Papers by Jhilam Mukherjee

Computers & Electrical Engineering
This paper proposes a novel prediction model for detection of sub-centimeter pulmonary nodules fr... more This paper proposes a novel prediction model for detection of sub-centimeter pulmonary nodules from thoracic computed tomography (CT) images. We perform multi-level thresholding for lesion segmentation followed by penalised multinomial classifier for identifying the lesion type. We have considered 17,893 sub-centimeter pulmonary structures from an independent dataset collected retrospectively from Peerless Hospital, Kolkata to develop the classification model. An important feature of this dataset is class imbalance i.e. pulmonary vessels account for 70% of the structures while bones, scars, and nodules respectively account for 2%, 5%, and 23%. To the best of our knowledge, only a few researchers have addressed the issue of imbalanced classes for lung computed tomography (CT) images. The specificity, sensitivity and misclassification rates indicate that the method has the potential to reduce the number of undetected early stage lesions.
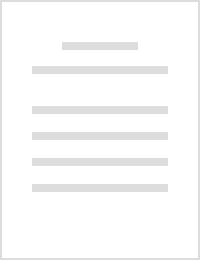
A Novel Technique for Contrast Enhancement of Chest X-Ray Images Based on Bio-Inspired Meta-Heuristics
Advances in Intelligent Systems and Computing, 2018
Chest radiography is considered as one of the most important radiological tools in pulmonary dise... more Chest radiography is considered as one of the most important radiological tools in pulmonary disease diagnosis. Due to the generation of low contrast images of X-ray machines, the detection of the lesions is a difficult issue and prone to error for a radiologist. Hence, a contrast enhancement algorithm is an obvious choice to enhance the contrast of the image, thus increasing the accuracy of detection of the lesions. This paper not only proposes a new algorithm for contrast enhancement of digital chest X-ray images using particle swarm optimization (PSO), but it also introduces a benchmark dataset of digital chest radiographs to justify the supremacy of our proposed algorithm over that of state-of-the-art contrast enhancement algorithms.

Biocybernetics and Biomedical Engineering, 2020
Early detection of lung cancer is the major challenge for physicians to treat and control this de... more Early detection of lung cancer is the major challenge for physicians to treat and control this deadly disease whose primary step is to detect pulmonary nodule from thoracic computed tomography (CT) images. In view of increasing the accuracy of the pulmonary nodule computer aided detection methodology, this paper proposes a novel pulmonary nodule detection methodology that can aid early diagnosis of the patients. The study has considered high resolution computed tomography (HRCT) images from two public datasets LIDC and Lung-TIME and an independent dataset, created in collaboration between Peerless Hospital Kolkata and University of Calcutta. The key feature of the test dataset is that the class features are imbalanced in nature. The structures associated with lung parenchyma are segmented using parameterized multi-level thresholding technique, grayscale morphology, and rolling ball algorithm. Then random under sampling is implemented to overcome the imbalance class problem, followed by a feature selection methodology using particle swarm optimization (PSO). The nodule and non-nodule classification are performed by implementing ensemble stacking. Indeed, it has been observed that there exists insufficient published literature that has been considered similar looking pulmonary abnormalities as non-nodule objects as well as imbalance class problem and feature selection algorithm to design an automated, accurate and robust model for automated detection of the pulmonary nodule. In reference to the LIDC dataset, the false positive, false negative detection rates and sensitivity are 1.01/scan, 0.56/scan and 99.01% respectively, which is an improvement in terms of accuracy as compared to the existing state-of-the-art research works.
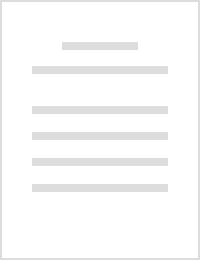
A Comparative Analysis of Image Segmentation Techniques Toward Automatic Risk Prediction of Solitary Pulmonary Nodules
Advances in Intelligent Systems and Computing, 2015
Lung cancer is considered as a leading cause of death throughout the globe. Manual interpretation... more Lung cancer is considered as a leading cause of death throughout the globe. Manual interpretation of cancer detection is time consuming and thus increases the death rate. With the help of improvement in medical imaging technology, a computer-aided diagnostics system could be an aid to combat this disease. Automatic segmentation of a region of interest is one of the most challenging problem in medical image analysis. An inaccurate segmentation of solitary pulmonary nodule may lead to an erroneous prediction of the disease. In this paper, we perform a comparative study among the available segmentation techniques, which can automatically segment the solitary pulmonary nodules from high-resolution computed tomography (CT) images and then we propose a computerized lung nodule risk prediction model based on the best segmentation technique.
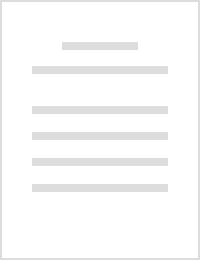
Automatic Detection and Classification of Solitary Pulmonary Nodules from Lung CT Images
2014 Fourth International Conference of Emerging Applications of Information Technology, 2014
Cancer is one of the fatal diseases, posing threat to human life. Among different types of cancer... more Cancer is one of the fatal diseases, posing threat to human life. Among different types of cancer, lung cancer can be considered as one of the most most deadly one. Lung nodules are small white spots that appear in lung parenchyma. Lung nodules are primarily of two types, solitary pulmonary nodules and juxtrapleural nodules. Solitary pulmonary nodules are round in shape, whereas juxtapleural nodules have a worm like shape, which are generally introduced through metathesis from the other cancerous organ of the human body. All lung nodules are not cancerous. Each of them has certain geometric features, upon which the nodules can be classified into cancerous and non-cancerous. Chest radiographs and computed tomography (CT) scan images are important for the purpose of diagnosis of the lung cancer. Manual detection of cancerous lung nodule is a very challenging task as it is time consuming and prone to human error. An automated detection is therefore needed for faster detection of malignant and benign lung nodules based on the shape features of the nodules. This automated detection will also help to reduce the cost of diagnosis by selectively choosing only the malignant nodule for biopsy tissue culture discarding the benign lung nodule. In this paper, we have proposed a novel method that detects and categorizes solitary pulmonary nodules responsible for lung cancer from lung CT images. Our method reduces variability in detection by automatic segmentation and classification of nodules. The experimental results are promising in respect to classification of lung nodules as malignant or benign.
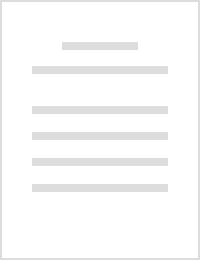
Variability of Cobb angle measurement from digital X-ray image based on different de-noising techniques
International Journal of Biomedical Engineering and Technology, 2014
ABSTRACT Scoliosis is a 3D deformity of the spine, which is characterised by both lateral curvatu... more ABSTRACT Scoliosis is a 3D deformity of the spine, which is characterised by both lateral curvature and vertebral rotation. Cobb angle measurement is the golden method for diagnosis of scoliosis. With the increasing trend of digital imaging-based diagnosis system, computerised Cobb angle measurement is gaining much popularity. In general, digital X-ray images are very sensitive to noise, so a good pre-processing step for noise removal is necessary to increase the accuracy and reduce the variability of computerised Cobb angle measurement method. In this paper, we have presented a review work on computerised Cobb angle measurement from digital X-ray images based on the different popular state-of-the-art de-noising techniques. This study helps in selecting the de-noising technique that best suits with different steps of the Cobb angle method and generates a more reliable angular measurement. This type of study based on de-noising technique for reducing Cobb angle measurement variability is the first of its kind. MATLAB2011Rb image processing toolbox was used for the simulation and verification of our proposed methodology.
Bengal Physician Journal
The goal of artificial intelligence (AI) is to imitate cognitive processes in humans. Due to expa... more The goal of artificial intelligence (AI) is to imitate cognitive processes in humans. Due to expanding availability of health care data and the quick advancement of analytics techniques, it is bringing about a paradigm shift in health care. Recent advancements in the digitized data-collecting process have allowed AI applications to expand into previously believed to be the sole domain of human experts. In this article, we discuss current developments in AI technology across a range of health care applications, as well as the challenges that must yet to be overcome to implement a precise medical AI system.
A connectionist approach for gray level image segmentation
Page 1. A Connectionist Approach for Gray Level Image Segmentation VVVinodt Sant anu C haudhur y ... more Page 1. A Connectionist Approach for Gray Level Image Segmentation VVVinodt Sant anu C haudhur y t t J.Mukherj ee$ S. Ghose t tDept. of Computer Sc. & Engg., Indian Institute of Technology, Kharagpur, India - 721302 $Dept. of Electronics & Elect. Commn. ...

