Papers by Antonius Alijoyo

Ilmu Komputer, Manajemen dan Sosial Swabumi : Suara Wawasan Sukabumi/Swabumi (Suara Wawasan Sukabumi), Mar 15, 2024
Di era perubahan cepat, peningkatan kualitas pelayanan pendidikan kesehatan, promosi program, dan... more Di era perubahan cepat, peningkatan kualitas pelayanan pendidikan kesehatan, promosi program, dan pemahaman biaya pendidikan berperan besar dalam memotivasi individu untuk melanjutkan pendidikan di bidang kesehatan. Faktor-faktor ini saling terkait dan memengaruhi keputusan siswa dan calon mahasiswa dalam melanjutkan pendidikan mereka. Memahami kompleksitas hubungan antara ketiga faktor ini dapat membantu lembaga pendidikan kesehatan dalam merancang strategi yang lebih efektif untuk meningkatkan minat dan partisipasi dalam pendidikan kesehatan. Dengan demikian, penelitian ini menjadi penting dalam merumuskan solusi yang relevan dan berkelanjutan dalam pengembangan pendidikan kesehatan. Penelitian ini akan dilakukan di STIKES Kota Sukabumi dengan mengolah informasi atau data dari hasil penyebaran kuisioner yang dilakukan pada 104 mahasiswa baru. Terdapat 22 indikator pertanyaan yang dijadikan referensi untuk pengolahan data menggunakan SPSS. Hasilnya dari variabel independen dan dependen yang diuji memenuhi standar reliabel dengan nilai 0,714 untuk variabel kualitas pelayanan, 0,698 untuk variabel promosi, 0,732 untuk variabel biaya dan 0,778 untuk variabel minat, sementara hasil dari uji validitas untuk 22 indikator pertanyaan yang diajukan semua indikator dinyatakan valid karena Rhitungnya lebih besar dari Rtabel yaitu: 0,192. Berdasarkan hasil perhitungan masing-masing variabel tersebut, diketahui bahwa variabel kualitas pelayanan, promosi dan biaya sama-sama berpengaruh secara simultan terhadap variabel minat.

Ilmu Komputer, Manajemen dan Sosial Swabumi : Suara Wawasan Sukabumi/Swabumi (Suara Wawasan Sukabumi), Mar 15, 2024
Usaha Mikro, kecil dan menengah (UMKM) Kota Sukabumi merupakan pelaku usaha disekitar daerah kota... more Usaha Mikro, kecil dan menengah (UMKM) Kota Sukabumi merupakan pelaku usaha disekitar daerah kota Sukabumi. UMKM Kota Sukabumi masih belum optimal dalam penggunaan digital marketing, inovasi produk dan modal usaha. Hal itu menyebabkan berkurangnya pemasaran dari UMKM itu sendiri sehingga menyebabkan pendapatan menjadi menurun. Penggunaan strategi marketing, inovasi produk dan modal usaha menjadi peranan penting untuk pelaku UMKM. Dalam penelitian ini, menganalisis seberapa pengaruh dari strategi marketing, inovasi produk dan modal usaha terhadap pendapatan pada usaha UMKM Kota Sukabumi. Dalam penelitian ini, menggunakan metode regresi linier sederhana dan menggunakan aplikasi SPSS versi 23.0 untuk pengolahan data. Hasil menunjukan variable digital marketing, inovasi dan modal usaha berpengaruh secara signifikan terhadap minat sebanyak 82% berdasarkan nilai koefisien determinasi, sisanya 18% dipengaruhi oleh variabel lainnya. Selanjutnya variabel X1, X2 dan X3 berpengaruh positif terhadap variabel Y.
Membangun Fondasi Complementary Agenda Untuk Transformasi Digital Bisnis
Coopetition, Apr 16, 2024
Rancang Bangun Sistem Informasi Manajemen Layanan Magang DI Diskominfo Kabupaten Purwakarta Berbasis Web Dengan Menggunakan Metode Extreme Programming
Jurnal Ilmiah Penelitian dan Pembelajaran Informatika, Feb 23, 2024
Enhancing AI interpretation and decision-making: Integrating cognitive computational models with deep learning for advanced uncertain reasoning systems
Alexandria Engineering Journal /Alexandria Engineering Journal, Jul 1, 2024
Ethical Considerations in Explainable AI: Balancing Transparency and User Privacy in English Language-based Virtual Assistants
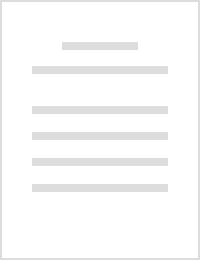
Unleashing the power of Bat optimized CNN-BiLSTM model for advanced network anomaly detection: Enhancing security and performance in IoT environments
Alexandria Engineering Journal, Nov 30, 2023
The growth of IoT (Internet of Things) devices has revolutionized several industries and brought ... more The growth of IoT (Internet of Things) devices has revolutionized several industries and brought about novel security threats. Recognizing network anomalies that may point to malicious activity or system flaws is a major issue. Traditional anomalous identification methods frequently need to catch up when dealing with the special traits of IoT environments, including resource limitations and changing network behavior. This paper introduces an innovative approach, the Bat-optimized CNN-BiLSTM model, to enhance the security and efficiency of IoT environments. This model combines the strengths of Convolutional Neural Networks (CNNs) for spatial analysis and Bidirectional Long Short-Term Memory (BiLSTM) networks for capturing temporal patterns, thus effectively representing time and space trends in IoT data. To optimize its performance further, researchers have leveraged the Bat algorithm, inspired by natural behaviors, to fine-tune the model. This program effectively searches for the best network anomaly detection parameters by imitating the echo activity of bats. Researchers want to increase detection accuracy by lowering false positives and false negatives using the Bat algorithm to enhance the CNN-BiLSTM model. The experimental findings show that the Bat-optimised CNN-BiLSTM model beats the state-ofthe-art anomaly detection methods with 99.43% accuracy and efficiency.

