articles by Arkadiusz Wiśniowski

International Journal of Population Data Science, 2021
Introduction
Length of Stay (LoS) in Intensive Care Units (ICUs) is an important measure for plan... more Introduction
Length of Stay (LoS) in Intensive Care Units (ICUs) is an important measure for planning beds capacity during the Covid-19 pandemic. However, as the pandemic progresses and we learn more about the disease, treatment and subsequent LoS in ICU may change.
Objectives
To investigate the LoS in ICUs in England associated with Covid-19, correcting for censoring, and to evaluate the effect of known predictors of Covid-19 outcomes on ICU LoS.
Data sources
We used retrospective data on Covid-19 patients, admitted to ICU between 6 March and 24 May, from the “Covid-19 Hospitalisation in England Surveillance System” (CHESS) database, collected daily from England’s National Health Service, and collated by Public Health England.
Methods
We used Accelerated Failure Time survival models with Weibull and log-normal distributional assumptions to investigate the effect of predictors, which are known to be associated with poor Covid-19 outcomes, on the LoS in ICU.
Results
Patients admitted before 25 March had significantly longer LoS in ICU (mean = 18.4 days, median = 12), controlling for age, sex, whether the patient received Extracorporeal Membrane Oxygenation, and a co-morbid risk factors score, compared with the period after 7 April (mean = 15.4, median = 10). The periods of admission reflected the changes in the ICU admission policy in England. Patients aged 50-65 had the longest LoS, while higher co-morbid risk factors score led to shorter LoS. Sex and ethnicity were not associated with ICU LoS.
Conclusions
The skew of the predicted LoS suggests that a mean LoS, as compared with median, might be better suited as a measure used to assess and plan ICU beds capacity. This is important for the ongoing second and any future waves of Covid-19 cases and potential pressure on the ICU resources. Also, changes in the ICU admission policy are likely to be confounded with improvements in clinical knowledge of Covid-19.
Forecasting of immigration flows until 2025 for selected European countries using expert information

The European Journal of Development Research
We improve upon the modelling of India’s pandemic vulnerability. Our model is multidisciplinary a... more We improve upon the modelling of India’s pandemic vulnerability. Our model is multidisciplinary and recognises the nested levels of the epidemic. We create a model of the risk of severe COVID-19 and death, instead of a model of transmission. Our model allows for socio-demographic-group differentials in risk, obesity and underweight people, morbidity status and other conditioning regional and lifestyle factors. We build a hierarchical multilevel model of severe COVID-19 cases, using three different data sources: the National Family Health Survey for 2015/16, Census data for 2011 and data for COVID-19 deaths obtained cumulatively until June 2020. We provide results for 11 states of India, enabling best-yet targeting of policy actions. COVID-19 deaths in north and central India were higher in areas with older and overweight populations, and were more common among people with pre-existing health conditions, or who smoke, or who live in urban areas. Policy experts may both want to ‘follo...
Forecasting of immigration flows until 2025 for selected European countries using expert information

BMC Infectious Diseases
Background
Predicting hospital length of stay (LoS) for patients with COVID-19 infection is essen... more Background
Predicting hospital length of stay (LoS) for patients with COVID-19 infection is essential to ensure that adequate bed capacity can be provided without unnecessarily restricting care for patients with other conditions. Here, we demonstrate the utility of three complementary methods for predicting LoS using UK national- and hospital-level data.
Method
On a national scale, relevant patients were identified from the COVID-19 Hospitalisation in England Surveillance System (CHESS) reports. An Accelerated Failure Time (AFT) survival model and a truncation corrected method (TC), both with underlying Weibull distributions, were fitted to the data to estimate LoS from hospital admission date to an outcome (death or discharge) and from hospital admission date to Intensive Care Unit (ICU) admission date. In a second approach we fit a multi-state (MS) survival model to data directly from the Manchester University NHS Foundation Trust (MFT). We develop a planning tool that uses LoS estimates from these models to predict bed occupancy.
Results
All methods produced similar overall estimates of LoS for overall hospital stay, given a patient is not admitted to ICU (8.4, 9.1 and 8.0 days for AFT, TC and MS, respectively). Estimates differ more significantly between the local and national level when considering ICU. National estimates for ICU LoS from AFT and TC were 12.4 and 13.4 days, whereas in local data the MS method produced estimates of 18.9 days.
