Papers by Dr. Tanupreet Sabharwal
Advancement of Intelligent Computational Methods and Technologies
CRC Press eBooks, Apr 2, 2024

Complex & intelligent systems, Mar 13, 2024
Facial identification for surgical and non-surgical datasets is getting popular. The reason behin... more Facial identification for surgical and non-surgical datasets is getting popular. The reason behind this popularity is the growing need of a robust facial recognition system which is consistent to occlusion, spoofing attacks and last but most important plastic surgery effects. Plastic therapies are undertaken by individuals to beautify their external appearance but it is also undertaken by impostors to commit crimes and falsify their true identities. This research work aims at developing a facial recognition system which can identify genuine and impostor pairs. The projected methodology optimizes face detection via Back-Propagation Neural Network (BPNN) and dimensionality reduction by means of Speeded Up Robust Features followed by Multi-K-Nearest-Neighbor technique. The novelty is the production of an innovative-fangled T-Database which trains the BPNN. Thus, BPNN converges faster and achieves higher recognition. The proposed scheme is not applied till date on a medically altered dataset. We have applied five distance metrics and integrated them to acquire T-Dataset, which is fed to the BPNN. This scheme is tested on surgical and non-surgical datasets and it is deduced that higher recognition is achieved with non-surgical databases as compared to surgical ones. For both surgical and non-surgical datasets, the computational cost attained is the modest. Human identification • Facial recognition after plastic surgery (FRAPS) • Speeded Up Robust Features (SURF) • Multi K-Nearest-Neighbor • Correlation metric • Euclidean metric • Canberra metric • Manhattan metric • Mahalanobis metric • Back-Propagation Neural Network (BPNN) • AT&T database • YALE database • Plastic surgery facial dataset • Recognition rate/identification accuracy (RR) • Computation time (CT) • Expected error rate (EER) B Tanupreet Sabharwal

Many people adopt cosmetic or medical changes for aesthetic or therapeutic objectives. The paper ... more Many people adopt cosmetic or medical changes for aesthetic or therapeutic objectives. The paper proposes a human identification technique for differentiating between samples taken before and after surgery. The system operates in three stages. First, pre-, and post-surgery images are preprocessed (cropped/converted to grayscale), then fuzzy edge detection is performed. Next, prominent features are extracted using SURF (Speeded Up Robust Features) extractor, and finally, a KNN classifier is used to determine which pairs are genuine and which are impostor. Utilizing fuzzy edge detection as a pre-processing step for appropriate (non-redundant) feature selection is the innovation/novelty in this method (dimensionality reduction). The selected features acquired by fuzzy detection are then subjected to SURF. The purpose of SURF is to compute operators quickly by utilizing box filters, rotation invariance, and anti-blur features. Since edges are local in nature and SURF is a local extracto...
Deep facial recognition after medical alterations
Multimedia Tools and Applications

International Journal of Information Technology, 2020
With technological innovations soaring high researchers are able to develop more efficient and ad... more With technological innovations soaring high researchers are able to develop more efficient and adaptable biometric recognition systems. Biometric systems work by matching different biometric traits to templates in a database. Recognition systems use soft as well as hard biometrics. Soft biometrics are facial or birth marks, gender, race etc. whereas hard biometrics include fingerprint, iris, retina, face etc. In this paper we have proposed a methodology by clubbing an existing facial recognition system with the statistics attained from facial marks in order to mend the recognition rate between before after surgically altered images of human beings. Firstly an algorithm is introduced for spontaneously identifying facial marks, which are represented using Histograms of Oriented Gradients (HOG), secondly they are accorded to their location in the face image. The proposed methodology is implemented on the plastic surgical facial dataset. Widespread experiments are lead in order to show the efficacy of the anticipated facial mark detection process, and to substantiate the benefits of using the statistics of facial marks on top of customary face acknowledgement schemes. The above mentioned methodology is till date not implemented on surgically altered facial image samples.