International Journal of Advanced Computer Science and Applications, Dec 31, 2022
The study introduces a comprehensive technique for enhancing the Natural Language Processing (NLP... more The study introduces a comprehensive technique for enhancing the Natural Language Processing (NLP) capabilities of virtual assistant systems. The method addresses the challenges of efficient information transfer and optimizing model size while ensuring improved performance, with a primary focus on model pertaining and distillation. To tackle the issue of vocabulary size affecting model performance, the study employs the SentencePiece tokenizer with unigram settings. This approach allows for the creation of a well-balanced vocabulary, which is essential for striking the right balance between task performance and resource efficiency. a novel pre-layernorm design is introduced, drawing inspiration from models like BERT and RoBERTa. This optimization optimizes the placement of layer normalization within transformer layers during the pretraining phase. Teacher models are effectively trained using masked language modeling objectives and the Deepspeed scaling framework. Modifications to model operations are made, and mixed precision training strategies are explored to ensure stability. The two-stage distillation method efficiently transfers knowledge from teacher models to student models. It begins with an intermediate model, and the data is distilled carefully using logit and hidden layer matching techniques. This information transfer significantly enhances the final student model while maintaining an ideal model size for low-latency applications. In this approach, innovative measurements, such as the precision of filling a mask, are employed to assess the effectiveness and quality of the methods. The findings demonstrate substantial improvements over publicly available models, showcasing the effectiveness of the strategy within complete virtual assistant systems. The proposed approach confirms the potential of the technique to enhance language comprehension and efficiency within virtual assistants, specifically addressing the challenges posed by real-world user inputs. Through extensive testing and rigorous analysis, the capability of the method to meet these objectives is validated.

International Journal of Advanced Computer Science and Applications, Dec 31, 2022
Effective detection has been extremely difficult due to plagiarism's pervasiveness throughout a v... more Effective detection has been extremely difficult due to plagiarism's pervasiveness throughout a variety of fields, including academia and research. Increasingly complex plagiarism detection strategies are being used by people, making traditional approaches ineffective. The assessment of plagiarism involves a comprehensive examination encompassing syntactic, lexical, semantic, and structural facets. In contrast to traditional string-matching techniques, this investigation adopts a sophisticated Natural Language Processing (NLP) framework. The preprocessing phase entails a series of intricate steps ultimately refining the raw text data. The crux of this methodology lies in the integration of two distinct metrics within the Encoder Representation from Transformers (E-BERT) approach, effectively facilitating a granular exploration of textual similarity. Within the realm of NLP, the amalgamation of Deep and Shallow approaches serves as a lens to delve into the intricate nuances of the text, uncovering underlying layers of meaning. The discerning outcomes of this research unveil the remarkable proficiency of Deep NLP in promptly identifying substantial revisions. Integral to this innovation is the novel utilization of the Waterman algorithm and an English-Spanish dictionary, which contribute to the selection of optimal attributes. Comparative evaluations against alternative models employing distinct encoding methodologies, along with logistic regression as a classifier underscore the potency of the proposed implementation. The culmination of extensive experimentation substantiates the system's prowess, boasting an impressive 99.5% accuracy rate in extracting instances of plagiarism. This research serves as a pivotal advancement in the domain of plagiarism detection, ushering in effective and sophisticated methods to combat the growing spectre of unoriginal content.