Conclusions
Given the complexity and partiality of different data sources and the rapidly evolving nature of the COVID-19 pandemic, it is most appropriate to use multiple analysis methods on multiple datasets. The AFT method accounts for censored cases, but does not allow for simultaneous consideration of different outcomes. The TC method does not include censored cases, instead correcting for truncation in the data, but does consider these different outcomes. The MS method can model complex pathways to different outcomes whilst accounting for censoring, but cannot handle non-random case missingness. Overall, we conclude that data-driven modelling approaches of LoS using these methods is useful in epidemic planning and management, and should be considered for widespread adoption throughout healthcare systems internationally where similar data resources exist.

Migration to and from Scotland might be affected by the outcome of the 2014 Scottish referendum o... more Migration to and from Scotland might be affected by the outcome of the 2014 Scottish referendum on the constitutional future of the United Kingdom. This potential change in migration has not been thoroughly analysed to date. The aim of this paper is thus to present a set of predictions of the possible effects of Scottish independence on internal and international migration. In particular, different sources of uncertainty of future migration flows are examined. The presented forecasts are based on the available historical data on migration flows, as well as on the opinions of a panel of experts on future migration trends. Bayesian statistical inference is used to combine different sources of uncertainty in a coherent manner. The results indicate that there is substantial uncertainty about future migration to and from Scotland, which increases with the forecast horizon. The most uncertain flow is international immigration to Scotland. Emigration from Scotland is more likely than not to increase in the near future, whereas migration between Scotland and the rest of the UK is expected to remain at similar levels to the present, irrespective of the outcome of the 2014 independence referendum.
Bayesian forecasting of immigration to selected European countries by using expert knowledge
Utilising expert opinion to improve the measurement of international migration in Europe
Integrated modeling of European migration
Forecasting environmental migration to the United Kingdom: an exploration using Bayesian models
Forecasting environmental migration to the United Kingdom: An exploration using Bayesian models
Erratum to Forecasting environmental migration to the United Kingdom: An exploration using Bayesian models (Popul Environ, 10.1007/s11111-013-0186-8)
Bayesian Population Forecasting: Extending the Lee-Carter Method
Migration from New-Accession Countries and Duration Expectancy in the EU-15: 2002?2008
Integrated modelling of age and sex patterns of European migration
Combining Labour Force Survey data to estimate migration flows: The case of migration from Poland to the UK
A multilevel functional data method for forecasting population, with an application to the United Kingdom
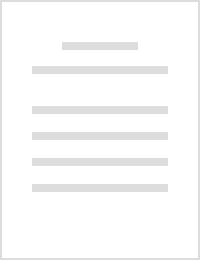
Applying and testing a forecasting model for age and sex patterns of immigration and emigration
International migration flows are considered the most difficult demographic component to forecast... more International migration flows are considered the most difficult demographic component to forecast and, for that reason, models for forecasting migration are few and relatively undeveloped. This is worrying because, in developed societies, international migration is often the most influential component of population growth and in debates about societal and economic change. In this paper, we address the need for better forecasting models of international migration by testing a hierarchical (bilinear) model within the Bayesian inferential framework recently developed to forecast age and sex patterns of immigration and emigration in the United Kingdom on other types of migration flow data. For the applications, we obtained age- and sex-specific time series data from Sweden, South Korea and Australia. The performance of the forecasts are compared and assessed with observed time series data. The results demonstrate the generality and flexibility of the model and Bayesian inference for forecasting migration, as well as highlighting areas for further research.
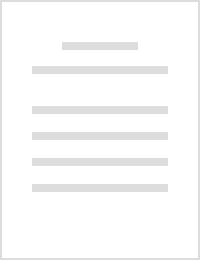
Assessing time series models for forecasting international migration: Lessons from the United Kingdom
Migration is one of the most unpredictable demographic processes. The aim of this article is to p... more Migration is one of the most unpredictable demographic processes. The aim of this article is to provide a blueprint for assessing various possible forecasting approaches in order to help safeguard producers and users of official migration statistics against misguided forecasts. To achieve that, we first evaluate the various existing approaches to modelling and forecasting of international migration flows. Subsequently, we present an empirical comparison of ex post performance of various forecasting methods, applied to international migration to and from the United Kingdom. The overarching goal is to assess the uncertainty of forecasts produced by using different forecasting methods, both in terms of their errors (biases) and calibration of uncertainty. The empirical assessment, comparing the results of various forecasting models against past migration estimates, confirms the intuition about weak predictability of migration, but also highlights varying levels of forecast errors for different migration streams. There is no single forecasting approach that would be well suited for different flows. We therefore recommend adopting a tailored approach to forecasts, and applying a risk management framework to their results, taking into account the levels of uncertainty of the individual flows, as well as the differences in their potential societal impact.
