Papers by Guillaume Wacquet
RclusTool: Graphical Toolbox for Clustering and Classification of Data Frames
Multiscale harmonised automated observations of phytoplankton biomass, diversity and productivity dynamics in the English Channel and North Sea as part of the coastal Pilot Super Site approach (JERICO S3)
Phytoplankton automated in vivo/in situ observations: towards a new approach of Essential Ocean Variables of marine coastal ecosystems
Monitoring of phytoplankton and Harmful Algal Blooms in coastal waters by combining innovative semi-automated tools (scanning flow cytometry & spectral fluorometry)
HAL (Le Centre pour la Communication Scientifique Directe), Mar 30, 2015

Bioinformatics, Oct 25, 2022
In recent years, Deep Learning (DL) has been increasingly used in many fields, in particular in i... more In recent years, Deep Learning (DL) has been increasingly used in many fields, in particular in image recognition, due to its ability to solve problems where traditional machine learning algorithms fail. However, building an appropriate DL model from scratch, especially in the context of ecological studies, is a difficult task due to the dynamic nature and morphological variability of living organisms, as well as the high cost in terms of time, human resources and skills required to label a large number of training images. To overcome this problem, Transfer Learning (TL) can be used to improve a classifier by transferring information learnt from many domains thanks to a very large training set composed of various images, to another domain with a smaller amount of training data. To compensate the lack of 'easy-to-use' software optimized for ecological studies, we propose the EcoTransLearn Rpackage, which allows greater automation in the classification of images acquired with various devices (FlowCam, ZooScan, photographs, etc.), thanks to different TL methods pre-trained on the generic ImageNet dataset. EcoTransLearn is an open-source package. It is implemented in R and calls Python scripts for image classification step (using reticulate and tensorflow libraries). The source code, instruction manual and examples can be found at .

This is a summary of the activities and results of JERICO-NEXT Work Package 3 Innovations in Tech... more This is a summary of the activities and results of JERICO-NEXT Work Package 3 Innovations in Technology and Methodology, Task 3.1 Automated platform for the observation of phytoplankton diversity in relation to ecosystem services. The aim is to provide an advanced report on the last developments dedicated to the observation of the phytoplankton diversity by applying novel techniques on automated platforms. The work was carried out in close connection with task 4.1 Biodiversity of plankton, harmful algal blooms and eutrophication. The partners involved in these developments are CNRS, SYKE, SMHI, HZG, RWS, VLIZ CEFAS and Ifremer. Subcontractors in WP4, task 4.1 are WHOI, Scanfjord AB, Tomas Rutten b.v., CytoBuoy b.v. and UGent - PAE. The work was carried out mainly in the field with activities in the Baltic Sea, the Kattegat-Skagerrak, the Celtic seas-English Channel-North Sea Area, the Western Mediterranean, as well as in shared studies with other WP3.4 and WP4.4 in the Bay of Biscay...

Dans les systèmes d'aide à la décision, sont généralement à disposition des données numérique... more Dans les systèmes d'aide à la décision, sont généralement à disposition des données numériques abondantes et éventuellement certaines connaissances contextuelles qualitatives, disponibles a priori ou fournies a posteriori par retour d'expérience. Les performances des approches de classification, en particulier spectrale, dépendent de l'intégration de ces connaissances dans leur conception. Les algorithmes de classification spectrale permettent de traiter la classification sous l'angle de coupes de graphe. Ils classent les données dans l'espace des vecteurs propres de la matrice Laplacienne du graphe. Cet espace est censé mieux révéler la présence de groupements naturels linéairement séparables. Dans ce travail, nous nous intéressons aux algorithmes intégrant des connaissances type contraintes de comparaison. L'espace spectral doit, dans ce cas, révéler la structuration en classes tout en respectant, autant que possible, les contraintes de comparaison. Nous pr...
zooimage: Analysis of Numerical Plankton Images
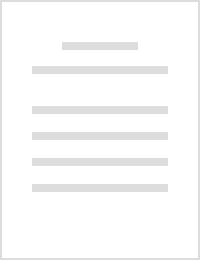
DCSMM2018 - HP - D1C6 - Ratio de la concentration de surface en chlorophylle a contenue dans les dinoflagellés sur celle contenue dans les diatomées (2012-2016)
Le critère de définition du bon état écologique pour le descripteur D1 – Habitats pélagiques est ... more Le critère de définition du bon état écologique pour le descripteur D1 – Habitats pélagiques est le critère D1C6 : « les caractéristiques du type d'habitat, notamment sa structure biotique et abiotique et ses fonctions, ne subissent pas d'effets néfastes dus à des pressions anthropiques ». Un des indicateurs retenus, développés pour les communautés phyto- et zooplanctoniques, est l'indicateur PH1, basé sur la dynamique comparée de groupes fonctionnels (ou paires de formes de vie). Les échelles d'évaluation géographiques considérées sont les masses d'eau côtières de la Directive Cadre sur l'Eau (DCE) à la côte et les paysages marins mensuels au large, combinés sur une seule couche annuelle pour les besoins de l'évaluation. Le PH1 est en cours de développement et d'adaptation aux données disponibles dans les SRM, la période de référence et les seuils restent à définir pour cet indicateur. L'évaluation du bon état écologique n'a donc pu être effe...

