captured over one season, using high definition RGB cameras mounted on a land-based imaging platform, and viewing the wheat plots from an oblique angle. A subset of in-field images has been accurately labeled by manually annotating all the spike regions. This annotated dataset, called SPIKE, is then used to train four region-based Convolutional Neural Networks (R-CNN) which take, as input, images of wheat plots, and accurately detect and count spike regions in each plot. The CNNs also output the spike density and a classification probability for each plot. Using the same R-CNN architecture, four different models were generated based on four different datasets of training and testing images captured at various growth stages. Despite the challenging field imaging conditions, e.g., variable illumination conditions, high spike occlusion, and complex background, the four R-CNN models achieve an average detection accuracy ranging from 88 to 94% across different sets of test images. The most robust R-CNN model, which achieved the highest accuracy, is then selected to study the variation in spike production over 10 wheat varieties and three treatments. The SPIKE dataset and the trained CNN are the main contributions of this paper.
Conclusion
With the availability of good training datasets such us the SPIKE dataset proposed in this article, deep learning techniques can achieve high accuracy in detecting and counting spikes from complex wheat field images. The proposed robust R-CNN model, which has been trained on spike images captured during different growth stages, is optimized for application to a wider variety of field scenarios. It accurately quantifies the differences in yield produced by the 10 varieties we have studied, and their respective responses to fertilizer treatment. We have also observed that the other R-CNN models exhibit more specialized performances. The data set and the R-CNN model, which we make publicly available, have the potential to greatly benefit plant breeders by facilitating the high throughput selection of high yielding varieties.
Field phenotyping by remote sensing has received increased interest in recent years with the possibility of achieving high-throughput analysis of crop fields. Along with the various technological developments, the application of machine learning methods for image analysis has enhanced the potential for quantitative assessment of a multitude of crop traits. For wheat breeding purposes, assessing the production of wheat spikes, as the grain-bearing organ, is a useful proxy measure of grain production. Thus, being able to detect and characterize spikes from images of wheat fields is an essential component in a wheat breeding pipeline for the selection of high yielding varieties.
Results
We have applied a deep learning approach to accurately detect, count and analyze wheat spikes for yield estimation. We have tested the approach on a set of images of wheat field trial comprising 10 varieties subjected to three fertilizer treatments. The images have been captured over one season, using high definition RGB cameras mounted on a land-based imaging platform, and viewing the wheat plots from an oblique angle. A subset of in-field images has been accurately labeled by manually annotating all the spike regions. This annotated dataset, called SPIKE, is then used to train four region-based Convolutional Neural Networks (R-CNN) which take, as input, images of wheat plots, and accurately detect and count spike regions in each plot. The CNNs also output the spike density and a classification probability for each plot. Using the same R-CNN architecture, four different models were generated based on four different datasets of training and testing images captured at various growth stages. Despite the challenging field imaging conditions, e.g., variable illumination conditions, high spike occlusion, and complex background, the four R-CNN models achieve an average detection accuracy ranging from 88 to 94% across different sets of test images. The most robust R-CNN model, which achieved the highest accuracy, is then selected to study the variation in spike production over 10 wheat varieties and three treatments. The SPIKE dataset and the trained CNN are the main contributions of this paper.
Conclusion
With the availability of good training datasets such us the SPIKE dataset proposed in this article, deep learning techniques can achieve high accuracy in detecting and counting spikes from complex wheat field images. The proposed robust R-CNN model, which has been trained on spike images captured during different growth stages, is optimized for application to a wider variety of field scenarios. It accurately quantifies the differences in yield produced by the 10 varieties we have studied, and their respective responses to fertilizer treatment. We have also observed that the other R-CNN models exhibit more specialized performances. The data set and the R-CNN model, which we make publicly available, have the potential to greatly benefit plant breeders by facilitating the high throughput selection of high yielding varieties.
