Papers by Alessandra Guglielmi

Flexible Services and Manufacturing Journal
Donor profiling and donation prediction are two key tasks that any blood collection center must f... more Donor profiling and donation prediction are two key tasks that any blood collection center must face. Profiling is important to target promotion campaigns, recruiting donors who will guarantee a high production of blood units over time. Predicting the future arrivals of donors allows to size the collection center properly and to provide reliable information on the future production of blood units. Both tasks can be addressed through a statistical prediction model for the intensity function of the donation event. We propose a Bayesian model, which describes this intensity as a function of individual donor’s random frailties and their fixed-time and time-dependent covariates. Our model explains donors’ behaviors from their first donation based on their individual characteristics. We apply it to data of recurrent donors provided by the Milan department of the Associazione Volontari Italiani del Sangue in Italy. Our method proved to fit those data, but it can also be easily applied to o...

Advances in Data Analysis and Classification, 2016
We propose a Bayesian semiparametric regression model to represent mixed-type multiple outcomes c... more We propose a Bayesian semiparametric regression model to represent mixed-type multiple outcomes concerning patients affected by Acute Myocardial Infarction. Our approach is motivated by data coming from the ST-Elevation Myocardial Infarction (STEMI) Archive, a multi-center observational prospective clinical study planned as part of the Strategic Program of Lombardy, Italy. We specifically consider a joint model for a variable measuring treatment time and in-hospital and 60-day survival indicators. One of our motivations is to understand how the various hospitals differ in terms of the variety of information collected as part of the study. We are particularly interested in using the available data to detect differences across hospitals. In order to do so we postulate a semiparametric random effects model that incorporates dependence on a location indicator that is used to explicitly differentiate among hospitals in or outside the city of Milano. The model is based on the two parameter Poisson-Dirichlet prior, also known as the Pitman-Yor process prior. We discuss the resulting posterior inference, including sensitivity analysis, and a comparison with the particular submodel arising when a Dirichlet process prior is assumed.

Frontiers in Ecology and Evolution, 2021
Modeling species distributions over space and time is one of the major research topics in both ec... more Modeling species distributions over space and time is one of the major research topics in both ecology and conservation biology. Joint Species Distribution models (JSDMs) have recently been introduced as a tool to better model community data, by inferring a residual covariance matrix between species, after accounting for species' response to the environment. However, these models are computationally demanding, even when latent factors, a common tool for dimension reduction, are used. To address this issue, Taylor-Rodriguez et al. (2017) proposed to use a Dirichlet process, a Bayesian nonparametric prior, to further reduce model dimension by clustering species in the residual covariance matrix. Here, we built on this approach to include a prior knowledge on the potential number of clusters, and instead used a Pitman–Yor process to address some critical limitations of the Dirichlet process. We therefore propose a framework that includes prior knowledge in the residual covariance m...
Bollettino Della Unione Matematica Italiana, 1998
sis-statistica.it
Riassunto: In questo lavoro seguiamo un'impostazione semiparametrica nell'ambito della ... more Riassunto: In questo lavoro seguiamo un'impostazione semiparametrica nell'ambito della statistica Bayesiana per un modello AFT (Accelerated Failure Time), che indivi-dua una relazione log-lineare fra i tempi di sopravvivenza e un vettore di covariate. La componente d'errore ...
Institute of Mathematical Statistics Lecture Notes - Monograph Series, 1996
In Betro ei ai (1994) the optimization of posterior functional E π (g \ x) with respect to prior ... more In Betro ei ai (1994) the optimization of posterior functional E π (g \ x) with respect to prior measures TΓ has been considered for the class of priors defined by a number of generalized moment conditions f Hidπ < ai. Constraints of this type are very general as they include for instance, besides ordinary moment conditions, bounds on prior quantiles or bounds on marginal probabilities of data. This paper presents an algorithm for the numerical solution of the above optimization problem based on ideas suggested by the interval approach to numerical optimization as well as from semi-infinite linear programming.
Bayesian Analysis, 2013
We introduce a model for a time series of continuous outcomes, that can be expressed as fully non... more We introduce a model for a time series of continuous outcomes, that can be expressed as fully nonparametric regression or density regression on lagged terms. The model is based on a dependent Dirichlet process prior on a family of random probability measures indexed by the lagged covariates. The approach is also extended to sequences of binary responses. We discuss implementation and applications of the models to a sequence of waiting times between eruptions of the Old Faithful Geyser, and to a dataset consisting of sequences of recurrence indicators for tumors in the bladder of several patients.
Statistics & Probability Letters, 2000
We show how to approximate de Finetti's measure of a partially exchangeable sequence by a mixture... more We show how to approximate de Finetti's measure of a partially exchangeable sequence by a mixture of products of Dirichlet measures, explicitly built once the approximation error has been ÿxed. These results are used to give a general method for the elicitation of prior distributions corresponding to partially exchangeable sequences, when prior information essentially derive from available data relative to phenomena similar to that we consider.
Statistics & Probability Letters, 2006
This paper shows some new results concerning the law of the random variance V of a Dirichlet proc... more This paper shows some new results concerning the law of the random variance V of a Dirichlet process P, expressed as the solution of a stochastic equation involving the squared difference between two independent copies of the mean of P. An explicit solution of this equation is obtained via the Zolotarev transform of V. Moreover, we discuss the correspondence between the distribution of the variance and the parameter of the Dirichlet process with given total mass.

