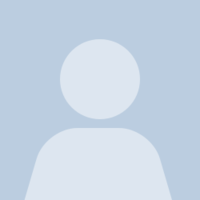
Mohd Marhaban
Related Authors
Francisco A . Monteiro
ISCTE - University Institute of Lisbon (ISCTE-IUL)
Basem M . El-Halawany
Benha University
Danielo G Gomes
Universidade Federal do Ceará
Suhail Shahab
Universiti Malaysia Pahang (UMP)
Steffen Beyme
University of British Columbia
Ayib Rosdi Bin Zainun
Universiti Malaysia Pahang (UMP)
Dinest Apakes
Anna University
Surendra Lal Hada
Kathmandu University
Armando Marques-Guedes
UNL - New University of Lisbon
Gwen Robbins Schug
University of North Carolina at Greensboro
Uploads
Papers by Mohd Marhaban
(QARXNN) model is able to demonstrate its ability for identification
and prediction highly nonlinear system. The model is
simplified by a linear correlation between the input vector and its
nonlinear coefficients. The coefficients are used to parameterize
the input vector performed by an embedded system called as state
dependent parameter estimation (SDPE), which is executed by
multi layer parceptron neural network (MLPNN). SDPE consists
of the linear and nonlinear parts. The controller law is derived
via SDPE of the linear and nonlinear parts through switching
mechanism. The dynamic tracking controller error is derived then
the stability analysis of the closed-loop controller is performed
based Lyapunov theorem. Linear based adaptive robust control
and nonlinear based adaptive robust control is performed with
the switching of the linear and nonlinear parts parameters based
Lyapunov theorem to guarantee bounded and convergence error
(QARXNN) model is able to demonstrate its ability for identification
and prediction highly nonlinear system. The model is
simplified by a linear correlation between the input vector and its
nonlinear coefficients. The coefficients are used to parameterize
the input vector performed by an embedded system called as state
dependent parameter estimation (SDPE), which is executed by
multi layer parceptron neural network (MLPNN). SDPE consists
of the linear and nonlinear parts. The controller law is derived
via SDPE of the linear and nonlinear parts through switching
mechanism. The dynamic tracking controller error is derived then
the stability analysis of the closed-loop controller is performed
based Lyapunov theorem. Linear based adaptive robust control
and nonlinear based adaptive robust control is performed with
the switching of the linear and nonlinear parts parameters based
Lyapunov theorem to guarantee bounded and convergence error