Publik, Feb 3, 2024
Penelitian ini memiliki tujuan untuk memberikan wawasan mengenai peran sistem dalam transformasi ... more Penelitian ini memiliki tujuan untuk memberikan wawasan mengenai peran sistem dalam transformasi proses bisnis operasional yayasan. Dalam era digital yang semakin maju, sistem database terintegrasi telah menjadi infrastruktur kunci dalam operasional berbagai sektor industri, termasuk sektor pendidikan. Yayasan Pendidikan X di Bandung memanfaatkan sistem database untuk melakukan perubahan proses bisnis serta mengoptimalkan efisiensi dalam manajemen Sumber Daya Manusia (SDM). Penelitian ini menggunakan pendekatan kuantitatif deskriptif dengan metode pengumpulan data berupa kuesioner yang dirancang secara sistematis dengan teknik pengambilan sampel secara proportional purposive sampling. Hasil penelitian memperlihatkan bahwa telah terjadi peningkatan yang signifikan dalam hal efisiensi waktu kerja, kemudahan dalam penginputan dan pengolahan data, serta penyajian informasi yang relevan untuk pengambilan keputusan berdasarkan hasil analisis data yang objektif. Penelitian ini juga kelak memberikan panduan serta manfaat bagi Yayasan Pendidikan lainnya terkait pemanfaatan sistem database terintegrasi di dalam pengelolaan manajemen SDM yang lebih baik.
Enterprise Risk Management : Pendekatan Praktis -2/E

Journal of Social Science, Nov 21, 2022
The objective of the study was analyzing the Business Transformation Strategy of PT. Krakatau Ste... more The objective of the study was analyzing the Business Transformation Strategy of PT. Krakatau Steel (Persero) Tbk Towards a Sustainable Company. The research followed a qualitative descriptive method. The results of the analysis show that the business transformation strategy carried out by PT. Krakatau Steel (Persero) Tbk towards a sustainable firm is done through debt restructuring and cost efficiency to increase labor productivity to lead the company to profitability. As a business entity that grows and develops in the community, this company also always strives to maintain harmonious relations with the surrounding community through corporate social responsibility (CSR) or partnerships and the Community Development Program (PKBL), which is held regularly every year. In addition, PT. Krakatau Steel (Persero) Tbk also consistently takes part in the Company Performance Assessment (PROPER) program conducted by the Republic of Indonesia's Ministry of Environment and Forestry.

JPPI (Jurnal Penelitian Pendidikan Indonesia), May 28, 2023
This study aims to understand how a pioneer Bus Rapid Transit (BRT) company in Indonesia strength... more This study aims to understand how a pioneer Bus Rapid Transit (BRT) company in Indonesia strengthens its business resilience when facing uncertainty due to the COVID-19 pandemic. A qualitative research approach with the case study method is used where the primary data source is respondents, who are determined based on the purposive sampling method. Data collection and analysis were conducted using triangulation techniques by distributing questionnaires, structured interviews, and focus group discussions, which have helped discover that the pioneer BRT company is not fully prepared for the COVID-19 pandemic. The company then decided to strengthen risk management capabilities by increasing risk management competencies that support decision-making in responding to the impact of the COVID-19 pandemic. Risk leaders believe that strengthening risk capabilities can boost the company's resilience in the face of the COVID-19 pandemic and help it succeed in the new-normal era later. However, it is recommended that companies implement a business continuity management system based on the ISO 22301:2019 Business Continuity Management System (BCMS) standard as a systematic and comprehensive approach. The implication of implementing this standard is strengthening the company's readiness to deal effectively with risks of resilience and business continuity in the future.
Dasar-dasar Enterprise Risk Management : Untuk Direktur dan Komisaris

This paper discusses a conceptual review on how Enterprise Risk Management (ERM) plays a role in ... more This paper discusses a conceptual review on how Enterprise Risk Management (ERM) plays a role in the competitiveness of companies that adopts the Value Chain (VC) model and Life Cycle Cost (LCC) approach. A literature review was conducted over some selected papers. The result shows that ERM is critically and fundamentally required to have an effective VC model and the practice of the LCC approach. Further, some discussions with practitioners in Indonesian listed companies gave a result that affirmed such conceptual review in a practical environment. The results lead to the recommendation that ERM should be a prerequisite before the value adoption chain model and LCC approach by a company. This paper, however, is without the field research, and therefore, an empirical study is strongly recommended to confirm the conclusion of this conceptual review and to understand the critical key success factors in building or establishing an effective interlink between ERM, VC, and LCC.

