Papers by priya chakriswaran
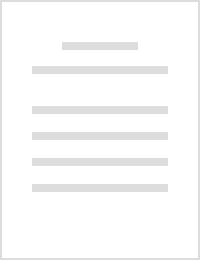
Ensemble of Artificial Intelligence Techniques for Bacterial Antimicrobial Resistance (AMR) Estimation Using Topic Modeling and Similarity Measure
International Journal of Uncertainty, Fuzziness and Knowledge-Based Systems
In recent times, bacterial Antimicrobial Resistance (AMR) analyses becomes a hot study topic. The... more In recent times, bacterial Antimicrobial Resistance (AMR) analyses becomes a hot study topic. The AMR comprises information related to the antibiotic product name, class name, subclass name, type, subtype, gene type, etc., which can fight against the illness. However, the tagging language used to determine the data is of free context. These contexts often contain ambiguous data, which leads to a hugely challenging issue in retrieving, organizing, merging, and finding the relevant data. Manually reading this text and labelling is not time-consuming. Hence, topic modeling overcomes these challenges and provides efficient results in categorizing the topic and in determining the data. In this view, this research work designs an ensemble of artificial intelligence for categorizing the AMR gene data and determine the relationship between the antibiotics. The proposed model includes a weighted voting based ensemble model by the incorporation of Latent Dirichlet Allocation (LDA) and Hierarc...

Applied Sciences
The essential use of natural language processing is to analyze the sentiment of the author via th... more The essential use of natural language processing is to analyze the sentiment of the author via the context. This sentiment analysis (SA) is said to determine the exactness of the underlying emotion in the context. It has been used in several subject areas such as stock market prediction, social media data on product reviews, psychology, judiciary, forecasting, disease prediction, agriculture, etc. Many researchers have worked on these areas and have produced significant results. These outcomes are beneficial in their respective fields, as they help to understand the overall summary in a short time. Furthermore, SA helps in understanding actual feedback shared across different platforms such as Amazon, TripAdvisor, etc. The main objective of this thorough survey was to analyze some of the essential studies done so far and to provide an overview of SA models in the area of emotion AI-driven SA. In addition, this paper offers a review of ontology-based SA and lexicon-based SA along wit...

Applied Sciences, Dec 12, 2019
The essential use of natural language processing is to analyze the sentiment of the author via th... more The essential use of natural language processing is to analyze the sentiment of the author via the context. This sentiment analysis (SA) is said to determine the exactness of the underlying emotion in the context. It has been used in several subject areas such as stock market prediction, social media data on product reviews, psychology, judiciary, forecasting, disease prediction, agriculture, etc. Many researchers have worked on these areas and have produced significant results. These outcomes are beneficial in their respective fields, as they help to understand the overall summary in a short time. Furthermore, SA helps in understanding actual feedback shared across different platforms such as Amazon, TripAdvisor, etc. The main objective of this thorough survey was to analyze some of the essential studies done so far and to provide an overview of SA models in the area of emotion AI-driven SA. In addition, this paper offers a review of ontology-based SA and lexicon-based SA along with machine learning models that are used to analyze the sentiment of the given context. Furthermore, this work also discusses different neural network-based approaches for analyzing sentiment. Finally, these different approaches were also analyzed with sample data collected from Twitter. Among the four approaches considered in each domain, the aspect-based ontology method produced 83% accuracy among the ontology-based SAs, the term frequency approach produced 85% accuracy in the lexicon-based analysis, and the support vector machine-based approach achieved 90% accuracy among the other machine learning-based approaches.
Uploads
Papers by priya chakriswaran