Papers by Mohamed Sadek Kemmouche

2010 13th International Conference on Information Fusion, 2010
Статья посвящена зарождению и развитию института адвокатуры в Великом княжестве Литовском в XVI в... more Статья посвящена зарождению и развитию института адвокатуры в Великом княжестве Литовском в XVI в. Магдебургское право оказало влияние на становление адвокатуры. Статут 1529 г. посвятил всего одну статью адвокатской деятельности. Она запрещала иностранцам быть адвокатами. В период 1529-1566 гг. происходило сближение статутного, обычного и Магдебургского права. На этой основе продолжил развиваться институт адвокатуры. Статут 1566 г. завершил оформление института адвокатуры. Он содержал больше статей, посвященных адвокатам. Статут 1566 г. сформулировал некоторые правила адвокатской этики, установил ответственность за нарушение адвокатом своих обязанностей. Предательство адвокатом своего клиента каралось смертью. Статут 1588 г. стал наиболее систематизированным законом Великого княжества Литовского, который подробно регламентировал порядок судебной защиты. Он продолжил традиции в отношении регулирования адвокатуры. Ключевые слова: Великое княжество Литовское, Магдебургское право, Статут, прокуратор, адвокат.
Object detection using Gaussian mixture-based optical flow modelling
The Imaging Science Journal, 2013
ABSTRACT
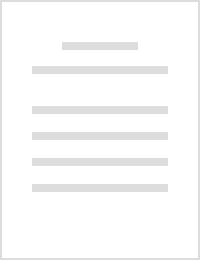
A Gaussian mixture based optical flow modeling for object detection
3rd International Conference on Imaging for Crime Detection and Prevention (ICDP 2009), 2009
This paper treats the problem of reliable foreground object classification from scene background ... more This paper treats the problem of reliable foreground object classification from scene background in an image sequence. Efficient solution for this problem is crucial in the development of automatic video surveillance and tracking systems. We present a background/foreground segmentation approach based on a subtraction of background model that combine color and optic flow information of the scene. A new technique to integrate optical flow information with color information in the background model is developed. The optical flow information complements a color background subtraction model based on spatially global Gaussian mixture. Experimental results that test the proposed approach showed better segmentation than color background/foreground segmentation approach. (6 pages)

This paper develops two background/foreground segmentation approaches based on a foreground subtr... more This paper develops two background/foreground segmentation approaches based on a foreground subtraction from a background model, which uses scene colour and motion information. In the first approach, the background is modelled by a Spatially Global Gaussian Mixture Model (SGGMM) based on scene Red, Green, Blue (RGB) colours. This model is then used to estimate motion based optical flow, which helps indirectly in the scene segmentation decision. In an alternative approach, motion based optical flow information is combined with colours as an augmented feature vector to model the background. For both approaches, we introduce an estimation method of the optical flow uncertainty statistics to use them in the background modelling. Evaluation results for both approaches based on indoor and outdoor image sequences show that the estimated background model is good at describing optical flow uncertainties and the segmentation obtained is better than colour only based segmentation. 1-INTRODUCTION In many applications, such as video surveillance, one of the most important challenges is how to automatically and correctly classify parts of the scene as foreground or background. For this task, a particularly popular approach is based on background subtraction. The idea behind background subtraction is to compare the current image with a reference image of the background, and thus decide what is, and what is not, part of the background. However, one problem with background/foreground subtraction is in obtaining the reference image when, for example, large parts of the background are occluded by moving foreground objects or parts of the background are not seen for a long time. Other issues could be: illumination changes which can easily be misinterpreted as foreground objects, the model of the background becoming obsolete, foreground objects casting shadows where the shadow might be interpreted as foreground, objects believed to be background could move, and moving foreground objects could stop for a long time. To deal with all these problems, one proposed solution is to statistically model the background, which is updated over time to account for progressive scene changes [1-6]. Optical flow is an important feature that provides information about the displacements of brightness patterns in the scene and has been largely used for object segmentation in vision systems. Optical flow calculation aims to estimate 2D apparent motion information from subsequent images. To deal with motion uncertainties, many research works have presented motion estimation approaches where optical flow distributions are able to represent any kind of motion uncertainty. Most of these developed approaches are inaccurate in some parts of the image even if they are robust globally in the overall image. This is due to fact that they do not take into account background modelling and thus their parameters are not changed according to changes in background appearance [6]. This might cause problems for optical flow based approaches if applied on their own in some image segmentation tasks even if the background initialization process is looked at carefully. In this work, we introduce an innovative background subtraction technique where the background is modelled by a Spatially Global Gaussian Mixture Model (SGGMM) [3], based on RGB colours and motion based optical flow features. Two techniques to include the optical flow information in the background model are presented: In the first version the SGGMM is estimated based on the RGB colours only and then used to estimate the optical flow uncertainty model based on spatiotemporal gradient. The estimated optical flow uncertainty model will be integrated with the RGB colours model to obtain a SGGMM based on feature vector combining both colour and optical flow, which is then used to generate the background subtraction support map. In the second version, the SGGMM background model estimation is based on a feature vector combining both RGB colours and optical flow information. To deal with motion induced noise, we introduced an estimation method of the optical flow uncertainty statistics which are used in the background
In this paper, we present a recursive estimation algorithm for nonlinear non-Gaussian tracking sy... more In this paper, we present a recursive estimation algorithm for nonlinear non-Gaussian tracking systems based on an adaptive Gaussian mixture technique. This estimation cannot be efficiently performed for nonlinear non-Gaussian systems because of the complex representation of the state density. To alleviate this complexity, approximation techniques based on Gaussian mixtures are used. An adaptive mixture approximation method is used based on optimal minimization of a least squares error function between the true density and the corresponding approximation mixture. The state Gaussian mixture is propagated over time through prediction and update steps. Results in comparison with other methods show the efficiency of the proposed algorithm.
This paper treats the problem of reliable foreground object classification from scene background ... more This paper treats the problem of reliable foreground object classification from scene background in an image sequence. Efficient solution for this problem is crucial in the development of automatic video surveillance and tracking systems. We present a background/foreground segmentation approach based on a subtraction of background model that combine color and optic flow information of the scene. A new technique to integrate optical flow information with color information in the background model is developed. The optical flow information complements a color background subtraction model based on spatially global Gaussian mixture. Experimental results that test the proposed approach showed better segmentation than color background/foreground segmentation approach.
Uploads
Papers by Mohamed Sadek Kemmouche