Papers by Suvodeep Majumder

Ethical bias in machine learning models has become a matter of concern in the software engineerin... more Ethical bias in machine learning models has become a matter of concern in the software engineering community. Most of the prior software engineering works concentrated on finding ethical bias in models rather than fixing it. After finding bias, the next step is mitigation. Prior researchers mainly tried to use supervised approaches to achieve fairness. However, in the real world, getting data with trustworthy ground truth is challenging and also ground truth can contain human bias. Semi-supervised learning is a machine learning technique where, incrementally, labeled data is used to generate pseudo-labels for the rest of the data (and then all that data is used for model training). In this work, we apply four popular semi-supervised techniques as pseudo-labelers to create fair classification models. Our framework, Fair-SSL, takes a very small amount (10%) of labeled data as input and generates pseudo-labels for the unlabeled data. We then synthetically generate new data points to ba...

ArXiv, 2021
Ethical bias in machine learning models has become a matter of concern in the software engineerin... more Ethical bias in machine learning models has become a matter of concern in the software engineering community. Most of the prior software engineering works concentrated on finding ethical bias in models rather than fixing it. After finding bias, the next step is mitigation. Prior researchers mainly tried to use supervised approaches to achieve fairness. However, in the real world, getting data with trustworthy ground truth is challenging and also ground truth can contain human bias. Semi-supervised learning is a machine learning technique where, incrementally, labelled data is used to generate pseudo-labels for the rest of data (and then all that data is used for model training). In this work, we apply four popular semi-supervised techniques as pseudo-labelers to create fair classification models. Our framework, Fair-SSL, takes a very small amount (10%) of labeled data as input and generates pseudo-labels for the unlabeled data. We then synthetically generate new data points to balan...

A "hero" project is one where 80% or more of the contributions are made by the 20% of t... more A "hero" project is one where 80% or more of the contributions are made by the 20% of the developers. Those developers are called "hero" developers. In the literature, heroes projects are deprecated since they might cause bottlenecks in development and communication. However, there is little empirical evidence on this matter. Further, recent studies show that such hero projects are very prevalent. Accordingly, this paper explores the effect of having heroes in project, from a code quality perspective by analyzing 1000+ open source GitHub projects. Based on the analysis, this study finds that (a) majority of the projects are hero projects; and (b)the commits from "hero developers" (who contribute most to the code) result in far fewer bugs than other developers. That is, contrary to the literature, heroes are standard and very useful part of modern open source projects.

Deep learning methods are useful for high-dimensional data and are becoming widely used in many a... more Deep learning methods are useful for high-dimensional data and are becoming widely used in many areas of software engineering. Deep learners utilizes extensive computational power and can take a long time to train-- making it difficult to widely validate and repeat and improve their results. Further, they are not the best solution in all domains. For example, recent results show that for finding related Stack Overflow posts, a tuned SVM performs similarly to a deep learner, but is significantly faster to train. This paper extends that recent result by clustering the dataset, then tuning very learners within each cluster. This approach is over 500 times faster than deep learning (and over 900 times faster if we use all the cores on a standard laptop computer). Significantly, this faster approach generates classifiers nearly as good (within 2\% F1 Score) as the much slower deep learning method. Hence we recommend this faster methods since it is much easier to reproduce and utilizes fa...
Communication and Code Dependency Effects on Software Code Quality (an Empirical Analysis of Herbsleb Hypothesis)
SSRN Electronic Journal

