Papers by Surayya Ado Bala

This paper is an effort to recognize the emotion, from speech signals. The emotion recognition sy... more This paper is an effort to recognize the emotion, from speech signals. The emotion recognition system uses vocal structural features of speech as features for emotion recognition. For finding emotions vocal expression of eight emotions (Happy, Angry, Sad, Depressed, Bored, Anxious, Fear and Nervous) were considered in Hausa language. In order to recognize emotions, features from these emotions are computed from acoustic pattern of the signals. For collecting features from emotions rhythm (duration, pitch and energy), enunciation (formant) and diction (ZCR) were considered. These features were finally combined and classified by using KNN classifier. The algorithm is tested on newly developed dataset for Hausa language based on audio signals. The recognition system is tested with 8000 (eight thousand) emotions collected from 100 (one hundred) different speakers with different age groups and genders.

In recent years, various encoder-decoder-based UNet architecture has shown remarkable performance... more In recent years, various encoder-decoder-based UNet architecture has shown remarkable performance in medical image segmentation. However, these encoder-decoder U-Net has a drawback in learning multi-scale features in complex segmentation tasks and weak ability to generalize to other tasks. This paper proposed a generalize encoder-decoder model called dense dilated inception network (DDI-Net) for medical image segmentation by modifying U-Net architecture. We utilize three steps; firstly, we propose a dense path to replace the skip connection in the middle of the encoder and decoder to make the model deeper. Secondly, we replace the U-Net's basic convolution blocks with a modified inception module called multi-scale dilated inception module (MDI) to make the model wider without gradient vanish and with fewer parameters. Thirdly, data augmentation and normalization are applied to the training data to improve the model generalization. We evaluated the proposed model on three subtask...
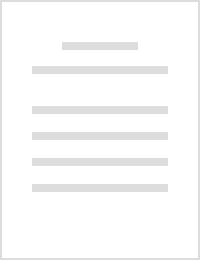
IPv4 and IPv6 Protocols: A Comparative Performance Study
2019 IEEE 10th Control and System Graduate Research Colloquium (ICSGRC)
The advancement in wireless technologies has allowed devices to access the internet from virtuall... more The advancement in wireless technologies has allowed devices to access the internet from virtually anywhere around the globe. However, the demand for reliable communication through voice and video streaming is significantly high. Internet protocol version 4 (IPv4) is a widely used internet protocol version in the world today, but due to the shortage of IPv4 addresses in the information technology (IT) world, a new internet protocol was introduced coined Internet protocol version 6 (IPv6) to address this issue. This paper aims to perform a comparative study on the performance of IPv4 and IPv6 on voice and video network traffic flow using performance metrics such as jitter, throughput, and packet loss. Accordingly, a testbed experimental environment is set-up with two hosts in client-server mode. Therefore, two scenarios (voice/video) were set-up to analyze the performance of the protocols. The result of this study shows that IPv6 has performed better than IPv4 in both experimental scenarios (voice/video).

2019 International Conference on Computing, Communication, and Intelligent Systems (ICCCIS)
Early diagnosis of diabetic retinopathy for treatment of the disease has been failing to reach di... more Early diagnosis of diabetic retinopathy for treatment of the disease has been failing to reach diabetic people living in rural areas. Shortage of trained ophthalmologists, limited availability of healthcare centers, and expensiveness of diagnostic equipment are among the reasons. Although many deep learning-based automatic diagnosis of diabetic retinopathy techniques have been implemented in the literature, these methods still fail to provide a point-of-care diagnosis. This raises the need for an independent diagnostic of diabetic retinopathy that can be used by a non-expert. Recently the usage of smartphones has been increasing across the world. Automated diagnoses of diabetic retinopathy can be deployed on smartphones in order to provide an instant diagnosis to diabetic people residing in remote areas. In this paper, inception based convolutional neural network and binary decision tree-based ensemble of classifiers have been proposed and implemented to detect and classify diabetic retinopathy. The proposed method was further imported into a smartphone application for mobile-based classification, which provides an offline and automatic system for diagnosis of diabetic retinopathy.
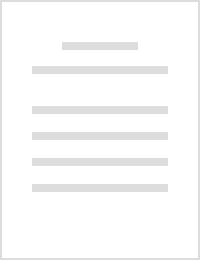
Deep Learning In Medical Imaging And Drug Design
Journal of Human Physiology
Over the last decade, deep learning (DL) methods have been extremely successful and widely used i... more Over the last decade, deep learning (DL) methods have been extremely successful and widely used in almost every domain. Researchers are now focusing on the convergence of medical imaging and drug design using deep learning to revolutionize medical diagnostic and improvement in the monitoring from response to therapy. DL a new machine learning paradigm that focuses on learning with deep hierarchical models of data. Medical imaging has transformed healthcare science, it was thought of as a diagnostic tool for disease, but now it is also used in drug design. Advances in medical imaging technology have enabled scientists to detect events at the cellular level. The role of medical imaging in drug design includes identification of likely responders, detection, diagnosis, evaluation, therapy monitoring, and follow-up. A qualitative medical image is transformed into a quantitative biomarker or surrogate endpoint useful in drug design decision-making. For this, a parameter needs to be identi...

In recent years, various encoder-decoder-based U-Net architecture has shown remarkable performanc... more In recent years, various encoder-decoder-based U-Net architecture has shown remarkable performance in medical image segmentation. However, these encoder-decoder U-Net has a drawback in learning multi-scale features in complex segmentation tasks and weak ability to generalize to other tasks. This paper proposed a generalize encoder-decoder model called dense dilated inception network (DDI-Net) for medical image segmentation by modifying U-Net architecture. We utilize three steps; firstly, we propose a dense path to replace the skip connection in the middle of the encoder and decoder to make the model deeper. Secondly, we replace the U-Net's basic convolution blocks with a modified inception module called multi-scale dilated inception module (MDI) to make the model wider without gradient vanish and with fewer parameters. Thirdly, data augmentation and normalization are applied to the training data to improve the model generalization. We evaluated the proposed model on three subtasks of the medical segmentation decathlon challenge. The experiment results prove that DDI-Net achieves superior performance than the compared methods with a Dice score of 0.82, 0.68, and 0.79 in brain tumor segmentation for edema, non-enhancing, and enhancing tumor. For the hippocampus segmentation, the result achieves 0.92 and 0.90 for anterior and posterior, respectively. For the heart segmentation, the method achieves 0.95 for the left atrial.
Uploads
Papers by Surayya Ado Bala