Jean-Baptiste ROMAGNAN, Ifremer de Nantes, 44311 Cedex 03, Rue de l'Île d'Yeu, 44980 Nant... more Jean-Baptiste ROMAGNAN, Ifremer de Nantes, 44311 Cedex 03, Rue de l'Île d'Yeu, 44980 Nantes Klas Ove Möller: Institute of Coastal Research, Helmholtz-Zentrum Geesthacht, Germany Luis Felipe Artigas & Guillaume Wacquet: CNRS, Univ. Lille, Univ. Littoral Côte d’Opale, UMR 8187, Laboratory of Oceanology and Geosciences, Wimereux, France Gérald Grégori & Melilotus Thyssen, Aix-Marseille Université, CNRS, IRD, Univ. Toulon, UMR 7294, Mediterranean Institute of Oceanology, Marseille, France Klaas Deneudt & Simon Claus: Flanders Marine Institute, Ostend, Belgium Bengt Karlson: Swedish Meteorological and Hydrological Institute, Oceanographic Research Lars Stemmann, Fabien Lombard, Jean Olivier irisson, Lionel Guidi, Dodji Soviadan: Sorbonne Université, UMR 7093, Laboratoire d’Océanographie de Villefranche sur Mer Alain Lefebvre: Ifremer, Laboratory of Environment and resources, Boulogne sur mer, France Jukka Seppälä, Kaisa Kraft: Marine Research Centre, Finnish Environment Institute...