International Journal of Environmental, Sustainability and Social Science, Mar 31, 2022
Toll road operators need to implement effective risk management. This study focuses on how a Stat... more Toll road operators need to implement effective risk management. This study focuses on how a State-Owned Enterprise (SOE) toll road operator assesses the maturity of their ISO 31000-based risk management practices by using an ISO 31000-based risk management maturity model, ERMA ISO31000 RM3. The study is predominantly based on a qualitative approach through document reviews, questionnaires, and interviews. The assessment result shows that the company's risk management maturity score reaches 3.62 (a scale of 0.00-5.00) or at the DEFINED level of the risk management maturity. The study also shows that the company's risk management process gets the highest score, 4.45, while the lowest score, 3.22, is for the company's performance management. By using the maturity assessment result, the company's management can develop a risk management improvement road map to assist their efforts in increasing the effectiveness of their existing risk management practices. Referring to the assessment result, the management can prioritize the improvement on low-score maturity attributes, such as their performance management, risk culture, resilience and sustainability, risk management framework, and management process, while maintaining their current practices of the risk management process, which has already reached a considerably high maturity level.

Effective detection has been extremely difficult due to plagiarism's pervasiveness throughout a v... more Effective detection has been extremely difficult due to plagiarism's pervasiveness throughout a variety of fields, including academia and research. Increasingly complex plagiarism detection strategies are being used by people, making traditional approaches ineffective. The assessment of plagiarism involves a comprehensive examination encompassing syntactic, lexical, semantic, and structural facets. In contrast to traditional string-matching techniques, this investigation adopts a sophisticated Natural Language Processing (NLP) framework. The preprocessing phase entails a series of intricate steps ultimately refining the raw text data. The crux of this methodology lies in the integration of two distinct metrics within the Encoder Representation from Transformers (E-BERT) approach, effectively facilitating a granular exploration of textual similarity. Within the realm of NLP, the amalgamation of Deep and Shallow approaches serves as a lens to delve into the intricate nuances of the text, uncovering underlying layers of meaning. The discerning outcomes of this research unveil the remarkable proficiency of Deep NLP in promptly identifying substantial revisions. Integral to this innovation is the novel utilization of the Waterman algorithm and an English-Spanish dictionary, which contribute to the selection of optimal attributes. Comparative evaluations against alternative models employing distinct encoding methodologies, along with logistic regression as a classifier underscore the potency of the proposed implementation. The culmination of extensive experimentation substantiates the system's prowess, boasting an impressive 99.5% accuracy rate in extracting instances of plagiarism. This research serves as a pivotal advancement in the domain of plagiarism detection, ushering in effective and sophisticated methods to combat the growing spectre of unoriginal content.

The growth of IoT (Internet of Things) devices has revolutionized several industries and brought ... more The growth of IoT (Internet of Things) devices has revolutionized several industries and brought about novel security threats. Recognizing network anomalies that may point to malicious activity or system flaws is a major issue. Traditional anomalous identification methods frequently need to catch up when dealing with the special traits of IoT environments, including resource limitations and changing network behavior. This paper introduces an innovative approach, the Bat-optimized CNN-BiLSTM model, to enhance the security and efficiency of IoT environments. This model combines the strengths of Convolutional Neural Networks (CNNs) for spatial analysis and Bidirectional Long Short-Term Memory (BiLSTM) networks for capturing temporal patterns, thus effectively representing time and space trends in IoT data. To optimize its performance further, researchers have leveraged the Bat algorithm, inspired by natural behaviors, to fine-tune the model. This program effectively searches for the best network anomaly detection parameters by imitating the echo activity of bats. Researchers want to increase detection accuracy by lowering false positives and false negatives using the Bat algorithm to enhance the CNN-BiLSTM model. The experimental findings show that the Bat-optimised CNN-BiLSTM model beats the state-ofthe-art anomaly detection methods with 99.43% accuracy and efficiency.

