Papers by Abdullah Baradaaji

ACM Transactions on Intelligent Systems and Technology
Recently, structured computing has become an interesting topic in the world of artificial intelli... more Recently, structured computing has become an interesting topic in the world of artificial intelligence, especially in the field of machine learning, as most researchers focus on the development of graph-based semi-supervised learning models. In this article, we present a new framework for graph-based semi-supervised learning. We present a powerful method for simultaneous label inference and linear transform estimation. The targeted linear transformation is used to obtain a discriminant subspace. To improve semi-supervised learning, our framework focuses on exploiting the data structure and soft labels of the available unlabeled samples. In the iterative optimization scheme used, the prior estimation of the label increases the supervision information indirectly through an introduced informative matrix called the label graph, thus avoiding the use of hard confidence-based decisions as used in self-supervised methods. In addition, the estimation of labels and projected data is made mor...
Information Sciences, 2021
This is a PDF file of an article that has undergone enhancements after acceptance, such as the ad... more This is a PDF file of an article that has undergone enhancements after acceptance, such as the addition of a cover page and metadata, and formatting for readability, but it is not yet the definitive version of record. This version will undergo additional copyediting, typesetting and review before it is published in its final form, but we are providing this version to give early visibility of the article. Please note that, during the production process, errors may be discovered which could affect the content, and all legal disclaimers that apply to the journal pertain.
2020 25th International Conference on Pattern Recognition (ICPR)
A unified semi-supervised model with joint estimation of graph, soft labels and latent subspace
Neural Networks
Simultaneous label inference and discriminant projection estimation through adaptive self-taught graphs
Expert Systems with Applications
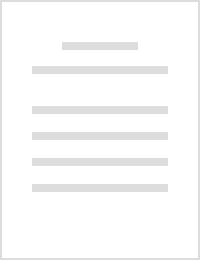
1999 - Soft Label and Discriminant Embedding Estimation for Semi-Supervised Classification
ICPR Browser Link: https://ailb-web.ing.unimore.it/icpr/paper/34/nn Abstract: In recent times, gr... more ICPR Browser Link: https://ailb-web.ing.unimore.it/icpr/paper/34/nn Abstract: In recent times, graph-based semi-supervised learning proved to be a powerful paradigm for processing and mining large datasets. The main advantage relies on the fact that these methods can be useful in propagating a small set of known labels to a large set of unlabeled data. The scarcity of labeled data may affect the performance of the semi-learning. This paper introduces a new semi-supervised framework for simultaneous linear feature extraction and label propagation. The proposed method simultaneously estimates a discriminant transformation and the unknown label by exploiting both labeled and unlabeled data. In addition, the unknowns of the learning model are estimated by integrating two types of graph-based smoothness constraints. The resulting semi-supervised model is expected to learn more discriminative information. Experiments are conducted on six public image datasets. These experimental results s...
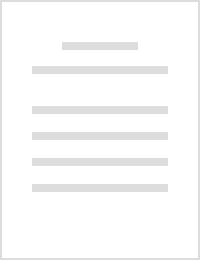
Joint Label Inference and Discriminant Embedding
IEEE transactions on neural networks and learning systems, 2021
Graph-based learning in semisupervised models provides an effective tool for modeling big data se... more Graph-based learning in semisupervised models provides an effective tool for modeling big data sets in high-dimensional spaces. It has been useful for propagating a small set of initial labels to a large set of unlabeled data. Thus, it meets the requirements of many emerging applications. However, in real-world applications, the scarcity of labeled data can negatively affect the performance of the semisupervised method. In this article, we present a new framework for semisupervised learning called joint label inference and discriminant embedding for soft label inference and linear feature extraction. The proposed criterion and its associated optimization algorithm take advantage of both labeled and unlabeled data samples in order to estimate the discriminant transformation. This type of criterion should allow learning more discriminant semisupervised models. Nine public image data sets are used in the experiments and method comparisons. These experimental results show that the perfo...
Uploads
Papers by Abdullah Baradaaji