In order to better understand phytoplankton temporal and spatial distribution in coastal systems,... more In order to better understand phytoplankton temporal and spatial distribution in coastal systems, automated in vivo approaches are being deployed since the last decade. These innovative techniques provide new insights into the detection of phytoplankton community changes affecting growth rates, size structure, taxonomic and/or pigmentary composition, which can occur at different time and spatial scales, evidencing rapid as well as long-term changes in environmental conditions. When implemented in automated environmental monitoring platforms, as fixed stations, moorings, research vessels and/or ships of opportunity, these techniques can represent valuable early-warning systems of community changes, as the occurrence of blooms and, in particular, of harmful algal blooms (HAB), which can lead to disruption of marine food webs and mass mortalities of marine organisms and which are of special interest in areas of fishing, aquaculture and tourism. Therefore, there is an urgent need to imp...
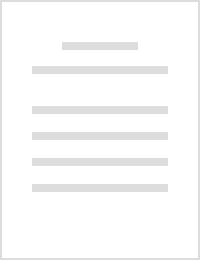
Semi-supervised K-Way Spectral Clustering with Determination of Number of Clusters
Studies in Computational Intelligence, 2013
ABSTRACT In this paper, we propose a new K-way semi-supervised spectral clustering method able to... more ABSTRACT In this paper, we propose a new K-way semi-supervised spectral clustering method able to estimate the number of clusters automatically and then to integrate some limited supervisory information. Indeed, spectral clustering can be guided thanks to the provision of prior knowledge. For the automatic determination of the number of clusters, we propose to use a criterion based on an outlier number minimization. Then, the prior knowledge consists of pairwise constraints which indicate whether a pair of objects belongs to a same cluster (Must-Link constraints) or not (Cannot-Link constraints). The spectral clustering then aims at optimizing a cost function built as a classical Multiple Normalized Cut measure, modified in order to penalize the non-respect of these constraints. We show the relevance of the proposed method with some UCI datasets. For experiments, a comparison with other semi-supervised clustering algorithms using pairwise constraints is proposed.
Dissimilarity-Based Classification of Multidimensional Signals by Conjoint Elastic Matching: Application to Phytoplanktonic Species Recognition
Communications in Computer and Information Science, 2009
The paper describes a classification method of multidimensional signals, based upon a dissimilari... more The paper describes a classification method of multidimensional signals, based upon a dissimilarity measure between signals. Each new signal is compared to some reference signals through a conjoint dynamic time warping algorithm of their time features series, of which proposed cost function gives out a normalized dissimilarity degree. The classification then consists in presenting these degrees to a classifier, like
Pattern Recognition Letters, 2013
Limnology and Oceanography
Plankton imaging systems supported by automated classification and analysis have improved ecologi... more Plankton imaging systems supported by automated classification and analysis have improved ecologists' ability to observe aquatic ecosystems. Today, we are on the cusp of reliably tracking plankton populations with a suite of lab-based and in situ tools, collecting imaging data at unprecedentedly fine spatial and temporal scales. But these data streams have potential well beyond examining the abundances of different taxa; the individual images themselves contain a wealth of information on functional traits. Here we outline traits that could be measured from image data, suggest computer vision approaches to extract functional trait information from the images, and discuss promising avenues for novel studies. The approaches we discuss are data agnostic and are broadly applicable to other aquatic or terrestrial organisms.
International Joint Conference on Computational Intelligence, 2011
In this paper, we propose a semi-supervised spectral clustering method able to integrate some lim... more In this paper, we propose a semi-supervised spectral clustering method able to integrate some limited supervisory information. This prior knowledge consists of pairwise constraints which indicate whether a pair of objects belongs to a same cluster (Must-Link constraints) or not (Cannot-Link constraints). The spectral clustering then aims at optimizing a cost function built as a classical Multiple Normalized Cut measure, modified in order to penalize the non-respect of these constraints. We show the relevance of the proposed method with an illustrative dataset and some UCI benchmarks, for which two-class and multi-class problems are dealt with. In all examples, a comparison with other semi-supervised clustering algorithms using pairwise constraints is proposed.
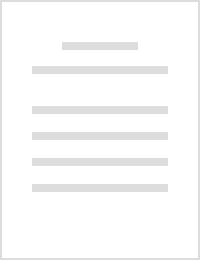
Finalisation d’un outil préopérationnel FlowCAM / ZooPhytoImage. Action9 - FlowCAM ZooPhytoImage. Livrable n° 3. Rapport final
Aujourd'hui, la methode la plus utilisee pour l'analyse des echantillons dans le cadre du... more Aujourd'hui, la methode la plus utilisee pour l'analyse des echantillons dans le cadre du REPHY est celle de la microscopie. Cependant, cette methode souffre de nombreux inconvenients : niveaux de competence differents selon les operateurs, taxons pouvant faire l'objet d'identifications erronees, observateur sujet a la fatigue et a la deconcentration, erreurs non quantifiables. C'est pourquoi, differents axes d'evolution ont ete proposes pour adapter le FlowCAM couple a ZooPhytoImage aux exigences du REPHY qui sont la justesse et la repetabilite de la mesure. L'objectif de ces nouveaux outils est d'offrir un gain de temps tangible aux observateurs REPHY par rapport aux observations au microscope optique. Le temps d'analyse etant un point clef de ce systeme, des etudes ont ete menees afin de pouvoir realiser une acquisition rapide. En ce sens, afin que la mesure soit representative du milieu, au moins 2000 particules doivent etre comptees. Pour red...
Graphical Toolbox for Clustering and Classification of Data Frames [R package RclusTool version 0.91.3]
Uploads
Papers by Guillaume Wacquet