Papers by Ngo Le Huy Hien

Cybernetics and Physics, 2024
The robust and accurate identification of different forms of manure stands as a pivotal imperativ... more The robust and accurate identification of different forms of manure stands as a pivotal imperative within the domain of agriculture. Near-infrared (NIR) Spectroscopy has emerged as an expeditious, efficient, nondestructive, and reliable approach to addressing this challenging task. NIR spectroscopy has the potential to serve as a valuable tool for the classification and identification of manure varieties. In order to enhance fertilizer identification performance, this study proposes a novel model called NIRsViT which classifies fertilizers by employing a combination of deep learning Vision Transformer model on NIR spectral data. The introduced model’s performance outperforms existing deep learning models, with an F1-Score of 86.42% and an accuracy rate of 95.19%. Additionally, the model’s classification performance has been significantly improved by proposed imbalanced data processing approaches, Focal Loss, and Upsample, with an F1-Score up to 93.91%, the improved F1-score proved that imbalanced data was considerably solved. The proposed method is a promising approach to handling imbalanced NIR spectral data and acts as a pioneering benchmark for subsequent models in manure identification through NIR spectroscopy. Future research gears toward improving the NIRsViT model’s temporal efficiency and computational load, while also testing the introduced imbalanced data handling approach for efficiency comparison across various models and larger datasets.

International Research Journal of Engineering and Technology, 2018
Autism Spectrum Disorder (ASD) is becoming a big issue in numerous countries around the world whi... more Autism Spectrum Disorder (ASD) is becoming a big issue in numerous countries around the world which can even negatively affect human natural evolution. Even though autism can be diagnosed early-before 2 years old, most children were not diagnosed with ASD until the age of 4 because of its complex symptoms and ambiguous manifestation in infant’s disorders. Applying science and technology into early autism diagnosis is of vital importance, especially when data mining branches and decision-making support systems are developing and achieving many accomplishments in various fields, medicine included. Contributing to those developments, the combination between the Artificial Neural Network (ANN) and Fuzzy logic has triggered a huge revolution in data mining and is able to solve a variety of problems. This paper is the elaboration on the method of employing this combination to facilitate the early diagnosis of ASD. The result of the paper shows that the aforementioned approach has the potential to be the fundamental basis of the supporting decision-making system in ASD researching and diagnosing.

Journal of Universal Computer Science, Jan 27, 2024
The deployment of autonomous vehicles has the potential to significantly lessen the variety of cu... more The deployment of autonomous vehicles has the potential to significantly lessen the variety of current harmful externalities, (such as accidents, traffic congestion, security, and environmental degradation), making autonomous vehicles an emerging topic of research. In this paper, a literature review of autonomous vehicle development has been conducted with a notable finding that autonomous vehicles will inevitably become an indispensable future greener solution. Subsequently, 5 different deep learning models, YOLOv5s, EfficientNet-B7, Xception, MobilenetV3, and InceptionV4, have been built and analyzed for 2-D object recognition in the navigation system. While testing on the BDD100K dataset, YOLOv5s and EfficientNet-B7 appear to be the two best models. Finally, this study has proposed Hessian, Laplacian, and Hessian-based Ridge Detection filtering techniques to optimize the performance and sustainability of those 2 models. The results demonstrate that these filters could increase the mean average precision by up to 11.81%, reduce detection time by up to 43.98%, and significantly reduce energy consumption by up to 50.69% when applied to YOLOv5s and EfficientNet-B7 models. Overall, all the experiment results are promising and could be extended to other domains for semantic understanding of the environment. Additionally, various filtering algorithms for multiple object detection and classification could be applied to other areas. Different recommendations and future work have been clearly defined in this study.