The rapid proliferation of Internet of Things (IoT) technology has resulted in an exponential inc... more The rapid proliferation of Internet of Things (IoT) technology has resulted in an exponential increase in sensor data generated by diverse connected devices. Extracting valuable insights from this vast and complex data has become a critical challenge, necessitating advanced analytics techniques. In this project, to improve sensors analysis of data in applications for the Internet of Things, we suggest a unique technique that combines a method of attention with Long Short-Term Memory (LSTM). The attention mechanism selectively focuses on relevant sensors and their readings, dynamically weighting their importance based on the context, allowing intricate trends and connections between dates in the data to be captured by the algorithm. Concurrently, LSTM excels at modeling sequential information, enabling accurate predictions and efficient anomaly detection. Extensive experimentation and performance evaluations are conducted to assess the efficacy of our approach, contrasting it with current practices. The outcomes show that our suggested technique produces improved predictions. accuracy, efficiency, scalability, and robustness to missing data, outperforming other approaches. The synergistic integration of attention mechanism and LSTM empowers IoT applications with deeper insights and more informed decision-making capabilities. This research highlights the potential of advanced analytics techniques in optimizing IoT systems, fostering data-driven innovation, and promoting efficient resource utilization across various industries, including smart manufacturing.

The study introduces a comprehensive technique for enhancing the Natural Language Processing (NL... more The study introduces a comprehensive technique for enhancing the Natural Language Processing (NLP) capabilities of virtual assistant systems. The method addresses the challenges of efficient information transfer and optimizing model size while ensuring improved performance, with a primary focus on model pertaining and distillation. To tackle the issue of vocabulary size affecting model performance, the study employs the SentencePiece tokenizer with unigram settings. This approach allows for the creation of a well-balanced vocabulary, which is essential for striking the right balance between task performance and resourceefficiency. a novel pre-layernorm design is introduced, drawing inspiration from models like BERT and RoBERTa. This optimization optimizes the placement of layer normalization within transformer layers during the pretraining phase. Teacher models are effectively trained using
masked language modeling objectives and the Deepspeed scaling framework. Modifications to model operations are made, and mixed precision training strategies are explored to ensure stability. The two-stage distillation method efficiently transfers knowledge from teacher models to student models. It begins with an intermediate model, and the data is distilled carefully using logit and hidden layer matching techniques. This information transfer significantly enhances the final student model while
maintaining an ideal model size for low-latency applications. In this approach, innovative measurements, such as the precision of filling a mask, are employed to assess the effectiveness and quality of the methods. The findings demonstrate substantial improvements over publicly available models, showcasing the effectiveness of the strategy within complete virtual assistant systems. The proposed approach confirms the potential of the technique to enhance language comprehension and efficiency within virtual assistants, specifically addressing the challenges posed by real-world user inputs. Through extensive testing and rigorous analysis, the capability of the method to meet these objectives is validated.
Uploads
Papers by Antonius Alijoyo
masked language modeling objectives and the Deepspeed scaling framework. Modifications to model operations are made, and mixed precision training strategies are explored to ensure stability. The two-stage distillation method efficiently transfers knowledge from teacher models to student models. It begins with an intermediate model, and the data is distilled carefully using logit and hidden layer matching techniques. This information transfer significantly enhances the final student model while
maintaining an ideal model size for low-latency applications. In this approach, innovative measurements, such as the precision of filling a mask, are employed to assess the effectiveness and quality of the methods. The findings demonstrate substantial improvements over publicly available models, showcasing the effectiveness of the strategy within complete virtual assistant systems. The proposed approach confirms the potential of the technique to enhance language comprehension and efficiency within virtual assistants, specifically addressing the challenges posed by real-world user inputs. Through extensive testing and rigorous analysis, the capability of the method to meet these objectives is validated.
masked language modeling objectives and the Deepspeed scaling framework. Modifications to model operations are made, and mixed precision training strategies are explored to ensure stability. The two-stage distillation method efficiently transfers knowledge from teacher models to student models. It begins with an intermediate model, and the data is distilled carefully using logit and hidden layer matching techniques. This information transfer significantly enhances the final student model while
maintaining an ideal model size for low-latency applications. In this approach, innovative measurements, such as the precision of filling a mask, are employed to assess the effectiveness and quality of the methods. The findings demonstrate substantial improvements over publicly available models, showcasing the effectiveness of the strategy within complete virtual assistant systems. The proposed approach confirms the potential of the technique to enhance language comprehension and efficiency within virtual assistants, specifically addressing the challenges posed by real-world user inputs. Through extensive testing and rigorous analysis, the capability of the method to meet these objectives is validated.