Papers by mokhtar mohammadi

Wireless Personal Communications, 2021
The early diagnosis and the accurate separation of COVID-19 from non-COVID-19 cases based on pulm... more The early diagnosis and the accurate separation of COVID-19 from non-COVID-19 cases based on pulmonary diffuse airspace opacities is one of the challenges facing researchers. Recently, researchers try to exploit the Deep Learning (DL) method's capability to assist clinicians and radiologists in diagnosing positive COVID-19 cases from chest X-ray images. In this approach, DL models, especially Deep Convolutional Neural Networks (DCNN), propose real-time, automated effective models to detect COVID-19 cases. However, conventional DCNNs usually use Gradient Descent-based approaches for training fully connected layers. Although GD-based Training (GBT) methods are easy to implement and fast in the process, they demand numerous manual parameter tuning to make them optimal. Besides, the GBT's procedure is inherently sequential, thereby parallelizing them with Graphics Processing Units is very difficult. Therefore, for the sake of having a real-time COVID-19 detector with parallel implementation capability, this paper proposes the use of the Whale Optimization Algorithm for training fully connected layers. The designed detector is then benchmarked on a verified dataset called COVID-Xray-5k, and the results are verified by a comparative study with classic DCNN, DUICM, and Matched Subspace classifier with Adaptive Dictionaries. The results show that the proposed model with an average accuracy of 99.06% provides 1.87% better performance than the best comparison model. The paper also considers the concept of Class Activation Map to detect the regions potentially infected by the virus. This was found to correlate with clinical results, as confirmed by experts. Although results are auspicious, further investigation is needed on a larger dataset of COVID-19 images to have a more comprehensive evaluation of accuracy rates.
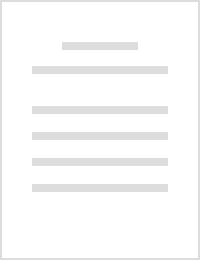
A new model for analyzing the role of new ICT-based technologies on the success of employees' learning programs
Kybernetes, 2021
Purpose The concept of e-learning is essential in employee education since it provides different ... more Purpose The concept of e-learning is essential in employee education since it provides different ways to develop employees' knowledge, skills and attitudes using modern technologies. E-learning has been overgrowing in employee education because learning can be held anytime and anywhere. In order to succeed in implementing e-learning and benefiting from its capacities, and avoiding potential threats in the country, it is necessary to address the factors affecting its success. This paper aims to test the role of internet of Things (IoT)-based systems, cloud-based services, virtual classes, evaluation tools, attitude, content management and creativity on the success of employees' e-learning programs based on a framework. Design/methodology/approach E-learning systems receive ever-increasing attention in academia, business and public administration. With the development of e-learning, employee education has also benefited from its capacities in various fields. To succeed in impl...
Artificial Intelligence: Theory and Applications, 2021
One popular example of metaheuristic algorithms from the swarm intelligence family is the Bat alg... more One popular example of metaheuristic algorithms from the swarm intelligence family is the Bat algorithm (BA). The algorithm was first presented in 2010 by Yang and quickly demonstrated its efficiency in comparison with other common algorithms. The BA is based on echolocation in bats. The BA uses automatic zooming to strike a balance between exploration and exploitation by imitating the deviations of the bat's pulse emission rate and loudness as it searches for prey. The BA maintains solution diversity using the frequency-tuning technique. In this way, the BA can quickly and efficiently switch from exploration to exploitation. Therefore, it becomes an efficient optimizer for any application when a quick solution is needed. In this

Mobile Edge Computing (MEC) is an interesting technology aimed at providing various processing an... more Mobile Edge Computing (MEC) is an interesting technology aimed at providing various processing and storage resources at the edge of the Internet of things (IoT) networks. However, MECs contain limited resources, and they should be managed effectively to improve resource utilization. Workflow scheduling is a process that tries to map the workflow tasks to the most proper set of computing resources regarding some objectives. For this purpose, this paper presents DBOA, a discrete version of the Butterfly Optimization Algorithm (BOA) that applies the Levy flight to improve its convergence speed and prevent the local optima problem. Then, DBOA is applied for DVFS-based data-intensive workflow scheduling and data placement in MEC environments. This scheme also employs the HEFT algorithm's task prioritization method to find the task execution order in the scientific workflows. For evaluating the performance of the proposed scheduling scheme, extensive simulations are conducted on vario...

