Papers by Sirawaj Itthipuripat
Within-participant differences in attention-related shifts in contrast response functions measured using EEG and fMRI
Journal of Vision, 2014

The Journal of Neuroscience, 2018
Decision-making becomes slower when more choices are available. Existing models attribute this sl... more Decision-making becomes slower when more choices are available. Existing models attribute this slowing to poor sensory processing, to attenuated rates of sensory evidence accumulation, or to increases in the amount of evidence required before committing to a decision (a higher decision threshold). However, studies have not isolated the effects of having more choices on sensory and decision-related processes from changes in task difficulty and divided attention. Here, we controlled task difficulty while independently manipulating the distribution of attention and the number of choices available to male and female human observers. We used EEG to measure steady-state visually evoked potentials (SSVEPs) and a frontal late positive deflection (LPD), EEG markers of sensory and postsensory decision-related processes, respectively. We found that dividing attention decreased SSVEP and LPD amplitudes, consistent with dampened sensory responses and slower rates of evidence accumulation, respec...

ABSTRACTMild cognitive impairment (MCI) is an early stage of age-inappropriate cognitive decline,... more ABSTRACTMild cognitive impairment (MCI) is an early stage of age-inappropriate cognitive decline, which could develop into dementia – an untreatable neurodegenerative disorder. An early detection of MCI is a crucial step for timely prevention and intervention. To tackle this problem, recent studies have developed deep learning models to detect MCI and various types of dementia using data obtained from the classic clock-drawing test (CDT), a popular neuropsychological screening tool that can be easily and rapidly implemented for assessing cognitive impairments in an aging population. While these models succeed at distinguishing severe forms of dementia, it is still difficult to predict the early stage of the disease using the CDT data alone. Also, the state-of-the-art deep learning techniques still face the black-box challenges, making it questionable to implement them in the clinical setting. Here, we propose a novel deep learning modeling framework that incorporates data from multi...
Corresponding modeling results during the early training phase in Fig 8
Linking changes in the psychophysical data and the P1 data using the efficient readout model
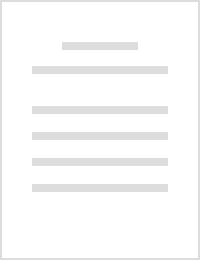
Modulations of the P1 component without baseline subtraction
<p>(A) The P1 contrast response functions (CRFs) based on event-related potentials (ERPs) w... more <p>(A) The P1 contrast response functions (CRFs) based on event-related potentials (ERPs) without the baseline subtraction. Note that the contrast values on the x-axis are not exactly the same across target and nontarget conditions because in the target conditions we used the averaged contrast values between the pedestal and incremental stimuli. (B) The P1 response amplitude obtained from 0% pedestal contrast stimuli (i.e., baseline activity) across training days (2 electroencephalography [EEG] sessions or ~1 day per time bin). Error bars in (A) and (B) represent within-subject SEM. There was no main effect of attention, no main effect of training, or no interaction between the 2 factors on the P1 baseline activity. (C) Corresponding maximum response (response at 100% contrast minus baseline) and half-maximum contrast for the P1-based CRFs in (A). Overall, the results are consistent with the P1 results with baseline subtraction (<a href="http://www.plosbiology.org/article/info:doi/10.1371/journal.pbio.2001724#pbio.2001724.g005" target="_blank">Fig 5</a>), in which a gain modulation of the maximum response was observed during the early training phase, but this attentional gain modulation disappeared after training. The main difference between this result and the baseline-subtracted result is that the baselines of the P1 CRFs across all experimental conditions were shifted down and were negative. This was due to an early negative component induced by the stimulus that was ipsilateral to the electrode of interest. Error bars represent the 68% CIs; * and *** represent significant pairwise comparisons between attention conditions in each training phase with <i>p</i> < 0.05 and <i>p</i> < 0.001, respectively. <sup>x</sup> represents a significant interaction between training (early and late) and attention (focused target and divided attention) with <i>p</i> < 0.05. (D) The day-by-day analysis of the P1 data without baseline subtraction (2 EEG sessions or ~1 day per each time bin). Left and right panels represent the estimated maximum response and half-maximum contrast parameters. Error bars represent the 68% CIs. Blue *, **, and *** in (D) represent significant pairwise comparisons between the maximum response on the second day, on which the behavioral performance started to reach a saturation level (<a href="http://www.plosbiology.org/article/info:doi/10.1371/journal.pbio.2001724#pbio.2001724.g002" target="_blank">Fig 2C</a>), and the maximum response on other time bins, with <i>p</i> < 0.05, <i>p</i> < 0.01, and <i>p</i> < 0.001 (false discovery rate [FDR]-corrected). Data are available from the Open Science Framework (<a href="https://osf.io/pc7dr/" target="_blank">https://osf.io/pc7dr/</a>). n.s., not significant.</p
Event-related potential (ERP) results
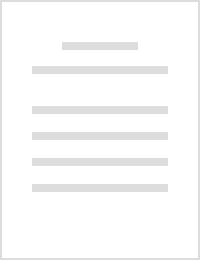
Modulations of the P1 component with baseline subtraction
