Papers by Zahra Dashtbozorgi

Acta Physico-Chimica Sinica, 2017
The purpose of this study was to develop a quantitative structure-property relationship (QSPR) mo... more The purpose of this study was to develop a quantitative structure-property relationship (QSPR) model based on the enhanced replacement method (ERM) and support vector machine (SVM) to predict the blood-to-brain barrier partitioning behavior (logBB) of various drugs and organic compounds. Different molecular descriptors were calculated using a dragon package to represent the molecular structures of the compounds studied. The enhanced replacement method (ERM) was used to select the variables and construct the SVM model. The correlation coefficient, R 2 , between experimental results and predicted logBB was 0.878 and 0.986, respectively. The results obtained demonstrated that, for all compounds, the logBB values estimated by SVM agreed with the experimental data, demonstrating that SVM is an effective method for model development, and can be used as a powerful chemometric tool in QSPR studies.
Journal of Separation Science, 2017
A prediction of quantitative structure-property relationships is developed to model the polarity ... more A prediction of quantitative structure-property relationships is developed to model the polarity parameter of a set of 146 organic compounds in acetonitrile in reversed-phase liquid chromatography. Enhanced replacement method and support vector machine regressions were employed to build prediction models based on molecular descriptors calculated from the structure alone. The correlation coefficients between experimental and predicted values of polarity parameter for the test set by enhanced replacement method and support vector machine were 0.970 and 0.993 respectively. The obtained results demonstrated that the support vector machine model is more reliable and has a better prediction performance than enhanced replacement method.

Global Journal of Physical Chemistry, Feb 20, 2012
In the present work, partial least squares (PLS), artificial neural network (ANN) and support vec... more In the present work, partial least squares (PLS), artificial neural network (ANN) and support vector machine (SVM) techniques were used for quantitative structure-property relationship (QSPR) studies of gas to carbon tetrachloride solvation enthalpy (ΔH solv) of various organic compounds based on molecular descriptors calculated from the optimized structures. Different kinds of molecular descriptors were calculated to characterize the molecular structures of compounds, such as constitutional, topological, charge, and geometric descriptors. The variable selection method of genetic algorithm-partial least squares (GA-PLS) was employed to select most favorable subset of descriptors. The five descriptors selected using GA-PLS were used as inputs of ANN and SVM to predict the gas to carbon tetrachloride solvation enthalpy. The correlation coefficients, R, between experimental and predicted solvation enthalpy for the test set by PLS, ANN and SVM are 0.922, 0.985 and 0.990 respectively. Satisfactory results indicated that the GA-PLS approach is a very effective method for variable selection and the predictive ability of the SVM model is superior to those obtained by PLS and ANN. The obtained results demonstrate that SVM can be used as a substitute powerful modeling tool for QSPR studies.

Journal of Structural Chemistry, 2012
An artificial neural network (ANN) is constructed and trained for the prediction of gas to water ... more An artificial neural network (ANN) is constructed and trained for the prediction of gas to water partition coefficients of various organic compounds. The inputs of this neural network are theoretically derived from molecular descriptors that were chosen by the genetic algorithmpartial least squares (GA-PLS) feature selection technique. These descriptors are: areaweighted surface charge of hydrogen bonding donor atoms (HDCA-2), average bond order of a C atom (P C), Kier flexibility index ()), atomic charge weighted partial positively charged surface area (PPSA-3), and difference between atomic charge weighted partial positive and negative surface areas (DPSA-3). By comparing the results obtained from PLS and ANN models, one can see that statistical parameters (Fisher ratio, correlation coefficient, and standard error) of the ANN model are better than those of the PLS model, which indicates that a nonlinear model can simulate more accurately the relationship between the structural descriptors and the partition coefficients of the investigated molecules. K e y w o r d s: artificial neural network, gas to water partition coefficient, genetic algorithm, partial least squares.
Journal of the Chilean Chemical Society, 2013
A rapid, specific and sensitive multi-residue method based on the quick, east, cheap, effective, ... more A rapid, specific and sensitive multi-residue method based on the quick, east, cheap, effective, rugged and safe (QuEChERS) has been developed and validated for the determination of 19 multi-class insecticide and fungicide residues (Aldrin
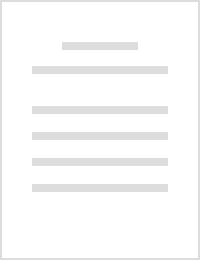
QSPR Study of the Solute Polarity Parameter in Reversed-Phase Liquid Chromatography Using Partial Least Squares and Artificial Neural Network
Journal of Liquid Chromatography & Related Technologies, 2012
A quantitative structure-property relationship (QSPR) study based on artificial neural network (A... more A quantitative structure-property relationship (QSPR) study based on artificial neural network (ANN) was carried out for the prediction of the solute polarity parameters of a set of 146 compounds of a very different chemical nature in reversed-phase liquid chromatography (RPLC). The genetic algorithm-partial least squares (GA-PLS) method was applied as a variable selection tool. A PLS method was used to select the best descriptors and the selected descriptors were used as input neurons in neural network model. These descriptors are: Topological electronic index (), Total hybridization components of the molecular dipole (μh), Total dipole moment of the molecule (μ), PPSA-3 Atomic charge weighted PPSA (PPSA-3), Kier&Hall index, order 0 (χ) and Molecular volume (MV). The results obtained showed the ability of developed artificial neural network to predict the solute polarity parameter of various compounds. Also results reveal the superiority of the ANN over the PLS model.