ArXiv, 2020
Mental disorders represent critical public health challenges as they are leading contributors to ... more Mental disorders represent critical public health challenges as they are leading contributors to the global burden of disease and intensely influence social and financial welfare of individuals. The present comprehensive review concentrate on the two mental disorders: Major depressive Disorder (MDD) and Bipolar Disorder (BD) with noteworthy publications during the last ten years. There's a big need nowadays for phenotypic characterization of psychiatric disorders with biomarkers. Electroencephalography (EEG) signals could offer a rich signature for MDD and BD and then they could improve understanding of pathophysiological mechanisms underling these mental disorders. In this work, we focus on the literature works adopting neural networks fed by EEG signals. Among those studies using EEG and neural networks, we have discussed a variety of EEG based protocols, biomarkers and public datasets for depression and bipolar disorder detection. We conclude with a discussion and valuable re...
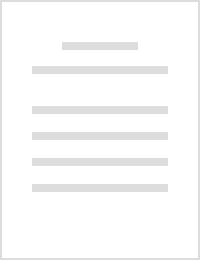
Predicting construction time and cost of tunnels using Markov chain model considering opinions of experts
Tunnelling and Underground Space Technology, 2021
Abstract The impact of uncertainty on decision-making is increasingly prioritized, especially for... more Abstract The impact of uncertainty on decision-making is increasingly prioritized, especially for large geotechnical projects such as tunnels. In this article, the effects of geological/geotechnical uncertainties in tunnel construction time and costs were reduced. The forecasting of geological/geotechnical conditions in a tunnel project was based on the Markov chain. To accessing the more accurate input data of the Markov chain and estimate the construction time and cost required for tunnel construction, tunneling experts were interviewed through a questionnaire. Also, to assess the updating effect on obtained outcomes during the tunnel construction, the model was updated for one time. Finally, comparing the predicted results with the actual results, the effects of the interviewing and updating process were investigated. According to the results, the uncertainties in the predictions were found to be reduced significantly. Lastly, this research can provide guidance or reference for the evaluation and decision-making of complex systems engineering problems, and indicate a direction for further research of risk assessment and decision-making issues.
IEEE Access, 2021
The result was created in solving the standard project no. 7429/2020/02 System approach to select... more The result was created in solving the standard project no. 7429/2020/02 System approach to selected information and communications technology trends'' using institutional support for long-term conceptual development of research of the University of Finance and Administration.
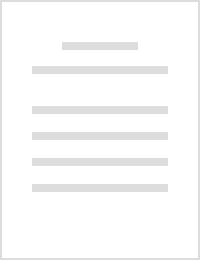
Dynamic prediction models of rock quality designation in tunneling projects
Transportation Geotechnics, 2021
Abstract Machine learning (ML) is becoming an appealing tool in various fields of civil engineeri... more Abstract Machine learning (ML) is becoming an appealing tool in various fields of civil engineering, such as tunneling. A very important issue in tunneling is to know the geological condition of the tunnel route before the construction. Various geological and geotechnical parameters can be considered according to data availability to define tunnels' ground conditions. The Rock Quality Designation (RQD) is one of the most important parameters that are very effective in tunnel geology. This article aims to maximize the prediction accuracy of the RQD parameter along a tunnel route through continuous updating techniques. For this purpose, four ML methods of K-nearest neighbor (KNN), Gaussian Process Regression (GPR), Support Vector Regression (SVR), and Decision Tree (DT) were considered. All the RQD observations along the tunnel route were considered as the models’ inputs. For predicting the RQD status along the entire tunnel route, the ML models use the regression technique. For checking the applicability of the models, the Hamru road tunnel in Iran was used. The models were updated twice to assess the update effect on the results achieved during the tunnel construction. In each prediction phase, all the prediction results were compared using different statistical evaluation criteria and the actual mode. Finally, the comparative tests' findings showed that predictions of the GPR model with R2 = 0.8746/root mean square error (RMSE) = 3.5942101, R2 = 0.9328/RMSE = 2.5580977, and R2 = 0.9433/RMSE = 1.8016325 are generally well-suited to actual results for pre-update, first update, and second update phases, respectively. The updating procedure also leads to prediction models that are more accurate and less uncertain than the previous prediction stage.
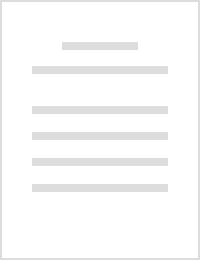
Artificial intelligence forecasting models of uniaxial compressive strength
Transportation Geotechnics, 2021
Abstract The uniaxial compressive strength (UCS) is a vital rock geomechanical parameter widely u... more Abstract The uniaxial compressive strength (UCS) is a vital rock geomechanical parameter widely used in rock engineering projects such as tunnels, dams, and rock slope stability. Since the acquisition of high-quality core samples is not always possible, researchers often indirectly estimate these parameters. The main objective of the present study is to evaluate the performance of the long short term memory (LSTM), deep neural networks (DNN), K-nearest neighbor (KNN), Gaussian process regression (GPR), support vector regression (SVR), and decision tree (DT) to predict the UCS of different rock types of Claystone, Granite, Schist and Sandstone, Travertine, Limestone, Slate, Dolomite and Marl acquired from almost all quarry locations of Iran. 170 data sets, including porosity (n), Schmidt hammer (SH), P-wave velocity (Vp), and point load index (Is(50)) were applied in the methods. Finally, a comparison was made between the results made by the prediction methods. To assess the performance ability of the applied methods, the 5-fold cross-validation (CV) was considered. The results proved that computational intelligence approaches are capable of predicting UCS. On the whole, the GPR with a correlation coefficient (R2) of 0.9955 and a route mean square error (RMSE) of 0.52169, performs best. Lastly, the UCS prediction intelligence methods were ordered as GPR, DT, SVR, LSTM, DNN and KNN, respectively.

