Papers by Shinta Sintieya Poetri
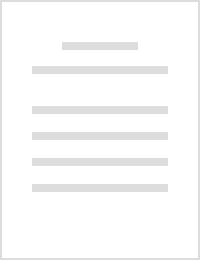
Face recognition/detection by probabilistic decision-based neural network
IEEE Transactions on Neural Networks, 1997
This paper proposes a face recognition system, based on probabilistic decision-based neural netwo... more This paper proposes a face recognition system, based on probabilistic decision-based neural networks (PDBNN). With technological advance on microelectronic and vision system, high performance automatic techniques on biometric recognition are now becoming economically feasible. Among all the biometric identification methods, face recognition has attracted much attention in recent years because it has potential to be most nonintrusive and user-friendly. The PDBNN face recognition system consists of three modules: First, a face detector finds the location of a human face in an image. Then an eye localizer determines the positions of both eyes in order to generate meaningful feature vectors. The facial region proposed contains eyebrows, eyes, and nose, but excluding mouth (eye-glasses will be allowed). Lastly, the third module is a face recognizer. The PDBNN can be effectively applied to all the three modules. It adopts a hierarchical network structures with nonlinear basis functions and a competitive credit-assignment scheme. The paper demonstrates a successful application of PDBNN to face recognition applications on two public (FERET and ORL) and one in-house (SCR) databases. Regarding the performance, experimental results on three different databases such as recognition accuracies as well as false rejection and false acceptance rates are elaborated. As to the processing speed, the whole recognition process (including PDBNN processing for eye localization, feature extraction, and classification) consumes approximately one second on Sparc10, without using hardware accelerator or co-processor.
IEEE Transactions on Pattern Analysis and Machine Intelligence, 2002
Human face detection is often the first step in applications such as video surveill:ance, human c... more Human face detection is often the first step in applications such as video surveill:ance, human computer interface, face recognition, and image database management.
Human face detection in a complex background
Pattern Recognition, 1994
The human face is a complex pattern. Finding human faces automatically in a scene is a difficult ... more The human face is a complex pattern. Finding human faces automatically in a scene is a difficult yet significant problem. It is the first important step in a fully automatic human face recognition system. In this paper a new method to locate human faces in a complex background is proposed. This system utilizes a hierarchical knowledge-based method and consists of three levels. The higher two levels are based on mosaic images at different resolutions. In the lower level, an improved edge detection method is proposed. In this research the problem of scale is dealt with, so that the system can locate unknown human faces spanning a wide range of sizes in a complex black-and-white picture. Some experimental results are given.
We present a neural network-based face detection system. A retinally connected neural network exa... more We present a neural network-based face detection system. A retinally connected neural network examines small windows of an image, and decides whether each window contains a face. The system arbitrates between multiple networks to improve performance over a single network. We use a bootstrap algorithm for training, which adds false detections into the training set as training progresses. This eliminates the difficult task of manually selecting non-face training examples, which must be chosen to span the entire space of non-face images. Comparisons with another state-of-the-art face detection system are presented; our system has better performance in terms of detection and false-positive rates.

International Journal of Computer Vision, 2001
This paper describes a face detection framework that is capable of processing images extremely ra... more This paper describes a face detection framework that is capable of processing images extremely rapidly while achieving high detection rates. There are three key contributions. The first is the introduction of a new image representation called the “Integral Image” which allows the features used by our detector to be computed very quickly. The second is a simple and efficient classifier which is built using the AdaBoost learning algorithm (Freund and Schapire, 1995) to select a small number of critical visual features from a very large set of potential features. The third contribution is a method for combining classifiers in a “cascade” which allows background regions of the image to be quickly discarded while spending more computation on promising face-like regions. A set of experiments in the domain of face detection is presented. The system yields face detection performance comparable to the best previous systems (Sung and Poggio, 1998; Rowley et al., 1998; Schneiderman and Kanade, 2000; Roth et al., 2000). Implemented on a conventional desktop, face detection proceeds at 15 frames per second.

International Journal of Computer Vision, 2001
This paper describes a visual object detection framework that is capable of processing images ext... more This paper describes a visual object detection framework that is capable of processing images extremely rapidly while achieving high detection rates. There are three key contributions. The first is the introduction of a new image representation called the "Integral Image" which allows the features used by our detector to be computed very quickly. The second is a learning algorithm, based on AdaBoost, which selects a small number of critical visual features and yields extremely efficient classifiers . The third contribution is a method for combining classifiers in a "cascade" which allows background regions of the image to be quickly discarded while spending more computation on promising object-like regions. A set of experiments in the domain of face detection are presented. The system yields face detection performace comparable to the best previous systems . Implemented on a conventional desktop, face detection proceeds at 15 frames per second.

We investigate the application of Support Vector Machines (SVMs) in computer vision. SVM is a lea... more We investigate the application of Support Vector Machines (SVMs) in computer vision. SVM is a learning technique developed by V. Vapnik and his team (AT&T Bell Labs., 1985) that can be seen as a new method for training polynomial, neural network, or Radial Basis Functions classifiers. The decision surfaces are found by solving a linearly constrained quadratic programming problem. This optimization problem is challenging because the quadratic form is completely dense and the memory requirements grow with the square of the number of data points. We present a decomposition algorithm that guarantees global optimality, and can be used to train SVM's over very large data sets. The main idea behind the decomposition is the iterative solution of sub-problems and the evaluation of optimality conditions which are used both to generate improved iterative values, and also establish the stopping criteria for the algorithm. We present experimental results of our implementation of SVM, and demonstrate the feasibility of our approach on a face detection problem that involves a data set of 50,000 data points
IEEE Transactions on Pattern Analysis and Machine Intelligence, 1999
We present a neural network-based face detection system. A retinally connected neural network exa... more We present a neural network-based face detection system. A retinally connected neural network examines small windows of an image, and decides whether each window contains a face. The system arbitrates between multiple networks to improve performance over a single network. We use a bootstrap algorithm for training the networks, which adds false detections into the training set as training progresses. This eliminates the difficult task of manually selecting non-face training examples, which must be chosen to span the entire space of non-face images. Comparisons with other stateof-the-art face detection systems are presented; our system has better performance in terms of detection and false-positive rates.
Example-Based Learning for View-Based Human Face Detection
IEEE Transactions on Pattern Analysis and Machine Intelligence, 1998
Abstract—We present an example-based learning approach for locating vertical frontal views of hum... more Abstract—We present an example-based learning approach for locating vertical frontal views of human faces in complex scenes. The technique models the distribution of human face patterns by means of a few view-based “face” ...
Uploads
Papers by Shinta Sintieya Poetri