interest, poor quality, crosstalk or side effects and visual quality degradation due to packet loss during transmission has hampered the advancement of 3D visualization. The quality
assessment of distorted 3D video is a crucial element in designing and arranging advanced immersive media distribution platforms. A widely accepted no-reference quality metric to ...
between these large volumes of data is very difficult and time consuming process. Moreover, the compression of these images has to be lossless to ensure faithful reproduction. Bit-plane coding technique allows us to take advantages from neighborhood similarity, often found in image plane which is more useful than accounting on gray level similarity. Moreover, bit-planes allow parallel processing of each plane in the clouds. In this paper, we present an approach to use the quadtree structures for efficient and progressive image compression using the cloud architecture which shows high compression ratios without any loss. Our proposed algorithm has simple structure and can compress/decompress any image very fast in the clouds while necessary.
many video processing applications, accurate knowledge of these errors or distortions present in the input video sequence is very important. In a real system, noises are mainly introduced by the camera and the quantization step of decoding process. But the distortions occur when the videos are transmitted through analog or digital medium. Some errors may be introduced when the analog video signal transmits
in wired channel, but in wireless communication it cannot be ignored as occurring frequently. So, structure-oriented video distortions detection significantly impacts the effectiveness of video processing algorithms.
Through the advancement of IPTV and HDTV technology, previously subtle errors in videos are now becoming more prominent because of the both structure oriented and compression based artifacts. To transmit high quality videos and images with low bit rate over the wireless channel, various compression algorithms are used. In B-DCT compression a slight change of luminance in border area can cause a step in the decoded image if the neighbor block fall into different quantization intervals. Therefore, the decompressed image and video exhibits various kind of artifacts. One of the most obtrusive artifacts is the ”Blocking Artifact”. Both compression and content based error occurs not only in video broadcasting but also surveillance systems during its transmission over wired and wireless channel.
In this dissertation, we focus towards the development of a real-time video quality check system.To overcome the above issues we first propose a measurement system for compression based and broadcasted artifacts and then analyze the distortion patterns occurred during broadcasting over wireless channels.
Firstly, The proposed system is achieved by a measurement of various artifacts of videos by analyzing the distribution of local properties of image signals like dominant edge magnitude and direction. We also propose a metric to detect damaged frame by considering the contextual information, such as their consistency and edge continuity.
To achieve human vision measurement system we incorporate light weighted edge gradient magnitude information for video artifacts. According to the statistical information the distorted frames are then estimated based on the characteristics of their surrounding frames. Then we generate a criteria function to detect the distorted frame from sequence of video frames.
Secondly, we propose a method to analyze the distortion pattern or block errors in video frames that occurred during its transmission and broadcasting over wireless channel. To achieve real-time performance we quantize edge gradient phase information of the image and histogram of the quantized values. By making the noise characteristics, we can filter out background pixels and compression based patterns.
Then use the prominent texture patterns to classify them in different block errors and analyze them for error concealment. So, through accumulating histogram-based edge gradient information we can achieve height, width, shape and rotation of the distortion patterns of the video frames and analyze the distorted content pattern not only in video error detection application but also in error concealment, restoration
and retrieval.
Finally, evaluating the performance of all the mechanisms through extensive experiments on prominent datasets and broadcasted videos show that the proposed algorithm is very much efficient to detect errors for video broadcast and surveillance applications in terms of computation time and analysis of distorted frames.
effort we deliver proper information to our nation.
Web application is an application that is accessed via Web browser over a network and also a computer software application that is coded in a browser-supported language (such as HTML, JavaScript, Java, etc.) and reliant on a common web browser to render the application executable. In this project an attempt has been made to build such a web site
which includes all kind of information related to liberation war as well as an informative resource of the Martyrs, the countrymen who sacrificed their lives to make our aspiration true. And through this work we want to introduce systematic, disciplined and quantifiable approaches to development, operation, and maintenance of Web-based applications
which termed as web Engineering which a pro-active approach and a growing collection of theoretical and empirical research in Web application development.