<p>The contrast response functions (CRFs), based on the amplitude of the extracted P1 compo... more <p>The contrast response functions (CRFs), based on the amplitude of the extracted P1 component, which was averaged over the contralateral posterior–occipital electrodes from 80–130 ms poststimulus. During the early training phase, there was a robust attentional gain amplification of the P1 component on focused attention trials compared to divided attention trials (left panel). However, no attention-related gain modulations were present during the late training phase (right panel). Error bars represent within-subject SEM. (B) Corresponding maximum response (response at 100% contrast minus baseline) and half-maximum contrast for the P1-based CRFs in (A). Error bars represent the 68% CIs; ** and *** represent significant pairwise comparisons between attention conditions in each training phase with <i>p</i> < 0.01 and <i>p</i> < 0.001, respectively. Note that in this and subsequent figures, we use 68% CIs for data that were analyzed via nonparametric bootstrapping methods (e.g., the fit parameters) and within-subject SEM for data that were analyzed using standard parametric approaches. <sup>xx</sup> represents a significant interaction between training (early and late) and attention (focused target and divided attention), with <i>p</i> < 0.01. (C) The P1-based CRFs evoked by the divided target and divided nontarget stimuli during the first and second stimulus intervals across the early and late training phases. There was no significant change in the P1-based CRFs across these stimulus conditions and training phases. This justified averaging between the P1 data elicited by the divided target and divided nontarget stimuli from both stimulus intervals. Error bars represent within-subject SEM. (D) Corresponding maximum response and half-maximum contrast for the P1-based CRFs in (C). Error bars represent the 68% CIs. (E) The day-by-day analysis of the P1 data with baseline subtraction (2 electroencephalography [EEG] sessions or ~1 day per each time bin). Left and right panels represent the estimated maximum response and half-maximum contrast parameters. Error bars represent the 68% CIs. Blue *, **, and *** in (E) represent significant pairwise comparisons between the maximum response on the second time bin in which the behavioral performance started to reach a saturation level (<a href="http://www.plosbiology.org/article/info:doi/10.1371/journal.pbio.2001724#pbio.2001724.g002" target="_blank">Fig 2C</a>) and the maximum response in other time bins with <i>p</i> < 0.05, <i>p</i> < 0.01, and <i>p</i> < 0.001 (false discovery rate [FDR]-corrected). Note that the contrast values on the x-axis in (A) and (C) are not exactly the same across target and nontarget conditions because in the target conditions, we used the averaged contrast values of the pedestal and incremental stimuli. Data are available from the Open Science Framework (<a href="https://osf.io/pc7dr/" target="_blank">https://osf.io/pc7dr/</a>). n.s., not significant.</p
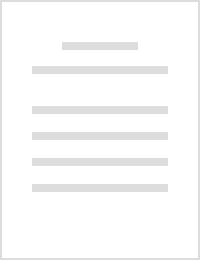
Modulations of the late positive deflection (LPD) component with baseline subtraction
<p>(A) The contrast response function (CRF), based on the amplitude of the LPD component, w... more <p>(A) The contrast response function (CRF), based on the amplitude of the LPD component, which was averaged over posterior electrodes from 230–380 ms poststimulus. Focused attention increased the LPD amplitude by a comparable amount across early and late training phases. Error bars represent within-subject SEM. (B) Corresponding maximum response (response at 100% contrast minus baseline) and half-maximum contrast parameters of the CRFs shown in (A). Error bars represent the 68% CIs; *, **, and *** represent significant pairwise comparisons between attention conditions in each training phase, with <i>p</i> <0.05, <i>p</i> < 0.01, and <i>p</i> < 0.001, respectively. (C) The LPD-based CRFs evoked by the divided target nontarget stimuli during the first and second stimulus intervals across the early and late training phases. We observed a separation between the divided target and nontarget responses only during the second stimulus interval of the late training phase, consistent with the idea that the LPD reflects postperceptual decision-making processes that can be enhanced with training [<a href="http://www.plosbiology.org/article/info:doi/10.1371/journal.pbio.2001724#pbio.2001724.ref067" target="_blank">67</a>–<a href="http://www.plosbiology.org/article/info:doi/10.1371/journal.pbio.2001724#pbio.2001724.ref068" target="_blank">68</a>]. Error bars represent within-subject SEM. (D) Corresponding maximum response and half-maximum contrast parameters of the CRFs shown in (C). Error bars represent the 68% CIs; **, and *** represent significant pairwise comparisons between attention conditions in each training phase with <i>p</i> < 0.01 and <i>p</i> < 0.001, respectively. (E) The day-by-day analysis of the LPD data with baseline subtraction (2 electroencephalography [EEG] sessions or ~1 day per each time bin). Top and bottom panels represent the estimated maximum response and half-maximum contrast parameters. (F) The day-by-day analysis of the LPD data shown in (C) and (D). Error bars in (E) and (F) represent the 68% CIs. Note that the contrast values on the x-axis in (A) and (C) are not exactly the same across target and nontarget conditions because, in the target conditions, we used the averaged contrast values between the pedestal and incremental stimuli. Data are available from the Open Science Framework (<a href="https://osf.io/pc7dr/" target="_blank">https://osf.io/pc7dr/</a>).</p
Dissociable neural mechanisms underlie effects of attention on visual appearance and response bias
Journal of Vision, 2020
Expectations about low-level visual features influence late stages of cortical information processing
Journal of Vision, 2018
Cortex, 2020
electrical stimulation was found on VSTM precision. Together, these results suggest that encoding... more electrical stimulation was found on VSTM precision. Together, these results suggest that encoding strategy has a selective influence on VSTM capacity and this influence of encoding strategy mediates the effect of electrical stimulation over PPC on VSTM function.
The Journal of Neuroscience, 2019
SI and TCS conceived, implemented the experiments, collected and analyzed the data, and co-wrote ... more SI and TCS conceived, implemented the experiments, collected and analyzed the data, and co-wrote the manuscript. JTS conceived and supervised the project and co-wrote the manuscript.