ArXiv, 2020
Machine learning software is increasingly being used to make decisions that affect people’s lives... more Machine learning software is increasingly being used to make decisions that affect people’s lives. But sometimes, the core part of this software (the learned model), behaves in a biased manner that gives undue advantages to a specific group of people (where those groups are determined by sex, race, etc.). This “algorithmic discrimination” in the AI software systems has become a matter of serious concern in the machine learning and software engineering community. There have been works done to find “algorithmic bias” or “ethical bias” in software system. Once the bias is detected in the AI software system, mitigation of bias is extremely important. In this work, we a) explain how ground truth bias in training data affects machine learning model fairness and how to find that bias in AI software, b) propose a method Fairway which combines preprocessing and in-processing approach to remove ethical bias from training data and trained model. Our results show that we can find bias and mitig...
CONTEXT: There has been a rapid growth in the use of data analytics to underpin evidence-based so... more CONTEXT: There has been a rapid growth in the use of data analytics to underpin evidence-based software engineering. However the combination of complex techniques, diverse reporting standards and complex underlying phenomena are causing some concern as to the reliability of studies. OBJECTIVE: Our goal is to provide guidance for producers and consumers of software analytics studies (computational experiments and correlation studies). METHOD: We propose using “bad smells", i.e. surface indications of deeper problems and popular in the agile software community and consider how they may be manifest in software analytics studies. RESULTS: We provide a list of 11 “bad smells" in decreasing order of severity and show their impact by examples. CONCLUSIONS: We should encourage more debate on what constitutes a ‘valid’ study (so we expect our list will mature over time).

Testing machine learning software for ethical bias has become a pressing current concern. In resp... more Testing machine learning software for ethical bias has become a pressing current concern. In response, recent research has proposed a plethora of new fairness metrics, for example, the dozens of fairness metrics in the IBM AIF360 toolkit. This raises the question: How can any fairness tool satisfy such a diverse range of goals? While we cannot completely simplify the task of fairness testing, we can certainly reduce the problem. This paper shows that many of those fairness metrics effectively measure the same thing. Based on experiments using seven real-world datasets, we find that (a) 26 classification metrics can be clustered into seven groups, and (b) four dataset metrics can be clustered into three groups. Further, each reduced set may actually predict different things. Hence, it is no longer necessary (or even possible) to satisfy all fairness metrics. In summary, to simplify the fairness testing problem, we recommend the following steps: (1) determine what type of fairness is ...

Managers and practitioners become dubious about software analytics when its conclusions keep chan... more Managers and practitioners become dubious about software analytics when its conclusions keep changing as we look at new projects. GENERAL is a new approach for quickly finding conclusions that generalize across hundreds of projects. This algorithm (a) removes spurious attributes via feature selection; (b) fixes training data imbalance via synthetic instances; (c) recursively clusters the project data; (d) finds the best model within any cluster, then promotes it up the cluster tree; (e) returns the model promoted to the top. GENERAL is much faster than prior methods (4.8 hours versus 204 hours our case studies) and theoretically scales better (O(N^2/m) versus O(N^2), which is a large reduction since often we find m>20 clusters). When tested on 756 Github projects, a single defect prediction model generalized over all those projects while also being useful and insightful and generalizable; i.e. that model worked just as well as 756 separate models learned from each project; and th...
ArXiv, 2019
A "hero" project is one where 80% or more of the contributions are made by the 20% of t... more A "hero" project is one where 80% or more of the contributions are made by the 20% of the developers. In the literature, such projects are deprecated since they might cause bottlenecks in development and communication. However, there is little empirical evidence on this matter. Further, recent studies show that such hero projects are very prevalent. Accordingly, this paper explores the effect of having heroes in project, from a code quality perspective. We identify the heroes developer communities in 1100+ open source GitHub projects. Based on the analysis, we find that (a) hero projects are majorly all projects; and (b) the commits from "hero developers" (who contribute most to the code) result in far fewer bugs than other developers. That is, contrary to the literature, heroes are standard and very useful part of modern open source projects.

Despite decades of research, SE lacks widely accepted models (that offer precise quantitative pre... more Despite decades of research, SE lacks widely accepted models (that offer precise quantitative predictions) about what factors most influence software quality. This paper provides a “good news” result that such general models can be generated using a new transfer learning framework called “GENERAL”. Given a tree of recursively clustered projects (using project meta-data), GENERAL promotes a model upwards if it performs best in the lower clusters (stopping when the promoted model performs worse than the models seen at a lower level). The number of models found by GENERAL is minimal: one for defect prediction (756 projects) and less than a dozen for project health (1628 projects). Hence, via GENERAL, it is possible to make conclusions that hold across hundreds of projects at a time. Further, the models produced in this manner offer predictions that perform as well or better than prior state-of-the-art. To the best of our knowledge, this is the largest demonstration of the generalizabil...