Informatica, 2024
In recent years, Magnetic Resonance Imaging (MRI) has emerged as a prevalent medical imaging tech... more In recent years, Magnetic Resonance Imaging (MRI) has emerged as a prevalent medical imaging technique, offering comprehensive anatomical and functional information. However, the MRI data acquisition process presents several challenges, including time-consuming procedures, prone motion artifacts, and hardware constraints. To address these limitations, this study proposes a novel method that leverages the power of generative adversarial networks (GANs) to generate multi-domain MRI images from a single input MRI image. Within this framework, two primary generator architectures, namely ResUnet and StarGANs generators, were incorporated. Furthermore, the networks were trained on multiple datasets, thereby augmenting the available data, and enabling the generation of images with diverse contrasts obtained from different datasets, given an input image from another dataset. Experimental evaluations conducted on the IXI and BraTS2020 datasets substantiate the efficacy of the proposed method compared to an existing method, as assessed through metrics such as Structural Similarity Index (SSIM), Peak Signal-to-Noise Ratio (PSNR) and Normalized Mean Absolute Error (NMAE). The synthesized images resulting from this method hold substantial potential as invaluable resources for medical professionals engaged in research, education, and clinical applications. Future research gears towards expanding experiments to larger datasets and encompassing the proposed approach to 3D images, enhancing medical diagnostics within practical applications.

Informatica, Jan 31, 2024
Due to the robust growth in the amount of data and Internet users, there has been a significant r... more Due to the robust growth in the amount of data and Internet users, there has been a significant rise in information overload, hindering timely access to user demand. While information retrieval systems, such as Google, Bing, and Altavista have partially addressed this challenge, prioritization and personalization of information have yet to be fully implemented. Therefore, recommendation systems are developed to resolve the issue by filtering and segmenting important information from an enormous volume of data based on different criteria such as preferences, interests, and user behaviors. By collecting data on users' interests and purchased products, the system can predict whether a particular user would enjoy an item, thus delivering an appropriate suggestion strategy. However, the increased number of Internet users and items has resulted in sparseness in increasingly vast datasets, reducing the performance of recommendation algorithms. Therefore, this study developed a model integrating Convolutional Neural Network (CNN) and Matrix Factorization (MF) to add extra product and user information, extract contexts, and add bias to the observed ratings in the training process, attempting to enhance the recommendation accuracy and context understanding. This approach can take advantage of CNN to efficiently capture an image's or document's local features, with the combination of MF to create relationships between 2 main entities, users and items. The proposed model obtained the highest RMSE of 0.93 when predicting favorable movies for 4,000 users, with an ability to learn complex contextual features and suggest more relevant content. The results are promising and can act as a reference for developing context understanding in recommendation systems, and future work may focus on optimizing the performance and developing more text-processing techniques. Povzetek: Razvit je nov model globokega učenja, ki združuje konvolucijske nevronske mreže (CNN) in matrično faktorizacijo (MF) za izboljšanje natančnosti in razumevanja konteksta v priporočilnih sistemih.
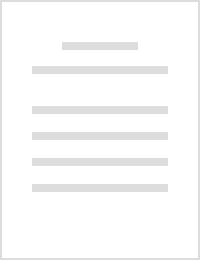
PlantKViT: A Combination Model of Vision Transformer and KNN for Forest Plants Classification
Journal of Universal Computer Science, Sep 27, 2023
The natural ecosystem incorporates thousands of plant species and distinguishing them is normally... more The natural ecosystem incorporates thousands of plant species and distinguishing them is normally manual, complicated, and time-consuming. Since the task requires a large amount of expertise, identifying forest plant species relies on the work of a team of botanical experts. The emergence of Machine Learning, especially Deep Learning, has opened up a new approach to plant classification. However, the application of plant classification based on deep learning models remains limited. This paper proposed a model, named PlantKViT, combining Vision Transformer architecture and the KNN algorithm to identify forest plants. The proposed model provides high efficiency and convenience for adding new plant species. The study was experimented with using Resnet-152, ConvNeXt networks, and the PlantKViT model to classify forest plants. The training and evaluation were implemented on the dataset of DanangForestPlant, containing 10,527 images and 489 species of forest plants. The accuracy of the proposed PlantKViT model reached 93%, significantly improved compared to the ConvNeXt model at 89% and the Resnet-152 model at only 76%. The authors also successfully developed a website and 2 applications called 'plant id' and 'Danangplant' on the iOS and Android platforms respectively. The PlantKViT model shows the potential in forest plant identification not only in the conducted dataset but also worldwide. Future work should gear toward extending the dataset and enhance the accuracy and performance of forest plant identification.