The ever-increasing complication and severity of the computer networks' security attacks have... more The ever-increasing complication and severity of the computer networks' security attacks have inspired security researchers to apply various machine learning methods to protect the organizations' data and reputation. Deep learning is one of the exciting techniques that recently have been widely used by intrusion detection systems (IDS) to secure computer networks and hosts' performance. This survey article focuses on the signature-based IDS using deep learning techniques and puts forward an in-depth survey and classification of these schemes. For this purpose, it first presents the essential background concepts about IDS architecture and various deep learning techniques. It then classifies these schemes according to the type of deep learning methods applied in each of them. It describes how deep learning networks are utilized in the misuse detection process to recognize intrusions accurately. Finally, a complete analysis of the investigated IDS frameworks is provided, an...

Soft Computing, 2021
The COVID19 pandemic globally and significantly has affected the life and health of many communit... more The COVID19 pandemic globally and significantly has affected the life and health of many communities. The early detection of infected patients is effective in fighting COVID19. Using radiology (X-Ray) images is, perhaps, the fastest way to diagnose the patients. Thereby, deep Convolutional Neural Networks (CNNs) can be considered as applicable tools to diagnose COVID19 positive cases. Due to the complicated architecture of a deep CNN, its real-time training and testing become a challenging problem. This paper proposes using the Extreme Learning Machine (ELM) instead of the last fully connected layer to address this deficiency. However, the parameters' stochastic tuning of ELM's supervised section causes the final model unreliability. Therefore, to cope with this problem and maintain network reliability, the sine-cosine algorithm was utilized to tune the ELM's parameters. The designed network is then benchmarked on the COVID-Xray-5k dataset, and the results are verified by a comparative study with canonical deep CNN, ELM optimized by cuckoo search, ELM optimized by genetic algorithm, and ELM optimized by whale optimization algorithm. The proposed approach outperforms comparative benchmarks with a final accuracy of 98.83% on the COVID-Xray-5k dataset, leading to a relative error reduction of 2.33% compared to a canonical deep CNN. Even more critical, the designed network's training time is only 0.9421 ms and the overall detection test time for 3100 images is 2.721 s. Keywords COVID19 Á Deep convolutional neural networks Á Sine-cosine algorithm Á Extreme learning machine Á Chest X-ray images Communicated by Victor Hugo C. de Albuquerque.