Computational Toxicology, 2017
A quantitative structure property relationship (QSPR) study based on enhanced replacement method ... more A quantitative structure property relationship (QSPR) study based on enhanced replacement method (ERM) and support vector machine (SVM) was used to correlate molecular structures to their bovine serum albumin water partition coefficients (K BSA/W). A wide variety of natural organic compounds and drugs were selected as a dataset and suitable sets of molecular descriptors were calculated using Dragon package. ERM was used as variable selection method. The nonlinear-support vector machine models were applied to correlate the ERMselected molecular descriptors with the experimental values of K BSA/W. Results obtained demonstrate the reliability and good predictability of support vector machine model to predict K BSA/W of organic compounds and drugs. Satisfactory results demonstrate that the ERM approach is a very powerful method for variable selection and the predictive ability of the SVM model is superior to those acquired by ERM.
Russian Journal of Physical Chemistry A, 2016
The purpose of this paper is to present a novel way for developing quantitative structure-propert... more The purpose of this paper is to present a novel way for developing quantitative structure-property relationship (QSPR) models to predict the gas-to-propanol solvation enthalpy (ΔH solv) of 95 organic compounds. Different kinds of descriptors were calculated for each compound using the Dragon software package. The variable selection technique of replacement method (RM) was employed to select the optimal subset of solute descriptors. Our investigation reveals that the dependence of physical chemistry properties of solution on solvation enthalpy is nonlinear and that the RM method is unable to model the solvation enthalpy accurately. The results established that the calculated ΔH solv values by SVM were in good agreement with the experimental ones, and the performances of the SVM models were superior to those obtained by RM model.
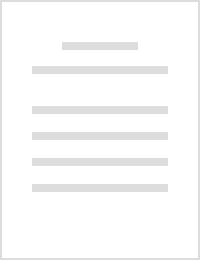
QSPR prediction of gas-to-methanol solvation enthalpy of organic compounds using replacement method and support vector machine
Physics and Chemistry of Liquids, 2014
ABSTRACT A quantitative structure–property relationship (QSPR) study was performed for the predic... more ABSTRACT A quantitative structure–property relationship (QSPR) study was performed for the prediction of the gas-to-methanol solvation enthalpy (ΔHSolv) of a set of 176 organic compounds based on molecular descriptors calculated solely from the molecular structure. The novel variable selection technique of replacement method (RM) was used to select an optimum subset of descriptors and the selected descriptors were used as inputs for constructing a support vector machine (SVM) model. The correlation coefficients, R, between experimental and predicted ΔHSolv for the prediction set by RM and SVM methods are 0.953 and 0.991, respectively. The results demonstrated that the calculated ΔHSolv values by SVM were in good agreement with the experimental ones, and the performance of the SVM model was superior to RM approach. This study shows that the RM is a novel and effective method for selecting the best descriptors, and can be used as a powerful chemometrics tool in QSPR studies.