Statistical Papers, 2013
We propose a Bayesian semiparametric accelerated failure time mixed-effects model with an illustr... more We propose a Bayesian semiparametric accelerated failure time mixed-effects model with an illustrative application to a Kevlar fibre lifetime dataset (with censoring). The error is a shape-scale mixture of Weibull densities, mixed by a normalized generalized gamma random measure, encompassing the Dirichlet process. We implement an MCMC scheme, obtaining posterior credibility intervals for the predictive distributions and for the quantiles of the failure times under different stress levels. Random spool effects are taken up by the nonparametric mixture, where every component accounts for a different spool. Compared to a previous parametric Bayesian analysis, we obtain narrower credibility intervals and a better fit to the data. We also fit a similar semiparametric model, which can be seen a a special case of ours, where the error is a scale mixture of Weibull densities, mixed by a Dirichlet process, whereas the shape parameter has a parametric prior. The adequacy of the two semiparametric models is comparable, but the more general model provides a different evaluation of the posterior variance of the left tail of the lifetime distribution, which is an effect of assuming a nonparametric prior on both parameters of the Weibull density.
Bayesian first order auto-regressive latent variable models for multiple binary sequences
Statistical Modelling, 2011
Longitudinal clinical trials often collect long sequences of binary data monitoring a disease pro... more Longitudinal clinical trials often collect long sequences of binary data monitoring a disease process over time. Our application is a medical study conducted in the US by the Veterans Administration Cooperative Urological Research Group to assess the effectiveness of a chemotherapy treatment (thiotepa) in preventing recurrence on subjects affected by bladder cancer. We propose a generalized linear model with latent auto-regressive structure for longitudinal binary data following a Bayesian approach. We discuss inference as well as sensitivity to prior choices for the bladder cancer data. We find that there is a significant treatment effect in the sense that treated patients have much smaller predicted recurrence probabilities than placebo patients.

Journal of the Royal Statistical Society: Series C (Applied Statistics), 2013
Bayesian semiparametric logit models are fitted to grouped data related to in-hospital survival o... more Bayesian semiparametric logit models are fitted to grouped data related to in-hospital survival outcome of patients hospitalized with an ST-segment elevation myocardial infarction diagnosis. Dependent Dirichlet process priors are considered for modelling the random-effects distribution of the grouping factor (hospital of admission), to provide a cluster analysis of the hospitals. The clustering structure is highlighted through the optimal random partition that minimizes the posterior expected value of a suitable loss function. There are two main goals of the work: to provide model-based clustering and ranking of the providers according to the similarity of their effect on patients' outcomes, and to make reliable predictions on the survival outcome at the patient's level, even when the survival rate itself is strongly unbalanced. The study is within a project, named the 'Strategic program of Regione Lombardia', and is aimed at supporting decisions in healthcare policies.
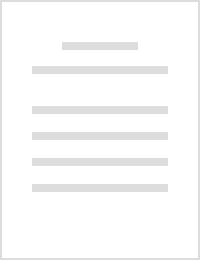
Bayesian and Conditional Frequentist Testing of a Parametric Model Versus Nonparametric Alternatives
Journal of the American Statistical Association, 2001
Testing the fit of data to a parametric model can be done by embedding the parametric model in a ... more Testing the fit of data to a parametric model can be done by embedding the parametric model in a nonparametric alternative and computing the Bayes factor of the parametric model to the nonparametric alternative. Doing so by specifying the nonparametric alternative via a Polya tree process is particularly attractive, from both theoretical and methodological perspectives. Among the benefits is a degree of computational simplicity that even allows for robustness analyses to be implemented. Default (nonsubjective) versions of this analysis are developed herein, in the sense that recommended choices are provided for the (many) features of the Polya tree process that need to be specified. Considerable discussion of these features is also provided to assist those who might be interested in subjective choices. A variety of examples involving location–scale models are studied. Finally, it is shown that the resulting procedure can be viewed as a conditional frequentist test, resulting in data-dependent reported error probabilities that have a real frequentist interpretation (as opposed to p values) in even small sample situations.