Automation in Construction, 2020
Accurate estimation of shape thickness from medical images is crucial in clinical applications. F... more Accurate estimation of shape thickness from medical images is crucial in clinical applications. For example, the thickness of myocardium is one of the key to cardiac disease diagnosis. While mathematical models are available to obtain accurate dense thickness estimation, they suffer from heavy computational overhead due to iterative solvers. To this end, we propose novel methods for dense thickness estimation, including a fast solver that estimates thickness from binary annular shapes and an end-to-end network that estimates thickness directly from raw cardiac images.We test the proposed models on three cardiac datasets and one synthetic dataset, achieving impressive results and generalizability on all. Thickness estimation is performed without iterative solvers or manual correction, which is 100× faster than the mathematical model. We also analyze thickness patterns on different cardiac pathologies with a standard clinical model and the results demonstrate the potential clinical value of our method for thickness based cardiac disease diagnosis.
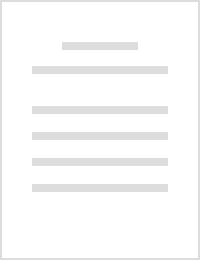
Hearing loss prevalence and years lived with disability, 1990–2019: findings from the Global Burden of Disease Study 2019
The Lancet, 2021
Summary Background Hearing loss affects access to spoken language, which can affect cognition and... more Summary Background Hearing loss affects access to spoken language, which can affect cognition and development, and can negatively affect social wellbeing. We present updated estimates from the Global Burden of Disease (GBD) study on the prevalence of hearing loss in 2019, as well as the condition's associated disability. Methods We did systematic reviews of population-representative surveys on hearing loss prevalence from 1990 to 2019. We fitted nested meta-regression models for severity-specific prevalence, accounting for hearing aid coverage, cause, and the presence of tinnitus. We also forecasted the prevalence of hearing loss until 2050. Findings An estimated 1·57 billion (95% uncertainty interval 1·51–1·64) people globally had hearing loss in 2019, accounting for one in five people (20·3% [19·5–21·1]). Of these, 403·3 million (357·3–449·5) people had hearing loss that was moderate or higher in severity after adjusting for hearing aid use, and 430·4 million (381·7–479·6) without adjustment. The largest number of people with moderate-to-complete hearing loss resided in the Western Pacific region (127·1 million people [112·3–142·6]). Of all people with a hearing impairment, 62·1% (60·2–63·9) were older than 50 years. The Healthcare Access and Quality (HAQ) Index explained 65·8% of the variation in national age-standardised rates of years lived with disability, because countries with a low HAQ Index had higher rates of years lived with disability. By 2050, a projected 2·45 billion (2·35–2·56) people will have hearing loss, a 56·1% (47·3–65·2) increase from 2019, despite stable age-standardised prevalence. Interpretation As populations age, the number of people with hearing loss will increase. Interventions such as childhood screening, hearing aids, effective management of otitis media and meningitis, and cochlear implants have the potential to ameliorate this burden. Because the burden of moderate-to-complete hearing loss is concentrated in countries with low health-care quality and access, stronger health-care provision mechanisms are needed to reduce the burden of unaddressed hearing loss in these settings. Funding Bill & Melinda Gates Foundation and WHO.
Detection of Frequency Modulated Signals Using a Robust IF Estimation Algorithm
Circuits, Systems, and Signal Processing, 2019
This paper presents a methodology to detect non-stationary frequency modulated signals under nois... more This paper presents a methodology to detect non-stationary frequency modulated signals under noise process uncertainty. The proposed method uses the combination of adaptive directional time–frequency distribution and modified Viterbi algorithm to robustly estimate the instantaneous frequency (IF) of the given signal. This IF information is then used to remove the frequency modulation from the given signal thus converting it into temporally correlated signal. This temporal correlation is then exploited to detect signals under noise power uncertainty. The effectiveness of the proposed detector is shown through numerical simulations.
Multidimensional Systems and Signal Processing, 2022
In this article the affiliation of Author Mokhtar Mohammadi was incorrectly given as 'Depart-

Detection of event-related potentials (ERPs) in electroencephalography (EEG) is of great interest... more Detection of event-related potentials (ERPs) in electroencephalography (EEG) is of great interest in the study of brain responses to various stimuli. This is challenging due to the low signal-to-noise ratio of these deflections. To address this problem, a new scheme to detect the ERPs based on smoothness priors is proposed. Methods: The problem is considered as a binary hypothesis test and solved using a smooth version of the generalized likelihood ratio test (SGLRT). First, we estimate the parameters of probability density functions from the training data under Gaussian assumption. Then, these parameters are treated as known values and the unknown ERPs are estimated under the smoothness constraint. The performance of the proposed SGLRT is assessed for ERP detection in poststimuli EEG recordings of two oddball settings. We compared our method with several powerful methods regarding ERP detection. Results: The presented method outperforms the competing algorithms and improves the classification accuracy. Conclusion: The proposed SGLRT could be employed as a powerful means for different ERP detection schemes. Significance: ERP-based systems (e.g. brain-machine interfaces) mainly suffer from the lack of classification accuracy, hence, the proposed method is an important step toward real-life applicability of these systems.
Hearing loss prevalence and years lived with disability, 1990–2019: findings from the Global Burden of Disease Study 2019
The Lancet, 2021
Background Hearing loss affects access to spoken language, which can affect cognition and develop... more Background Hearing loss affects access to spoken language, which can affect cognition and development, and can negatively affect social wellbeing. We present updated estimates from the Global Burden of Disease (GBD) study on the prevalence of hearing loss in 2019, as well as the condition's associated disability.
Forecasting tunnel geology, construction time and costs using machine learning methods
Neural Computing and Applications

Mathematics
This paper works on one of the most recent pedestrian crowd evacuation models—i.e., “a simulation... more This paper works on one of the most recent pedestrian crowd evacuation models—i.e., “a simulation model for pedestrian crowd evacuation based on various AI techniques”—which was developed in late 2019. This study adds a new feature to the developed model by proposing a new method and integrating it into the model. This method enables the developed model to find a more appropriate evacuation area design regarding safety due to selecting the best exit door location among many suggested locations. This method is completely dependent on the selected model’s output—i.e., the evacuation time for each individual within the evacuation process. The new method finds an average of the evacuees’ evacuation times of each exit door location; then, based on the average evacuation time, it decides which exit door location would be the best exit door to be used for evacuation by the evacuees. To validate the method, various designs for the evacuation area with various written scenarios were used. Th...
Forecasting sidewall displacement of underground caverns using machine learning techniques
Automation in Construction
Uploads
Papers by mokhtar mohammadi