Papers by shivam tripathi
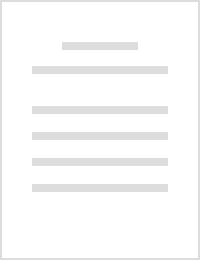
Support Vector Machine Approach to Downscale Precipitation in Climate Change Scenarios
Concern over changes in global climate has increased in recent years with improvement in understa... more Concern over changes in global climate has increased in recent years with improvement in understanding of atmospheric dynamics and growth in evidence of climate link to long‐term variability in hydrologic records. Climate impact studies rely on climate change information at fine spatial resolution. Towards this, the past decade has witnessed significant progress in development of downscaling models to cascade the climate information provided by General Circulation Models (GCMs) at coarse spatial resolution to the scale relevant for hydrologic studies. While a plethora of downscaling models have been applied successfully to mid‐latitude regions, a few studies are available on tropical regions where the atmosphere is known to have more complex behavior. In this paper, a support vector machine (SVM) approach is proposed for statistical downscaling to interpret climate change signals provided by GCMs over tropical regions of India. Climate variables affecting spatio‐temporal variation of precipitation at each meteorological sub‐division of India are identified. Following this, cluster analysis is applied on climate data to identify the wet and dry seasons in each year. The data pertaining to climate variables and precipitation of each meteorological sub‐division is then used to develop SVM based downscaling model for each season. Subsequently, the SVM based downscaling model is applied to future climate predictions from the second generation Coupled Global Climate Model (CGCM2) to assess the impact of climate change on hydrological inputs to the meteorological sub‐divisions. The results obtained from the SVM downscaling model are then analyzed to assess the impact of climate change on precipitation over India.
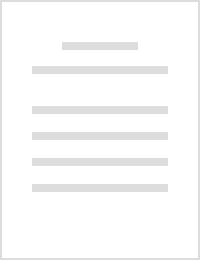
Propagating Uncertainty in Climate Data for Hydrologic Prediction
World Environmental and Water Resources Congress 2008, May 1, 2008
Understanding the impact of climate change and its variability on regional hydrology is predicate... more Understanding the impact of climate change and its variability on regional hydrology is predicated, to a large extent, on availability of long records of high quality climate data. Although recent advances in satellite technology have improved our observation capabilities, past records come from traditional in situ measurements that have large associated uncertainties. Further, the uncertainties in historical observations vary signicantly over space and in time. The information on uncertainty that is available with latest datasets is vastly ignored in the hydrologic literature. Since any relationship linking hydrologic and climatic variables is underpinned by historical data, ignoring this uncertainty information can be misleading. In this study, a methodology is developed to incorporate the observation uncertainty in climate data for hydrologic prediction by combining Bayesian learning theory and the concepts of robust optimization. A Bayesian variant of principal component analysis is used to propagate uncertainties in observations to derive a new representation of data with reduced dimensionality. Robust optimization is then used to obtain the parameters of the prediction model, a linear support vector machine in this case, by accounting for the uncertainty in data representation. The developed methodology is applied to make long-range prediction of Indian summer monsoon rainfall (ISMR) by capturing dynamic relationships between ISMR and sea surface temperature. The advantages of incorporating observation uncertainties for hydrologic predictions are discussed in the light of results obtained.
Application of Relevance Vector Machine for Sediment Transport Estimation
World Environmental and Water Resources Congress 2007, May 11, 2007
ABSTRACT