Cybernetics and physics, Sep 29, 2023
In the current digital era, text documents become valuable for businesses to reach potential cust... more In the current digital era, text documents become valuable for businesses to reach potential customers and curtail advertising costs. However, extracting and classifying beneficial information from texts can prove challenging and time-consuming, particularly in complex languages like Vietnamese. This study aims to classify the sentiment of Vietnamese comments on e-commerce websites into negative and positive classes. To enhance the performance of sentiment classification, the study fine-tuned traditional models of Convolutional Neural Networks and Recurrent Neural Networks (RNN). Then, this research proposed a combination of RNN and attention mechanisms at the word and word-and-sentence levels of the input document. The results showed an impressive accuracy of 93.72% and an F1 score of 93.7% on the RNN model with a word-and-sentence-level attention mechanism. This research outcome contributes to the field of text classification and could be applied in opinion mining, customer feedback analysis, and natural language processing. Future work aims to enhance sentiment analysis accuracy and expand the models' scope to encompass more languages.

Cybernetics and physics, Nov 30, 2020
The World Wide Web is a large, wealthy, and accessible information system whose users are increas... more The World Wide Web is a large, wealthy, and accessible information system whose users are increasing rapidly nowadays. To retrieve information from the web as per users' requests, search engines are built to access web pages. As search engine systems play a significant role in cybernetics, telecommunication, and physics, many efforts were made to enhance their capacity. However, most of the data contained on the web are unmanaged, making it impossible to access the entire network at once by current search engine system mechanisms. Web Crawler, therefore, is a critical part of search engines to navigate and download full texts of the web pages. Web crawlers may also be applied to detect missing links and for community detection in complex networks and cybernetic systems. However, template-based crawling techniques could not handle the layout diversity of objects from web pages. In this paper, a web crawler module was designed and implemented, attempted to extract article-like contents from 495 websites. It uses a machine learning approach with visual cues, trivial HTML, and text-based features to filter out clutters. The outcomes are promising for extracting article-like contents from websites, contributing to the search engine systems development and future research gears towards proposing higher performance systems.

International journal of engineering trends and technology, Apr 25, 2020
It is complicated to distinguish among thousands of plant species in the natural ecosystem, and m... more It is complicated to distinguish among thousands of plant species in the natural ecosystem, and many efforts have been investigated to address the issue. In Vietnam, the task of identifying one from 12,000 species requires specialized experts in flora management, with thorough training skills and indepth knowledge. Therefore, with the advance of machine learning, automatic plant identification systems have been proposed to benefit various stakeholders, including botanists, pharmaceutical laboratories, taxonomists, forestry services, and organizations. The concept has fueled an interest in research and application from global researchers and engineers in both fields of machine learning and computer vision. In this paper, the Vietnamese plant image dataset was collected from an online encyclopedia of Vietnamese organisms, together with the Encyclopedia of Life, to generate a total of 28,046 environmental images of 109 plant species in Vietnam. A comparative evaluation of four deep convolutional feature extraction models, which are MobileNetV2, VGG16, ResnetV2, and Inception Resnet V2, is presented. Those models have been tested on the Support Vector Machine (SVM) classifier to experiment with the purpose of plant image identification. The proposed models achieve promising recognition rates, and MobilenetV2 attained the highest with 83.9%. This result demonstrates that machine learning models are potential for plant species identification in the natural environment, and future works need to examine proposing higher accuracy systems on a larger dataset to meet the current application demand.