Journal of Chemistry, 2013
A quantitative structure-retention relationships (QSRRs) method is employed to predict the retent... more A quantitative structure-retention relationships (QSRRs) method is employed to predict the retention time of 300 pesticide residues in animal tissues separated by gas chromatography-mass spectroscopy (GC-MS). Firstly, a six-parameter QSRR model was developed by means of multiple linear regression. The six molecular descriptors that were considered to account for the effect of molecular structure on the retention time are number of nitrogen, Solvation connectivity index-chi 1, BalabanYindex, Moran autocorrelation-lag 2/weighted by atomic Sanderson electronegativity, total absolute charge, and radial distribution function-6.0/unweighted. A 6-7-1 back propagation artificial neural network (ANN) was used to improve the accuracy of the constructed model. The standard error values of ANN model for training, test, and validation sets are 1.559, 1.517, and 1.249, respectively, which are less than those obtained reveals by multiple linear regressions model (2.402, 1.858, and 2.036, resp.). R...
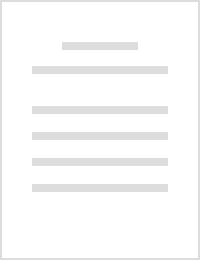
Prediction of gas to water solvation enthalpy of organic compounds using support vector machine
Thermochimica Acta, 2012
ABSTRACT a b s t r a c t Quantitative structure–property relationship (QSPR) models were develope... more ABSTRACT a b s t r a c t Quantitative structure–property relationship (QSPR) models were developed to predict gas to water solvation enthalpy (H Solv) of various organic compounds based on physico-chemical descriptors. Six molecular descriptors selected by genetic algorithm (GA) feature selection technique were used as inputs to perform partial least squares (PLS), artificial neural network (ANN) and support vector machine (SVM) studies. The correlation coefficient (R) between experimental and predicted solvation enthalpy for pre-diction sets by PLS, ANN and SVM are 0.935, 0.990 and 0.993, respectively. The results demonstrated that the calculated H Solv values by SVM were in good agreement with the experimental ones, and the per-formances of the SVM models were comparable or superior to those of PLS and ANN ones. This indicates that SVM can be used as an alternative modeling tool for quantitative structure–property relationship (QSPR) studies.

Structural Chemistry, 2013
Support vector machines (SVMs), as a novel type of learning machine, were used to develop a quant... more Support vector machines (SVMs), as a novel type of learning machine, were used to develop a quantitative structure-property relationship (QSPR) model to predict the gas-to-heptane and gas-to-hexadecane solvation enthalpies (DH Solv) of various organic compounds based on molecular descriptors calculated from the structure alone. Partial least squares (PLSs) and artificial neural network (ANN) were also employed to create linear and nonlinear models to compare with the results attained by SVM. The correlation coefficients, R, between experimental and predicted values of gas-to-heptane solvation enthalpy for the test set by PLS; ANN; and SVM were 0.945, 0.987, and 0.996, respectively. These values for gas-to-hexadecane solvation enthalpy were 0.938, 0.985, and 0.994, respectively. The prediction result of the SVM model was superior to those obtained by the PLS and ANN methods, which showed that SVM was a practical tool in the prediction of the solvation enthalpy. This paper provides an original and effectual method for predicting DH Solv of organic compounds, and also discloses that SVM can be employed as an influential chemometrics tool for QSPR studies.
Application of QSPR for the prediction of gas to 1-octanol solvation enthalpy using support vector regression
Physics and Chemistry of Liquids, 2013
A quantitative structure property relationship model was developed to predict gas to 1-octanol so... more A quantitative structure property relationship model was developed to predict gas to 1-octanol solvation enthalpy (ΔHSolv) of 127 different organic compounds using support vector machine (SVM). The variable selection method of genetic algorithm (GA) was employed to select optimal subset of descriptors. The five descriptors selected by GA were used as inputs for construction of the multiple linear regression (MLR),