Journal of Information Security and Applications, 2019
American society of plastic surgeons (ASPS) Regions of interest (ROIs) Speeded up robust features... more American society of plastic surgeons (ASPS) Regions of interest (ROIs) Speeded up robust features (SURF) K-nearest-neighbour (KNN) Weighted score level fusion Expected error rate (EER) Recognition rate (RR) Computation time (CT) a b s t r a c t Plastic surgery alters original facial features of an individual thereby making Face Recognition after plastic surgery difficult. Cosmetic procedures introduce geometrical deviations which are difficult to analyze by state of the art facial identification procedures. Here a region based score level fusion approach for local facial features is proposed to equalize former and latter surgery images. Steps involved in the recognition process are; firstly identifying the ROIs (areas/regions of interest/concern) of before and after surgery images. ROIs are eyes, nose and mouth regions; feature extraction from identified regions via Speeded Up Robust Features and K Nearest Neighbour techniques; region wise and full face geometrical distance calculation between matched feature vectors of pre and post surgery image samples by a distance metric (sum of squared differences); final recognition rate calculation by weighted score level fusion. The projected procedure gives recognition of 89.7% for local surgical treatment and 87% for global surgery.

Artificial Intelligence Review, 2018
Biometric recognition plays a vital role in our daily lives. Face recognition is a subset of biom... more Biometric recognition plays a vital role in our daily lives. Face recognition is a subset of biometric recognition. Face verification and identification processes are prone to plastic surgery challenges which are commonly used nowadays to alter facial features for good looking demonstration. With increasing trend in technology and intellect robust biometric recognition systems are developed for human recognition after plastic surgery. However, these systems have some limitations because recognition after plastic surgery is affected by lightning, aging, pose, expressions, disguise and occlusion effects. In this survey, we aim to highlight the mitigating effects of cutting edge plastic surgical operations. These procedures lead to medical identity thefts, which is a serious offense for human community as an individual's identity is forged. Thus, this makes one's safety a critical issue and human recognition after plastic surgery a crucial challenge. Since the existing methods for human recognition after plastic surgical operations are not promising, in the current scenario plastic surgical operations secure above facial recognition. A number of existing biometric recognition algorithms for face images have been opted such as principal component analysis, Fisher/linear discriminant analysis, local feature analysis, local/circular binary patterns, speeded up robust features, granular system, correlation based approach, evolutionary granular/genetic approach, grouping recognition by parts and sparse demonstration approach, geometrical face recognition after plastic surgery, feature/texture based fusion scheme and deep convolutional neural networks (DCNN). The validation metrics used for the evaluation of recognition techniques are expected error rate, recognition rate, half total error rate and F-score. All algorithms are tested on an open plastic surgery facial dataset containing 1800 before and after surgery image samples pertaining to 900 humans. For a particular human being, two front facing image samples with appropriate luminance and unbiased gesture are taken: the former is taken pre cosmetic procedure and the latter is taken post cosmetic procedure. It has been deduced that feature and texture based fusion approach gives best results till date. It is predicted that DCNN has full potential of giving consistent results on surgical databases as it is already validated on non surgical databases. The need of a novel human identification system which is steady to the anomalies posed by plastic surgical operations is highlighted in this survey.
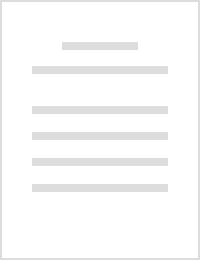
A Comparative Analysis of Various Deep Learning Models for Facial Recognition
2019 6th International Conference on Computing for Sustainable Global Development (INDIACom), 2019
The main idea behind facial recognition is to identify human faces in images as well as videos. M... more The main idea behind facial recognition is to identify human faces in images as well as videos. Multiple techniques have been developed till date to improve the performance of facial recognition systems. Earlier methods were not competent of capturing prominent facial information due to pose, illumination, occlusion, resolution and skin color effects. A new emerging technique known as deep learning is showing impressive improvement in performance metrics for facial identification. With recent developments in deep learning techniques, facial detection systems can learn data by themselves and can extract useful features. It has been shown to exhibit promising recognition results for diverse real time applications. In this paper, we have examined deep convolutional neural networks and discussed various models related to deep learning such as VGGNet, GoogleNet, SqueezeNet, AlexNet and ResNet and have compared these with respect to various evaluation metrics such as error rate, predictio...
Uploads
Papers by Dr. Tanupreet Sabharwal