Cybernetics and Physics, 2023
In the current digital era, text documents become valuable for businesses to reach potential cust... more In the current digital era, text documents become valuable for businesses to reach potential customers and curtail advertising costs. However, extracting and classifying beneficial information from texts can prove challenging and time-consuming, particularly in complex languages like Vietnamese. This study aims to classify the sentiment of Vietnamese comments on e-commerce websites into negative and positive classes. To enhance the performance of sentiment classification, the study fine-tuned traditional models of Convolutional Neural Networks and Recurrent Neural Networks (RNN). Then, this research proposed a combination of RNN and attention mechanisms at the word and word-and-sentence levels of the input document. The results showed an impressive accuracy of 93.72% and an F1 score of 93.7% on the RNN model with a word-and-sentence-level attention mechanism. This research outcome contributes to the field of text classification and could be applied in opinion mining, customer feedback analysis, and natural language processing. Future work aims to enhance sentiment analysis accuracy and expand the models’ scope to encompass more languages.

Journal of Universal Computer Science, vol. 29, no. 9 , 2023
The natural ecosystem incorporates thousands of plant species and distinguishing them is normally... more The natural ecosystem incorporates thousands of plant species and distinguishing them is normally manual, complicated, and time-consuming. Since the task requires a large amount of expertise, identifying forest plant species relies on the work of a team of botanical experts. The emergence of Machine Learning, especially Deep Learning, has opened up a new approach to plant classification. However, the application of plant classification based on deep learning models remains limited. This paper proposed a model, named PlantKViT, combining Vision Transformer architecture and the KNN algorithm to identify forest plants. The proposed model provides high efficiency and convenience for adding new plant species. The study was experimented with using Resnet-152, ConvNeXt networks, and the PlantKViT model to classify forest plants. The training and evaluation were implemented on the dataset of DanangForestPlant, containing 10,527 images and 489 species of forest plants. The accuracy of the proposed PlantKViT model reached 93%, significantly improved compared to the ConvNeXt model at 89% and the Resnet-152 model at only 76%. The authors also successfully developed a website and 2 applications called 'plant id' and 'Danangplant' on the iOS and Android platforms respectively. The PlantKViT model shows the potential in forest plant identification not only in the conducted dataset but also worldwide. Future work should gear toward extending the dataset and enhance the accuracy and performance of forest plant identification.

Journal of Universal Computer Science, 2023
The natural ecosystem incorporates thousands of plant species and distinguishing them is normally... more The natural ecosystem incorporates thousands of plant species and distinguishing them is normally manual, complicated, and time-consuming. Since the task requires a large amount of expertise, identifying forest plant species relies on the work of a team of botanical experts. The emergence of Machine Learning, especially Deep Learning, has opened up a new approach to plant classification. However, the application of plant classification based on deep learning models remains limited. This paper proposed a model, named PlantKViT, combining Vision Transformer architecture and the KNN algorithm to identify forest plants. The proposed model provides high efficiency and convenience for adding new plant species. The study was experimented with using Resnet-152, ConvNeXt networks, and the PlantKViT model to classify forest plants. The training and evaluation were implemented on the dataset of DanangForestPlant, containing 10,527 images and 489 species of forest plants. The accuracy of the proposed PlantKViT model reached 93%, significantly improved compared to the ConvNeXt model at 89% and the Resnet-152 model at only 76%. The authors also successfully developed a website and 2 applications called 'plant id' and 'Danangplant' on the iOS and Android platforms respectively. The PlantKViT model shows the potential in forest plant identification not only in the conducted dataset but also worldwide. Future work should gear toward extending the dataset and enhance the accuracy and performance of forest plant identification.