Decisions made about identical perceptual stimuli can be radically different under changing task ... more Decisions made about identical perceptual stimuli can be radically different under changing task demands. For example, the need to make a fast decision undermines the accuracy of that decision, a well-documented effect termed the speed-accuracy tradeoff (SAT). Models of the SAT are generally based on theories of decision making in which responses are triggered only after sensory evidence accumulation terminates at a set threshold. Within this accumulate-to-bound framework, speed pressure operates by lowering the response threshold, allowing for faster responses at the expense of accumulated sensory evidence. To empirically examine the mechanisms necessary for adaptively adjusting the speed and accuracy of decisions, we used an event-related potential that indexes sensory evidence accumulation in the human brain. Instead of lowering response thresholds, we found that speed pressure adaptively shifts responses to occur close to where the rate of evidence accumulation peaks. Moreover, ...

Functional magnetic resonance imaging (fMRI) and electroencephalography (EEG) are the two most po... more Functional magnetic resonance imaging (fMRI) and electroencephalography (EEG) are the two most popular non-invasive methods used to study the neural mechanisms underlying human cognition. These approaches are considered complementary: fMRI has higher spatial resolution but sluggish temporal resolution, whereas EEG has millisecond temporal resolution, but only at a broad spatial scale. Beyond the obvious fact that fMRI measures properties of blood and EEG measures changes in electric fields, many foundational studies assume that, aside from differences in spatial and temporal precision, these two methods index the same underlying neural modulations. We tested this assumption by using EEG and fMRI to measure attentional modulations of neural responses to stimuli of different visual contrasts. We found that equivalent experiments performed using fMRI and EEG on the same participants revealed remarkably different patterns of attentional modulations: event-related fMRI responses provided...

Nature Communications, 2017
Rhythmic neural activity in the alpha band (8-13 Hz) is thought to have an important role in the ... more Rhythmic neural activity in the alpha band (8-13 Hz) is thought to have an important role in the selective processing of visual information. Typically, modulations in alpha amplitude and instantaneous frequency are thought to reflect independent mechanisms impacting dissociable aspects of visual information processing. However, in complex systems with interacting oscillators such as the brain, amplitude and frequency are mathematically dependent. Here, we record electroencephalography in human subjects and show that both alpha amplitude and instantaneous frequency predict behavioral performance in the same visual discrimination task. Consistent with a model of coupled oscillators, we show that fluctuations in instantaneous frequency predict alpha amplitude on a single trial basis, empirically demonstrating that these metrics are not independent. This interdependence suggests that changes in amplitude and instantaneous frequency reflect a common change in the excitatory and inhibitory neural activity that regulates alpha oscillations and visual information processing.