Water Resources Research, Oct 1, 2008
Principal component analysis (PCA) is the most widely used method for dimensionality reduction, d... more Principal component analysis (PCA) is the most widely used method for dimensionality reduction, data reconstruction, feature extraction, and data visualization in geosciences. However, in its standard form, PCA makes no distinction between data points for which the associated measurement errors vary in both space and time. Using the backdrop of sea surface temperature (SST) data, a Bayesian variant of noisy principal component analysis (BaNPCA) was developed to incorporate observation uncertainty when performing PCA. The algorithm was first assessed using synthetic data sets. Comparison of BaNPCA results with current PCA techniques showed that BaNPCA has lower data reconstruction error; that is, for a given number of principal components, it explains more variance in SST data. Using the automatic relevance determination method, BaNPCA could correctly identify the appropriate number of principal components in the data. BaNPCA was shown to exhibit distinct advantages in filling missing values in the data when compared to existing methods. In addition, the extracted principal vectors from BaNPCA were found to be smoother and more representative of large-scale signals like El Niño-Southern Oscillation and Pacific Decadal Oscillation. To classify extreme states of all India summer monsoon rainfall, we used robust optimization that utilizes the PCs along with computed uncertainty from BaNPCA algorithm as inputs, thus engaging uncertainty in data. Results from this study demonstrate the value of utilizing uncertainty information available with hydrologic data sets.
From flumes to rivers: Can sediment transport in natural alluvial channels be predicted from observations at the laboratory scale?
Water Resources Research, Aug 1, 2009
Doubt regarding the applicability of laboratory results to alluvial streams has led some to devel... more Doubt regarding the applicability of laboratory results to alluvial streams has led some to develop sediment transport predictors based solely on field data, and most current sediment transport formulae have typically been calibrated at least partially on field data. This paper examines the transferability of flume results to the field by exploring the extent to which a unified approach to
Journal of Hydrologic Engineering, Jul 1, 2013

Journal of Hydrology, 2008
For the period of the Barnett Coordinated Campaign, October 16-31, 2013, hourly concentrations fo... more For the period of the Barnett Coordinated Campaign, October 16-31, 2013, hourly concentrations for 46 volatile organic compounds (VOCs) were recorded at 14 air monitoring stations within the Barnett Shale of North Texas. These measurements are used to identify and analyze multi-species hydrocarbon signatures on a regional scale through the novel combination of two techniques: domain filling with Lagrangian trajectories and the machine learning unsupervised classification algorithm called a self-organizing map (SOM). This combination of techniques is shown to accurately identify concentration enhancements in the lightest measured alkane species at and downwind of the locations of activepermit oil and gas facilities, despite the model having This article is part of the Topical Collection on Topical Collection on Geospatial Technology in Environmental Health Applications
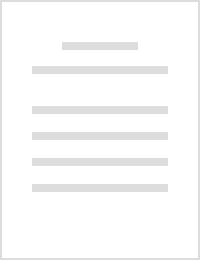
An Analysis of Spatio-Temporal Changes in Drought Characteristics over India
Springer water, 2019
Regional drought mitigation efforts depend on reliable estimates of intensity and severity of dro... more Regional drought mitigation efforts depend on reliable estimates of intensity and severity of drought events. Most operational methods used for drought classification do not account modeling uncertainties and provide discrete drought classification. However, when uncertainty estimates in classification are available, they can be used to make informed decisions. This study compares a gamma-mixture-model-based probabilistic drought classification method that quantifies uncertainties in drought classification with the standardized precipitation index (SPI) that provides discrete classification. Further, if the precipitation data are nonstationary, then classical methods of drought classification are not applicable, and an alternate method for drought classification for trend stationary precipitation series is presented. This method is tested on synthetic and real-world precipitation data over India. The alternate method offers flexibility in modeling nonstationary time series. The advantages and limitations of this method are discussed along with a set of concluding remarks.
Canonical Correlation Analysis for Hydroclimatic Datasets with Known Measurement Uncertainties

Journal of Hydrology, Nov 1, 2006
The Climate impact studies in hydrology often rely on climate change information at fine spatial ... more The Climate impact studies in hydrology often rely on climate change information at fine spatial resolution. However, general circulation models (GCMs), which are among the most advanced tools for estimating future climate change scenarios, operate on a coarse scale. Therefore the output from a GCM has to be downscaled to obtain the information relevant to hydrologic studies. In this paper, a support vector machine (SVM) approach is proposed for statistical downscaling of precipitation at monthly time scale. The effectiveness of this approach is illustrated through its application to meteorological subdivisions (MSDs) in India. First, climate variables affecting spatio-temporal variation of precipitation at each MSD in India are identified. Following this, the data pertaining to the identified climate variables (predictors) at each MSD are classified using cluster analysis to form two groups, representing wet and dry seasons. For each MSD, SVM-based downscaling model (DM) is developed for season(s) with significant rainfall using principal components extracted from the predictors as input and the contemporaneous precipitation observed at the MSD as an output. The proposed DM is shown to be superior to conventional downscaling using multi-layer back-propagation artificial neural networks. Subsequently, the SVM-based DM is applied to future climate predictions from the second generation Coupled Global Climate Model (CGCM2) to obtain future projections of precipitation for the MSDs. The results are then analyzed to assess the impact of climate change on precipitation over India. It is shown that SVMs provide a promising alternative to conventional artificial neural networks for statistical downscaling, and are suitable for conducting climate impact studies.
Forecasting Extremes of Indian Summer Monsoon Rainfall using Bayesian Learning
Indian International Conference on Artificial Intelligence, 2007
Prediction of Flow Regimes in Alluvial Channels
Indian International Conference on Artificial Intelligence, 2009
A Machine Learning Approach for Probabilistic Drough Classification

