Papers by Ioannis Psorakis
Abstract: Classifier combination methods need to make best use of the outputs of multiple, imperf... more Abstract: Classifier combination methods need to make best use of the outputs of multiple, imperfect classifiers to enable higher accuracy classifications. In many situations, such as when human decisions need to be combined, the base decisions can vary enormously in reliability. A Bayesian approach to such uncertain combination allows us to infer the differences in performance between individuals and to incorporate any available prior knowledge about their abilities when training data is sparse.
Results��� We describe how recent work in changepoint detection [1] can be brought to bear on the... more Results��� We describe how recent work in changepoint detection [1] can be brought to bear on the observation of dynamic interactions among heterogeneous agents.
Abstract: We propose a Bayesian methodology for one-mode projecting a bipartite network that is b... more Abstract: We propose a Bayesian methodology for one-mode projecting a bipartite network that is being observed across a series of discrete time steps. The resulting one mode network captures the uncertainty over the presence/absence of each link and provides a probability distribution over its possible weight values. Additionally, the incorporation of prior knowledge over previous states makes the resulting network less sensitive to noise and missing observations that usually take place during the data collection process.
Abstract: Identifying overlapping communities in networks is a challenging task. In this work we ... more Abstract: Identifying overlapping communities in networks is a challenging task. In this work we present a novel approach to community detection that utilises the Bayesian non-negative matrix factorisation (NMF) model to produce a probabilistic output for node memberships. The scheme has the advantage of computational efficiency, soft community membership and an intuitive foundation.
Inferring social network structure in ecological systems from spatio-temporal data streams
Abstract We propose a methodology for extracting social network structure from spatio-temporal da... more Abstract We propose a methodology for extracting social network structure from spatio-temporal datasets that describe timestamped occurrences of individuals. Our approach identifies temporal regions of dense agent activity and links are drawn between individuals based on their co-occurrences across these 'gathering events'. The statistical significance of these connections is then tested against an appropriate null model.
Identifying overlapping communities in networks is a challenging task. In this work we present a ... more Identifying overlapping communities in networks is a challenging task. In this work we present a novel
approach to community detection that utilizes a Bayesian nonnegative matrix factorization (NMF) model to
extract overlapping modules from a network. The scheme has the advantage of soft-partitioning solutions,
assignment of node participation scores to modules and an intuitive foundation. We present the performance of
the method against a variety of benchmark problems and compare and contrast it to several other algorithms for
community detection.
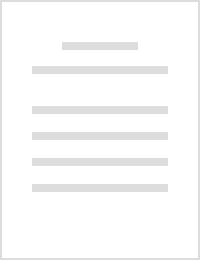
Multiclass Relevance Vector Machines: Sparsity and Accuracy
In this paper, we investigate the sparsity and recognition capabilities of two approximate Bayesi... more In this paper, we investigate the sparsity and recognition capabilities of two approximate Bayesian classification algorithms, the multiclass multi-kernel relevance vector machines (mRVMs) that have been recently proposed. We provide an insight into the behavior of the mRVM models by performing a wide experimentation on a large range of real-world datasets. Furthermore, we monitor various model fitting characteristics that identify the predictive nature of the proposed methods and compare against existing classification techniques. By introducing novel convergence measures, sample selection strategies and model improvements, it is demonstrated that mRVMs can produce state-of-the-art results on multiclass discrimination problems. In addition, this is achieved by utilizing only a very small fraction of the available observation data.
Uploads
Papers by Ioannis Psorakis
approach to community detection that utilizes a Bayesian nonnegative matrix factorization (NMF) model to
extract overlapping modules from a network. The scheme has the advantage of soft-partitioning solutions,
assignment of node participation scores to modules and an intuitive foundation. We present the performance of
the method against a variety of benchmark problems and compare and contrast it to several other algorithms for
community detection.
approach to community detection that utilizes a Bayesian nonnegative matrix factorization (NMF) model to
extract overlapping modules from a network. The scheme has the advantage of soft-partitioning solutions,
assignment of node participation scores to modules and an intuitive foundation. We present the performance of
the method against a variety of benchmark problems and compare and contrast it to several other algorithms for
community detection.