Proceedings of the 29th ACM Joint Meeting on European Software Engineering Conference and Symposium on the Foundations of Software Engineering
Increasingly, software is making autonomous decisions in case of criminal sentencing, approving c... more Increasingly, software is making autonomous decisions in case of criminal sentencing, approving credit cards, hiring employees, and so on. Some of these decisions show bias and adversely affect certain social groups (e.g. those defined by sex, race, age, marital status). Many prior works on bias mitigation take the following form: change the data or learners in multiple ways, then see if any of that improves fairness. Perhaps a better approach is to postulate root causes of bias and then applying some resolution strategy. This paper checks if the root causes of bias are the prior decisions about (a) what data was selected and (b) the labels assigned to those examples. Our Fair-SMOTE algorithm removes biased labels; and rebalances internal distributions so that, based on sensitive attribute, examples are equal in positive and negative classes. On testing, this method was just as effective at reducing bias as prior approaches. Further, models generated via Fair-SMOTE achieve higher performance (measured in terms of recall and F1) than other state-of-the-art fairness improvement algorithms. To the best of our knowledge, measured in terms of number of analyzed learners and datasets, this study is one of the largest studies on bias mitigation yet presented in the literature. • Software and its engineering → Software creation and management; • Computing methodologies → Machine learning.
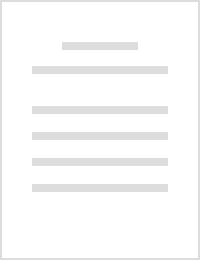
Reliability and NDT Methods
Advanced Structured Materials
Composites are finding increased use in structural high demanding and high added value applicatio... more Composites are finding increased use in structural high demanding and high added value applications in advanced industries. A wide diversity exists in terms of matrix type, which can be either polymeric or metallic and type of reinforcements (ceramic, polymeric or metallic). Several technologies have been used to produce these composites; among them, additive manufacturing (AM) is currently being applied. In structural applications, the presence of defects due to fabrication is of major concern, since it affects the performance of a component with negative impact, which can affect, ultimately, human lives. Thus, the detection of defects is highly important, not only surface defects but also barely visible defects. This chapter describes the main types of defects expected in composites produced by AM. The fundamentals of different non-destructive testing (NDT) techniques are briefly discussed, as well as the state of the art of numerical simulation for several NDT techniques. A multiparametric and customized inspection system was developed based on the combination of innovative techniques in modelling and testing. Experimental validation with eddy currents, ultrasounds, X-ray and thermography is presented and analysed, as well as integration of distinctive techniques and 3D scanning characterization.

2021 IEEE/ACM 43rd International Conference on Software Engineering (ICSE)
Many methods in defect prediction are "datahungry"; i.e. (1) given a choice of using more data, o... more Many methods in defect prediction are "datahungry"; i.e. (1) given a choice of using more data, or some smaller sample, researchers assume that more is better; (2) when data is missing, researchers take elaborate steps to transfer data from another project; and (3) given a choice of older data or some more recent sample, researchers usually ignore older data. Based on the analysis of hundreds of popular Github projects (with 1.2 million commits), we suggest that for defect prediction, there is limited value in such data-hungry approaches. Data for our sample of projects last for 84 months and contains 3,728 commits (median values). Across these projects, most of the defects occur very early in their life cycle. Hence, defect predictors learned from the first 150 commits and four months perform just as well as anything else. This means that, contrary to the "data-hungry" approach, (1) small samples of data from these projects are all that is needed for defect prediction; (2) transfer learning has limited value since it is needed only for the first 4 of 84 months (i.e. just 4% of the life cycle); (3) after the first few months, we need not continually update our defect prediction models. We hope these results inspire other researchers to adopt a 'simplicity-first" approach to their work. Certainly, there are domains that require a complex and data-hungry analysis. But before assuming complexity, it is prudent to check the raw data looking for "short cuts" that simplify the whole analysis.