Data Management, Analytics and Innovation, 2019
Lung cancer has the highest prevalence in cancer-related deaths due to its rapid progression and ... more Lung cancer has the highest prevalence in cancer-related deaths due to its rapid progression and it is detected at advanced stages. The paper proposes a novel method for predicting the risk of being malignant of Pulmonary Nodule (PN), presence of which can be an indication of lung cancer, with the motive to reduce the number of unnecessary biopsies and prevent anxiety among the patients. The study has considered different morphological features along with the clinical history of the patient having the particular nodule as described in medical literature. Depending upon these features, we have classified the risk of being malignant of pulmonary nodule into two classes, namely, low-risked or benign and high-risked or malignant. The entire dataset required to design the model is collected from a retrospective dataset, containing 476 (401 Malignant or high-risked and 75 low-risked or benign) PNs. The classification is performed by Recursive Partitioning Algorithm (RPA). RPA not only improves the accuracy but also helps to interpret how the morphological features are classifying the true risk of being malignant of the nodules.

Scoliosis is a 3D deformity of the spine, which is characterised by both lateral curvature and ve... more Scoliosis is a 3D deformity of the spine, which is characterised by both lateral curvature and vertebral rotation. Cobb angle measurement is the golden method for diagnosis of scoliosis. With the increasing trend of digital imaging-based diagnosis system, computerised Cobb angle measurement is gaining much popularity. In general, digital X-ray images are very sensitive to noise, so a good pre-processing step for noise removal is necessary to increase the accuracy and reduce the variability of computerised Cobb angle measurement method. In this paper, we have presented a review work on computerised Cobb angle measurement from digital X-ray images based on the different popular state-of-the-art de-noising techniques. This study helps in selecting the de-noising technique that best suits with different steps of the Cobb angle method and generates a more reliable angular measurement. This type of study based on de-noising technique for reducing Cobb angle measurement variability is the first of its kind. MATLAB2011Rb image processing toolbox was used for the simulation and verification of our proposed methodology.
Uploads
Papers by Jhilam Mukherjee