Informatica, 2021
Keyphrase extraction has recently become a foundation for developing digital library applications... more Keyphrase extraction has recently become a foundation for developing digital library applications, especially in semantic information retrieval techniques. From that context, in this paper, a keyphrase extraction model was formulated in terms of Natural Language Processing, applied explicitly in extracting information and searching techniques in tourism. The proposed process includes collecting and processing data from tourism sources such as Tripadvisor.com, Agoda.com, and vietnam-guide.com. Then, the raw data was analyzed and pre-processed with labeling keyphrase and fed data forward to Pretrained BERT model and Bidirectional Long Short-Term Memory with Conditional Random Field. The model performed the combination of Bidirectional Long Short-Term Memory with Conditional Random Field in order to solve keyphrase extraction tasks. Furthermore, the model integrated the Elasticsearch technique to enhance performance and time of looking up tourism destinations' information. The outcome extracted key phrases produce high accuracy and can be applied for extraction problems and textual content summaries. Povzetek: Predstavljen je pristop na osnovi ključnih fraz za uporabo v turističnih sistemih.

Cybernetics and Physics, 2021
Art in general and fine arts, in particular, play a significant role in human life, entertaining ... more Art in general and fine arts, in particular, play a significant role in human life, entertaining and dispelling stress and motivating their creativeness in specific ways. Many well-known artists have left a rich treasure of paintings for humanity, preserving their exquisite talent and creativity through unique artistic styles. In recent years, a technique called ’style transfer’ allows computers to apply famous artistic styles into the style of a picture or photograph while retaining the shape of the image, creating superior visual experiences. The basic model of that process, named ’Neural Style Transfer,’ has been introduced promisingly by Leon A. Gatys; however, it contains several limitations on output quality and implementation time, making it challenging to apply in practice. Based on that basic model, an image transform network was proposed in this paper to generate higher-quality artwork and higher abilities to perform on a larger image amount. The proposed model significant...

Applied Sciences
Due to the alarming rate of climate change, fuel consumption and emission estimates are critical ... more Due to the alarming rate of climate change, fuel consumption and emission estimates are critical in determining the effects of materials and stringent emission control strategies. In this research, an analytical and predictive study has been conducted using the Government of Canada dataset, containing 4973 light-duty vehicles observed from 2017 to 2021, delivering a comparative view of different brands and vehicle models by their fuel consumption and carbon dioxide emissions. Based on the findings of the statistical data analysis, this study makes evidence-based recommendations to both vehicle users and producers to reduce their environmental impacts. Additionally, Convolutional Neural Networks (CNN) and various regression models have been built to estimate fuel consumption and carbon dioxide emissions for future vehicle designs. This study reveals that the Univariate Polynomial Regression model is the best model for predictions from one vehicle feature input, with up to 98.6% accur...

Cybernetics and Physics
The World Wide Web is a large, wealthy, and accessible information system whose users are increas... more The World Wide Web is a large, wealthy, and accessible information system whose users are increasing rapidly nowadays. To retrieve information from the web as per users’ requests, search engines are built to access web pages. As search engine systems play a significant role in cybernetics, telecommunication, and physics, many efforts were made to enhance their capacity.However, most of the data contained on the web are unmanaged, making it impossible to access the entire network at once by current search engine system mechanisms. Web Crawler, therefore, is a critical part of search engines to navigate and download full texts of the web pages. Web crawlers may also be applied to detect missing links and for community detection in complex networks and cybernetic systems. However, template-based crawling techniques could not handle the layout diversity of objects from web pages. In this paper, a web crawler module was designed and implemented, attempted to extract article-like contents...

International Journal of Engineering Trends and Technology
It is complicated to distinguish among thousands of plant species in the natural ecosystem, and m... more It is complicated to distinguish among thousands of plant species in the natural ecosystem, and many efforts have been investigated to address the issue. In Vietnam, the task of identifying one from 12,000 species requires specialized experts in flora management, with thorough training skills and indepth knowledge. Therefore, with the advance of machine learning, automatic plant identification systems have been proposed to benefit various stakeholders, including botanists, pharmaceutical laboratories, taxonomists, forestry services, and organizations. The concept has fueled an interest in research and application from global researchers and engineers in both fields of machine learning and computer vision. In this paper, the Vietnamese plant image dataset was collected from an online encyclopedia of Vietnamese organisms, together with the Encyclopedia of Life, to generate a total of 28,046 environmental images of 109 plant species in Vietnam. A comparative evaluation of four deep convolutional feature extraction models, which are MobileNetV2, VGG16, ResnetV2, and Inception Resnet V2, is presented. Those models have been tested on the Support Vector Machine (SVM) classifier to experiment with the purpose of plant image identification. The proposed models achieve promising recognition rates, and MobilenetV2 attained the highest with 83.9%. This result demonstrates that machine learning models are potential for plant species identification in the natural environment, and future works need to examine proposing higher accuracy systems on a larger dataset to meet the current application demand.