A Bayesian hierarchical model is proposed to forecast outcomes of binary referenda based on opini... more A Bayesian hierarchical model is proposed to forecast outcomes of binary referenda based on opinion poll data acquired over a period of time. It is demonstrated how the model provides a consistent probabilistic predictions of the final outcomes over the preceding months, effectively smoothing the volatility exhibited by individual polls. The method is illustrated using opinion poll data published before the Scottish independence referendum in 2014, in which Scotland voted to remain a part of the United Kingdom, and subsequently validate it on the data related to the 2016 referendum on the continuing membership of the United Kingdom in the European Union.
Uploads
articles by Arkadiusz Wiśniowski
Length of Stay (LoS) in Intensive Care Units (ICUs) is an important measure for planning beds capacity during the Covid-19 pandemic. However, as the pandemic progresses and we learn more about the disease, treatment and subsequent LoS in ICU may change.
Objectives
To investigate the LoS in ICUs in England associated with Covid-19, correcting for censoring, and to evaluate the effect of known predictors of Covid-19 outcomes on ICU LoS.
Data sources
We used retrospective data on Covid-19 patients, admitted to ICU between 6 March and 24 May, from the “Covid-19 Hospitalisation in England Surveillance System” (CHESS) database, collected daily from England’s National Health Service, and collated by Public Health England.
Methods
We used Accelerated Failure Time survival models with Weibull and log-normal distributional assumptions to investigate the effect of predictors, which are known to be associated with poor Covid-19 outcomes, on the LoS in ICU.
Results
Patients admitted before 25 March had significantly longer LoS in ICU (mean = 18.4 days, median = 12), controlling for age, sex, whether the patient received Extracorporeal Membrane Oxygenation, and a co-morbid risk factors score, compared with the period after 7 April (mean = 15.4, median = 10). The periods of admission reflected the changes in the ICU admission policy in England. Patients aged 50-65 had the longest LoS, while higher co-morbid risk factors score led to shorter LoS. Sex and ethnicity were not associated with ICU LoS.
Conclusions
The skew of the predicted LoS suggests that a mean LoS, as compared with median, might be better suited as a measure used to assess and plan ICU beds capacity. This is important for the ongoing second and any future waves of Covid-19 cases and potential pressure on the ICU resources. Also, changes in the ICU admission policy are likely to be confounded with improvements in clinical knowledge of Covid-19.
Predicting hospital length of stay (LoS) for patients with COVID-19 infection is essential to ensure that adequate bed capacity can be provided without unnecessarily restricting care for patients with other conditions. Here, we demonstrate the utility of three complementary methods for predicting LoS using UK national- and hospital-level data.
Method
On a national scale, relevant patients were identified from the COVID-19 Hospitalisation in England Surveillance System (CHESS) reports. An Accelerated Failure Time (AFT) survival model and a truncation corrected method (TC), both with underlying Weibull distributions, were fitted to the data to estimate LoS from hospital admission date to an outcome (death or discharge) and from hospital admission date to Intensive Care Unit (ICU) admission date. In a second approach we fit a multi-state (MS) survival model to data directly from the Manchester University NHS Foundation Trust (MFT). We develop a planning tool that uses LoS estimates from these models to predict bed occupancy.
Results
All methods produced similar overall estimates of LoS for overall hospital stay, given a patient is not admitted to ICU (8.4, 9.1 and 8.0 days for AFT, TC and MS, respectively). Estimates differ more significantly between the local and national level when considering ICU. National estimates for ICU LoS from AFT and TC were 12.4 and 13.4 days, whereas in local data the MS method produced estimates of 18.9 days.