Proceedings of the 28th ACM Joint Meeting on European Software Engineering Conference and Symposium on the Foundations of Software Engineering
Machine learning software is increasingly being used to make decisions that affect people's lives... more Machine learning software is increasingly being used to make decisions that affect people's lives. But sometimes, the core part of this software (the learned model), behaves in a biased manner that gives undue advantages to a specific group of people (where those groups are determined by sex, race, etc.). This "algorithmic discrimination" in the AI software systems has become a matter of serious concern in the machine learning and software engineering community. There have been works done to find "algorithmic bias" or "ethical bias" in software system. Once the bias is detected in the AI software system, mitigation of bias is extremely important. In this work, we a) explain how ground truth bias in training data affects machine learning model fairness and how to find that bias in AI software, b) propose a method Fairway which combines preprocessing and in-processing approach to remove ethical bias from training data and trained model. Our results show that we can find bias and mitigate bias in a learned model, without much damaging the predictive performance of that model. We propose that (1) testing for bias and (2) bias mitigation should be a routine part of the machine learning software development life cycle. Fairway offers much support for these two purposes.

Proceedings of the 15th International Conference on Mining Software Repositories
Deep learning methods are useful for high-dimensional data and are becoming widely used in many a... more Deep learning methods are useful for high-dimensional data and are becoming widely used in many areas of so ware engineering. Deep learners utilizes extensive computational power and can take a long time to train-making it di cult to widely validate and repeat and improve their results. Further, they are not the best solution in all domains. For example, recent results show that for nding related Stack Over ow posts, a tuned SVM performs similarly to a deep learner, but is signi cantly faster to train. is paper extends that recent result by clustering the dataset, then tuning very learners within each cluster. is approach is over 500 times faster than deep learning (and over 900 times faster if we use all the cores on a standard laptop computer). Signi cantly, this faster approach generates classi ers nearly as good (within 2% F1 Score) as the much slower deep learning method. Hence we recommend this faster methods since it is much easier to reproduce and utilizes far fewer CPU resources. More generally, we recommend that before researchers release research results, that they compare their supposedly sophisticated methods against simpler alternatives (e.g applying simpler learners to build local models).

Information and Software Technology
CONTEXT: There has been a rapid growth in the use of data analytics to underpin evidence-based so... more CONTEXT: There has been a rapid growth in the use of data analytics to underpin evidence-based software engineering. However the combination of complex techniques, diverse reporting standards and poorly understood underlying phenomena are causing some concern as to the reliability of studies. OBJECTIVE: Our goal is to provide guidance for producers and consumers of software analytics studies (computational experiments and correlation studies). METHOD: We propose using "bad smells", i.e., surface indications of deeper problems and popular in the agile software community and consider how they may be manifest in software analytics studies. RESULTS: We list 12 "bad smells" in software analytics papers (and show their impact by examples). CONCLUSIONS: We believe the metaphor of bad smell is a useful device. Therefore we encourage more debate on what contributes to the validty of software analytics studies (so we expect our list will mature over time).

Empirical Software Engineering
Numerous methods can build predictive models from software data. However, what methods and conclu... more Numerous methods can build predictive models from software data. However, what methods and conclusions should we endorse as we move from analytics in-the-small (dealing with a handful of projects) to analytics in-thelarge (dealing with hundreds of projects)? To answer this question, we recheck prior small-scale results (about process versus product metrics for defect prediction and the granularity of metrics) using 722,471 commits from 700 Github projects. We find that some analytics in-the-small conclusions still hold when scaling up to analytics in-the-large. For example, like prior work, we see that process metrics are better predictors for defects than product metrics (best process/product-based learners respectively achieve recalls of 98%/44% and AUCs of 95%/54%, median values). That said, we warn that it is unwise to trust metric importance results from analytics in-the-small studies since those change dramatically when moving to analytics in-the-large. Also, when reasoning in-the-large about hundreds of projects, it is better to use predictions from multiple models (since single model predictions can become confused and exhibit a high variance).
Uploads
Papers by Suvodeep Majumder