Journal of Computational and Graphical Statistics, 2014
In this paper we propose a new model for cluster analysis in a Bayesian nonparametric framework. ... more In this paper we propose a new model for cluster analysis in a Bayesian nonparametric framework. Our model combines two ingredients, species sampling mixture models of Gaussian distributions on one hand, and a deterministic clustering procedure (DBSCAN) on the other. Here, two observations from the underlying species sampling mixture model share the same cluster if the distance between the densities corresponding to their latent parameters is smaller than a threshold. We complete this definition in order to define an equivalence relation among data labels. The resulting new random partition is coarser than the one induced by the species sampling mixture. Of course, since this procedure depends on the value of the threshold, we suggest a strategy to fix it. In addition, we discuss implementation and applications of the model to a simulated bivariate dataset from a mixture of two densities with a curved cluster, and to a dataset consisting of gene expression profiles measured at different times, known in literature as Yeast cell cycle data. Comparison with more standard clustering algorithm will be given. In both cases, the cluster estimates from our model turn out to be more effective. A primary application of our model is to the case of data from heavy tailed or curved clusters.
Perfect Simulation Involving Functionals of a Dirichlet Process
Journal of Computational and Graphical Statistics, 2002
Page 1. Perfect Simulation Involving Functionals of a Dirichlet Process Alessandra GUGLIELMI, Chr... more Page 1. Perfect Simulation Involving Functionals of a Dirichlet Process Alessandra GUGLIELMI, Chris C. HOLMES, and Stephen G. WALKER This article shows how to perform perfect simulation of a functional of a Dirichlet process ...
Computational Statistics & Data Analysis, 2010
We consider mixtures of parametric densities on the positive reals with a normalized generalized ... more We consider mixtures of parametric densities on the positive reals with a normalized generalized gamma process (Brix, 1999) as mixing measure. This class of mixtures encompasses the Dirichlet process mixture (DPM) model, but it is supposedly more flexible in the detection of clusters in the data. With an almost sure approximation of the posterior distribution of the mixing process we can run a Markov chain Monte Carlo algorithm to estimate linear and nonlinear functionals of the predictive distributions. The best-fitting mixing measure is found by minimizing a Bayes factor for parametric against non-parametric alternatives. We illustrate the method with simulated and hystorical data, finding a tradeoff between the best-fitting model and the correct identification of the number of components in the mixture.
Non-informative invariant priors yield peculiar marginals
Communications in Statistics - Theory and Methods, 1998
Among the different criteria which lead to non-informativeness, in our opinion the invariance of ... more Among the different criteria which lead to non-informativeness, in our opinion the invariance of a prior with respect to the action of a group is the most meaningful from a statistical point of view. In the cr-additive setting this invariance often yields improper distributions that we will not consider, not being coherent probabilities. For this reason, we adopt a finitely additive approach to properly evaluate some features of invariant priors and their consequences on the other elements - in particular the marginal - of the Bayesian paradigm.
MCMC estimation of the law of the mean of a Dirichlet process
Research Report, 2000
MCMC estimation of the law of the mean of a Dirichlet process Alessandra Guglielmi y and RichardT... more MCMC estimation of the law of the mean of a Dirichlet process Alessandra Guglielmi y and RichardTweedie z ... (2) then Feigin and Tweedie (1989) show that fPn; n 0g is a Markov chain whose invariant distribu-tion is the law of a Dirichlet process on (IR;B(IR)) with parameter . ...
Applied Mathematical Sciences, 2009
The paper deals with the approximation of the law of a random functional of a Dirichlet process u... more The paper deals with the approximation of the law of a random functional of a Dirichlet process using a finite number of its moments. In particular, three classes of approximation proceduresexpansions in series of orthonormal polynomials, the ...
Bernoulli, 2012
Measure-valued Markov chains have raised interest in Bayesian nonparametrics since the seminal pa... more Measure-valued Markov chains have raised interest in Bayesian nonparametrics since the seminal paper by (Math. Proc. Cambridge Philos. Soc. 105 (1989) 579-585) where a Markov chain having the law of the Dirichlet process as unique invariant measure has been introduced. In the present paper, we propose and investigate a new class of measure-valued Markov chains defined via exchangeable sequences of random variables. Asymptotic properties for this new class are derived and applications related to Bayesian nonparametric mixture modeling, and to a generalization of the Markov chain proposed by (Math. Proc. Cambridge Philos. Soc. 105 (1989) 579-585), are discussed. These results and their applications highlight once again the interplay between Bayesian nonparametrics and the theory of measure-valued Markov chains.
Uploads
Papers by Alessandra Guglielmi