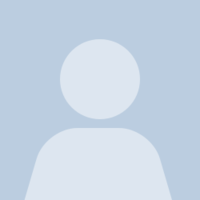
Jaesung Lee
Address: Republic of Korea
less
Related Authors
José M Giménez-García
Universidad de Valladolid
Amin Beheshti
The University of New South Wales
Anudeep C
Massachusetts Institute of Technology (MIT)
Jan Hidders
Birkbeck College, University of London
Christophe Gueret
Accenture Technology Labs
Nigel Shadbolt
University of Southampton
Kesheng Wu
Lawrence Berkeley National Laboratory
Uploads
Papers by Jaesung Lee
present Indexing by latent Dirichlet allocation (LDI), an
automatic document indexing method. Many ad hoc
applications, or their variants with smoothing techniques
suggested in LDA-based language modeling, can
result in unsatisfactory performance as the document
representations do not accurately reflect concept space.
To improve document retrieval performance, we introduce
a new definition of document probability vectors in
the context of LDA and present a novel scheme for
automatic document indexing based on LDA. Second,
we propose an Ensemble Model (EnM) for document
retrieval. EnM combines basic indexing models by
assigning different weights and attempts to uncover the
optimal weights to maximize the mean average precision.
To solve the optimization problem, we propose an
algorithm, which is derived based on the boosting
method. The results of our computational experiments
on benchmark data sets indicate that both the proposed
approaches are viable options for document retrieval.
In this study, we introduce a new definition of document
probability vectors in the context of LDA and present a scheme for automatic document indexing based on it. The results of our computational experiment on a benchmark data set indicate that the proposed approach is a viable option to use in the document indexing. A small illustrative example is also included.
present Indexing by latent Dirichlet allocation (LDI), an
automatic document indexing method. Many ad hoc
applications, or their variants with smoothing techniques
suggested in LDA-based language modeling, can
result in unsatisfactory performance as the document
representations do not accurately reflect concept space.
To improve document retrieval performance, we introduce
a new definition of document probability vectors in
the context of LDA and present a novel scheme for
automatic document indexing based on LDA. Second,
we propose an Ensemble Model (EnM) for document
retrieval. EnM combines basic indexing models by
assigning different weights and attempts to uncover the
optimal weights to maximize the mean average precision.
To solve the optimization problem, we propose an
algorithm, which is derived based on the boosting
method. The results of our computational experiments
on benchmark data sets indicate that both the proposed
approaches are viable options for document retrieval.
In this study, we introduce a new definition of document
probability vectors in the context of LDA and present a scheme for automatic document indexing based on it. The results of our computational experiment on a benchmark data set indicate that the proposed approach is a viable option to use in the document indexing. A small illustrative example is also included.