Informatica, 2021
Keyphrase extraction has recently become a foundation for developing digital library applications... more Keyphrase extraction has recently become a foundation for developing digital library applications, especially in semantic information retrieval techniques. From that context, in this paper, a keyphrase extraction model was formulated in terms of Natural Language Processing, applied explicitly in extracting information and searching techniques in tourism. The proposed process includes collecting and processing data from tourism sources such as Tripadvisor.com, Agoda.com, and vietnam-guide.com. Then, the raw data was analyzed and pre-processed with labeling keyphrase and fed data forward to Pretrained BERT model and Bidirectional Long Short-Term Memory with Conditional Random Field. The model performed the combination of Bidirectional Long Short-Term Memory with Conditional Random Field in order to solve keyphrase extraction tasks. Furthermore, the model integrated the Elasticsearch technique to enhance performance and time of looking up tourism destinations' information. The outcome extracted key phrases produce high accuracy and can be applied for extraction problems and textual content summaries. Povzetek: Predstavljen je pristop na osnovi ključnih fraz za uporabo v turističnih sistemih.

MDPI Applied Scienes, 2022
Due to the alarming rate of climate change, fuel consumption and emission estimates are critical ... more Due to the alarming rate of climate change, fuel consumption and emission estimates are critical in determining the effects of materials and stringent emission control strategies. In this research, an analytical and predictive study has been conducted using the Government of Canada dataset, containing 4973 light-duty vehicles observed from 2017 to 2021, delivering a comparative view of different brands and vehicle models by their fuel consumption and carbon dioxide emissions. Based on the findings of the statistical data analysis, this study makes evidence-based recommendations to both vehicle users and producers to reduce their environmental impacts. Additionally, Convolutional Neural Networks (CNN) and various regression models have been built to estimate fuel consumption and carbon dioxide emissions for future vehicle designs. This study reveals that the Univariate Polynomial Regression model is the best model for predictions from one vehicle feature input, with up to 98.6% accuracy. Multiple Linear Regression and Multivariate Polynomial Regression are good models for predictions from multiple vehicle feature inputs, with approximately 75% accuracy. Convolutional Neural Network is also a promising method for prediction because of its stable and high accuracy of around 70%. The results contribute to the quantifying process of energy cost and air pollution caused by transportation, followed by proposing relevant recommendations for both vehicle users and producers. Future research should aim towards developing higher performance models and larger datasets for building APIs and applications.

Cybernetics and Physics, 2021
Art in general and fine arts, in particular, play a significant role in human life, entertaining ... more Art in general and fine arts, in particular, play a significant role in human life, entertaining and dispelling stress and motivating their creativeness in specific ways. Many well-known artists have left a rich treasure of paintings for humanity, preserving their exquisite talent and creativity through unique artistic styles. In recent years, a technique called 'style transfer' allows computers to apply famous artistic styles into the style of a picture or photograph while retaining the shape of the image, creating superior visual experiences. The basic model of that process, named 'Neural Style Transfer,' has been introduced promisingly by Leon A. Gatys; however, it contains several limitations on output quality and implementation time, making it challenging to apply in practice. Based on that basic model, an image transform network was proposed in this paper to generate higher-quality artwork and higher abilities to perform on a larger image amount. The proposed model significantly shortened the execution time and can be implemented in a real-time application, providing promising results and performance. The outcomes are auspicious and can be used as a referenced model in color grading or semantic image segmentation, and future research focuses on improving its applications.
Uploads
Papers by Ngo Le Huy Hien