Journal of Hydrologic Engineering, Dec 1, 2011
Climatic variables that are used as inputs in hydrologic models often have large measurement unce... more Climatic variables that are used as inputs in hydrologic models often have large measurement uncertainties that are mostly ignored in hydrologic applications because of lack of appropriate tools. This study develops a set of algorithms to engage uncertainty information in three of the most common statistical procedures applied on climatic data, namely correlation (BaNCorr), principal component analysis (VBaNPCA), and regression (VNRVM). These new algorithms are developed within a common framework of Bayesian learning, and together they provide a comprehensive tool to account for uncertainty in various stages of model development. The developed algorithms are first tested and compared with traditional methods and state-of-the-art algorithms on synthetic data. Practical application of the proposed algorithms is demonstrated by developing a seasonal prediction model for all India summer monsoon rainfall by using sea surface temperature (SST) data and associated measurement errors as inputs. The results suggest that incorporating measurement errors in hydrologic models improves their prediction performance and provides better assessment of their predictive capabilities.
On the identification of intra-seasonal changes in the Indian summer monsoon
On the Identification of Intra-seasonal Changes in the Indian Summer Monsoon Shivam Tripathi and ... more On the Identification of Intra-seasonal Changes in the Indian Summer Monsoon Shivam Tripathi and Rao S. Govindaraju School of Civil Engineering, Purdue University West Lafayette, Indiana, USA [email protected] ... Evi-dently, correlations up to four days lag are significant. ...

Sachetas
The following paper discusses the introduction to Central Bank Digital Currency (CBDC) and how th... more The following paper discusses the introduction to Central Bank Digital Currency (CBDC) and how the adoption of such a measure will result in a developing economy like India looking at past references. The paper aims to reach new measures of adoption procedure and why it becomes essential to bring out such a concept at an early age. Various documents and research on technologies have proved the significance of virtual currencies. The social media forums which are abuzz with such investment options as provided by cryptocurrency market leads to a talk on potential regulation of the market for fiscal benefits. The committee developing such a digital currency has many options at hand, like hash graph or blockchain, POW or POS framework, adopting ABFT or not and others. The paper concludes determining the best measures a committee can adopt to frame CBDCs, discussing the pros and cons while timing the launch based on certain indices available yet lesser known in general.
Impact of renewed solar dimming on streamflow generation in monsoon dominated tropical river basins
Journal of Hydro-environment Research, 2022
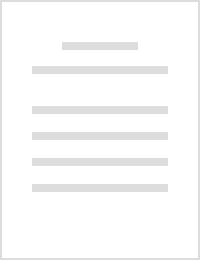
Detection of Temporal Changes in Droughts Over Indiana
Trends and Changes in Hydroclimatic Variables, 2019
Classification of droughts based on their intensity and severity is essential for regional planni... more Classification of droughts based on their intensity and severity is essential for regional planning of drought mitigation efforts. Most of the operational methods for drought classification do not account modeling uncertainties and provide discrete drought classification. However, when uncertainty estimates in classification are available they can be used to make informed decisions. This study compares two probabilistic drought classification methods (hidden Markov model and Gamma mixture model–based methods) that quantify uncertainties in drought classification with the standardized precipitation index (SPI) that provides discrete classification. The three methods are applied to study meteorological droughts over Indiana. Comparison among model results showed important differences among the methods, particularly when SPI assumptions on data distribution are violated. Further, trend analysis of precipitation data revealed that many stations in Indiana have significant trends, and he...
SPH Simulation of Impact of a Surge on a Wall
Uploads
Papers by shivam tripathi