Molecular Informatics, 2012
In this study, a quantitative structureÀproperty relationship (QSPR) study is developed for the p... more In this study, a quantitative structureÀproperty relationship (QSPR) study is developed for the prediction of gas to dimethyl sulfoxide solvation enthalpy (DH Solv) of organic compounds based on molecular descriptors calculated solely from molecular structure considerations. Diverse types of molecular descriptors were calculated to represent the molecular structures of the various compounds studied. Multiple linear regression (MLR) was employed to select an optimal subset of descriptors that have significant contributions to the DH Solv overall property. Our investigation revealed that the dependence of physicochemical properties on solvation enthalpy is a nonlinear observable fact and that MLR method is unable to model the solvation enthalpy accurately. It has been observed that support vector machine (SVM) and artificial neural network (ANN) demonstrates better performance compared with MLR. The standard error value of the test set for SVM is 1.731 kJ mol À1 , while it is 2.303 kJ mol À1 and 5.146 kJ mol À1 for ANN and MLR, respectively. The results showed that the calculated DH Solv values by SVM were in good agreement with the experimental data, and the performance of the SVM model was superior to those of MLR and ANN ones.
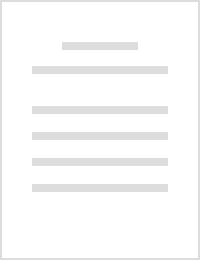
Prediction of Bovine Serum Albumin-Water Partition Coefficients of a Wide Variety of Neutral Organic Compounds by Means of Support Vector Machine
Molecular Informatics, 2012
Support vector machine (SVM) was used to develop a quantitative structure property relationship (... more Support vector machine (SVM) was used to develop a quantitative structure property relationship (QSPR) model that correlates molecular structures to their bovine serum albumin water partition coefficients (KBSA/W ). The performance and predictive aptitude of SVM are considered and compared with other methods such as multiple linear regression (MLR) and artificial neural network (ANN) methods. A set of 83 natural organic compounds and drugs were selected and suitable sets of molecular descriptors were calculated. Genetic algorithm (GA) was used to select important molecular descriptors, and linear and nonlinear models were applied to correlate the selected descriptors with the experimental values of log KBSA/W . The correlation coefficients, R, between experimental and predicted log KBSA/W for the validation set by MLR, ANN and SVM are 0.951, 0.986 and 0.991, respectively. Results obtained document the reliability and good predictability of the nonlinear QSPR model to predict partition coefficients of organic compounds. Comparison between the values of statistical parameters demonstrates that the predictive ability of the SVM model is comparable or superior to those obtained by MLR and ANN.

Microchemical Journal, 2013
In this study, a quantitative structure-property relationship (QSPR) method was employed to predi... more In this study, a quantitative structure-property relationship (QSPR) method was employed to predict the retention time (t R) of 368 pesticide residues in animal tissues separated by gas chromatography-mass spectroscopy (GC-MS). The variable selection method of genetic algorithm-partial least squares (GA-PLS) was employed to select most favorable subset of descriptors. The six descriptors selected using GA-PLS were used as inputs of PLS, ANN and SVM to predict the retention times. These descriptors are: number of nitrogen atoms, solvation connectivity index-Chi 1, Balaban Y index, Moran autocorrelation-lag 2/weighted by atomic Sanderson electronegativity, total absolute charge and radial distribution function-6.0/unweighted. The correlation coefficients, R, between experimental and predicted t R for the prediction set by PLS, ANN and SVM are 0.907, 0.963 and 0.985 respectively. Results obtained reveal the reliability and good predictability of nonlinear QSPR model to predict the retention time of pesticides. Comparison between the values of statistical parameters reveals the superiority of the SVM model over PLS and ANN ones.