The Journal of Neuroscience, 2018
Visual short-term memory (VSTM) provides an on-line mental space for incoming sensory information... more Visual short-term memory (VSTM) provides an on-line mental space for incoming sensory information to be temporally maintained to carry out complex behavioral tasks. Despite its essential functions, the capacity at which VSTM could maintain sensory information is limited (i.e., VSTM can hold only about three to four visual items at once). Moreover, the quality of sensory representation (i.e., precision) degrades as more information has to be maintained in VSTM. Correlational evidence suggests that the level and the pattern of neural activity measured in the posterior parietal cortex (PPC) track both VSTM capacity and precision. However, the causal contributions of the PPC to these different VSTM operations are unclear. Here, we tested whether stimulating the PPC with transcranial direct current stimulation (tDCS) could increase VSTM capacity or precision. We found that stimulating the PPC in male and female human participants selectively enhanced VSTM capacity when the number of memory items exceeded capacity limit, without significant effects on VSTM precision. Moreover, this enhancement of VSTM capacity is region specific as stimulating the prefrontal cortex did not change VSTM capacity or precision. Null stimulation effects in the sensory memory condition confirmed that the tDCS-induced enhancement of VSTM capacity was not simply due to changes in sensory or attentional processes. Altogether, these results provide causal evidence suggesting that the PPC has a more dominant role in supporting the storage capacity of VSTM compared with maintaining the quality of sensory representations. Furthermore, tDCS could be used as a promising noninvasive method to enhance this PPC VSTM-related function.

Journal of neurophysiology, Jan 28, 2018
Computational models posit that visual attention is guided by activity within spatial maps that i... more Computational models posit that visual attention is guided by activity within spatial maps that index the image-computable salience and the behavioral relevance of objects in the scene. These spatial maps are theorized to be instantiated as activation patterns across a series of retinotopic visual regions in occipital, parietal, and frontal cortex. While previous research has identified sensitivity to either the behavioral relevance or the image-computable salience of different scene elements, the simultaneous influence of these factors on neural 'attentional priority maps' in human cortex is not well understood. We tested the hypothesis that visual salience and behavioral relevance independently impact the activation profile across retinotopically-organized cortical regions by quantifying attentional priority maps measured in human brains using functional MRI while participants attended one of two differentially-salient stimuli. We found that the topography of activation in...

PLOS Biology, 2017
Selective attention supports the prioritized processing of relevant sensory information to facili... more Selective attention supports the prioritized processing of relevant sensory information to facilitate goal-directed behavior. Studies in human subjects demonstrate that attentional gain of cortical responses can sufficiently account for attention-related improvements in behavior. On the other hand, studies using highly trained nonhuman primates suggest that reductions in neural noise can better explain attentional facilitation of behavior. Given the importance of selective information processing in nearly all domains of cognition, we sought to reconcile these competing accounts by testing the hypothesis that extensive behavioral training alters the neural mechanisms that support selective attention. We tested this hypothesis using electroencephalography (EEG) to measure stimulus-evoked visual responses from human subjects while they performed a selective spatial attention task over the course of~1 month. Early in training, spatial attention led to an increase in the gain of stimulusevoked visual responses. Gain was apparent within~100 ms of stimulus onset, and a quantitative model based on signal detection theory (SDT) successfully linked the magnitude of this gain modulation to attention-related improvements in behavior. However, after extensive training, this early attentional gain was eliminated even though there were still substantial attention-related improvements in behavior. Accordingly, the SDT-based model required noise reduction to account for the link between the stimulus-evoked visual responses and attentional modulations of behavior. These findings suggest that training can lead to fundamental changes in the way attention alters the early cortical responses that support selective information processing. Moreover, these data facilitate the translation of results across different species and across experimental procedures that employ different behavioral training regimes.

Attention supports the selection of relevant sensory information from competing irrelevant sensor... more Attention supports the selection of relevant sensory information from competing irrelevant sensory information. This selective processing is thought to be supported via the attentional gain amplification of sensory responses evoked by attended compared to unattended stimuli. However, recent studies in highly trained subjects suggest that attentional gain plays a relatively modest role and that other types of neural modulations, such as a reduction in neural noise, better explain attention-related changes in behavior. We hypothesized that the amount of training may alter neural mechanisms that support attentional selection in visual cortex. To test this hypothesis, we investigated the influence of training on attentional modulations of stimulus-evoked visual responses by recording electroencephalography (EEG) from humans performing a selective visuospatial attention task over the course of one month. Early in training, visuospatial attention induced a robust attentional gain amplific...
Uploads
Papers by Sirawaj Itthipuripat