Conclusions
Given the complexity and partiality of different data sources and the rapidly evolving nature of the COVID-19 pandemic, it is most appropriate to use multiple analysis methods on multiple datasets. The AFT method accounts for censored cases, but does not allow for simultaneous consideration of different outcomes. The TC method does not include censored cases, instead correcting for truncation in the data, but does consider these different outcomes. The MS method can model complex pathways to different outcomes whilst accounting for censoring, but cannot handle non-random case missingness. Overall, we conclude that data-driven modelling approaches of LoS using these methods is useful in epidemic planning and management, and should be considered for widespread adoption throughout healthcare systems internationally where similar data resources exist.
Length of Stay (LoS) in Intensive Care Units (ICUs) is an important measure for planning beds capacity during the Covid-19 pandemic. However, as the pandemic progresses and we learn more about the disease, treatment and subsequent LoS in ICU may change.
Objectives
To investigate the LoS in ICUs in England associated with Covid-19, correcting for censoring, and to evaluate the effect of known predictors of Covid-19 outcomes on ICU LoS.
Data sources
We used retrospective data on Covid-19 patients, admitted to ICU between 6 March and 24 May, from the “Covid-19 Hospitalisation in England Surveillance System” (CHESS) database, collected daily from England’s National Health Service, and collated by Public Health England.
Methods
We used Accelerated Failure Time survival models with Weibull and log-normal distributional assumptions to investigate the effect of predictors, which are known to be associated with poor Covid-19 outcomes, on the LoS in ICU.
Results
Patients admitted before 25 March had significantly longer LoS in ICU (mean = 18.4 days, median = 12), controlling for age, sex, whether the patient received Extracorporeal Membrane Oxygenation, and a co-morbid risk factors score, compared with the period after 7 April (mean = 15.4, median = 10). The periods of admission reflected the changes in the ICU admission policy in England. Patients aged 50-65 had the longest LoS, while higher co-morbid risk factors score led to shorter LoS. Sex and ethnicity were not associated with ICU LoS.
Conclusions
The skew of the predicted LoS suggests that a mean LoS, as compared with median, might be better suited as a measure used to assess and plan ICU beds capacity. This is important for the ongoing second and any future waves of Covid-19 cases and potential pressure on the ICU resources. Also, changes in the ICU admission policy are likely to be confounded with improvements in clinical knowledge of Covid-19.
Predicting hospital length of stay (LoS) for patients with COVID-19 infection is essential to ensure that adequate bed capacity can be provided without unnecessarily restricting care for patients with other conditions. Here, we demonstrate the utility of three complementary methods for predicting LoS using UK national- and hospital-level data.
Method
On a national scale, relevant patients were identified from the COVID-19 Hospitalisation in England Surveillance System (CHESS) reports. An Accelerated Failure Time (AFT) survival model and a truncation corrected method (TC), both with underlying Weibull distributions, were fitted to the data to estimate LoS from hospital admission date to an outcome (death or discharge) and from hospital admission date to Intensive Care Unit (ICU) admission date. In a second approach we fit a multi-state (MS) survival model to data directly from the Manchester University NHS Foundation Trust (MFT). We develop a planning tool that uses LoS estimates from these models to predict bed occupancy.
Results
All methods produced similar overall estimates of LoS for overall hospital stay, given a patient is not admitted to ICU (8.4, 9.1 and 8.0 days for AFT, TC and MS, respectively). Estimates differ more significantly between the local and national level when considering ICU. National estimates for ICU LoS from AFT and TC were 12.4 and 13.4 days, whereas in local data the MS method produced estimates of 18.9 days.
Conclusions
Given the complexity and partiality of different data sources and the rapidly evolving nature of the COVID-19 pandemic, it is most appropriate to use multiple analysis methods on multiple datasets. The AFT method accounts for censored cases, but does not allow for simultaneous consideration of different outcomes. The TC method does not include censored cases, instead correcting for truncation in the data, but does consider these different outcomes. The MS method can model complex pathways to different outcomes whilst accounting for censoring, but cannot handle non-random case missingness. Overall, we conclude that data-driven modelling approaches of LoS using these methods is useful in epidemic planning and management, and should be considered for widespread adoption throughout healthcare systems internationally where similar data resources exist.