Journal of Structural Chemistry, 2010
A Quantitative Structure-Property Relationship (QSPR) model based on Genetic Algorithm (GA), Mult... more A Quantitative Structure-Property Relationship (QSPR) model based on Genetic Algorithm (GA), Multiple Linear Regression (MLR) and Artificial Neural Network (ANN) techniques was developed for the prediction of water-to-polydimethylsiloxane partition coefficients (log K PDMS-water) of 139 organic compounds. A suitable set of molecular descriptors was calculated and important descriptors were selected by genetic algorithm and stepwise multiple regression. These descriptors were: Minimum Atomic Orbital Electronic Population (P PP), Kier Shape Index (order 3) (3 N), Polarity Parameter / Square Distance (PP), and Complementary Information Content (order 2) (2 CIC). In order to find a better way to depict the nonlinear nature of the relationships, these descriptors were used as inputs for a generated ANN. The root mean square errors for the neural network calculated log K PDMS-water of training, test, and validation sets were 0.116, 0.179, and 0.183, respectively, which are smaller than those obtained by MLR model (0.422, 0.425, and 0.480, respectively). The results obtained showed the ability of developed artificial neural network to predict water-to-polydimethylsiloxane partition coefficients of various organic compounds. Also, the results revealed the superiority of the artificial neural network over the multiple linear regression model.
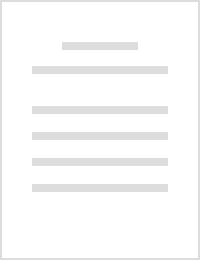
Prediction of Heat Capacities of Hydration of Various Organic Compounds Using Partial Least Squares and Artificial Neural Network
Journal of Solution Chemistry, 2013
ABSTRACT A quantitative structure–property relationship study based on artificial neural network ... more ABSTRACT A quantitative structure–property relationship study based on artificial neural network (ANN) was carried out for the prediction of the heat capacities of hydration of a set of 289 organic compounds of very different chemical natures. The genetic algorithm-partial least squares (GA-PLS) method was applied as a variable selection tool. A PLS method was used to select the best descriptors, and the selected descriptors were then used as input neurons in a neural network model. These descriptors are: number of H atoms (NHA), maximum partial charge in the molecule (Q max), atomic charge weighted PPSA (PPSA3), relative positive charge (RPCG), minimum net atomic charge (Q min), fractional PPSA (FPSA3), and Randic index (order 1) (1χ). The results obtained show the ability of the developed artificial neural network model to predict heat capacities of hydration of various organic compounds. Also, the results reveal the superiority of the ANN over the PLS model.

Journal of Molecular Liquids, 2014
A quantitative structure-property relationship (QSPR) model was developed to predict the gas-to-i... more A quantitative structure-property relationship (QSPR) model was developed to predict the gas-to-ionic liquid partition coefficient of a set of diverse organic solutes dissolved in 1-(2-hydroxyethyl)-1methylimidazoliumtris(pentafluoroethyl)trifluorophosphate ([EtOHMIm] + [FAP] −) at 323 K using the replacement method (RM) and support vector machine (SVM). Several types of molecular descriptors were calculated to represent the molecular structures of the different organic compounds studied. The replacement method (RM) was employed to select the optimal subset of descriptors that makes significant contributions to the gas-to-ionic liquid partition coefficient (K) overall property. Our study revealed that the dependence of physico-chemical properties on partition coefficient is a nonlinear and that RM method is incapable of modeling it precisely. The results obtained, exemplify that, the calculated log K values by SVM method were in good agreement with the experimental data, and the performance of the SVM model was superior to RM one.

Journal of Molecular Liquids, 2012
Quantitative structure-properties relationship (QSPR) has been applied to modelling and predictin... more Quantitative structure-properties relationship (QSPR) has been applied to modelling and predicting the gas to acetone and gas to acetonitrile solvation enthalpies (ΔH Solv) of organic compounds using partial least squares (PLS), artificial neural network (ANN) and support vector machine (SVM) techniques. Two different datasets were assessed. The first one contained a set of gas to acetone enthalpy of solvation data of 68 different organic compounds while the second one included a total of 69 experimental data points for the enthalpy of solvation in acetonitrile. Genetic algorithm (GA) was used to search the descriptor space and select the descriptors responsible for property. After the variable selection, PLS, ANN and SVM were utilized to construct linear and non-linear QSPR models. Our study demonstrates that the reliance of chemical properties on solvation enthalpies is a nonlinear phenomenon and that PLS method is not capable to model it. The results obtained, illustrate that, for both datasets, the calculated ΔH Solv values by SVM were in good agreement with the experimental ones, and the performances of the SVM models were comparable or superior to those of PLS and ANN ones.
Uploads
Papers by Zahra Dashtbozorgi