Papers by Mostafa M. Fouda

IEEE access, 2024
In this paper, re-configurable intelligent reflecting surfaces (IRS) based on cell-free communica... more In this paper, re-configurable intelligent reflecting surfaces (IRS) based on cell-free communications to serve multi-user (MU) are considered. This is to enhance the transmission for the next generation of wireless communications. This technique has witnessed lots of interest recently due to its ability to increase diversity gain, especially in the presence of obstacles between the users and the service providers. The IRS contains low-cost and large-scale reflection elements that work passively to guide the electromagnetic waves toward the direction of interest. These re-configurable meta-surface cells have reflection coefficients that can be adjusted by changing their phase shift to enhance the desired signal of interest and apply interference mitigation. Moreover, the IRS can be exploited to improve the overall sum rate throughput and reduce outage probability. The proposed system considers a transmission between multiple base stations (BSs) that equipped with multiple antennas and several single antenna users through an IRS in the presence and absence of a traditional path between them. Optimization techniques are employed to select the optimum beamforming precoders and to control the IRS's phase shifts by steering the incident signals toward the intended users. Furthermore, an off-the-shelf power allocation optimization approach, called Polyhedron, is exploited to enhance the overall spectral efficiency (SE) and energy efficiency (EE) of the proposed system and reduce the required transmitted power. The proposed system with the suggested optimization approaches demonstrates significant improvement in the SE with a considerable reduction of the entire transmitted power by all BSs especially when increasing the number of antennas at the BSs along with using a higher number of IRS's reflected elements. Intelligent reflecting surfaces (IRS), cell-free (CF), Polyhedron optimization technique, Multiple-input single-output (MISO), Power allocation, Optimum beamforming, Multi-user communications, and Spectral efficiency (SE).

IEEE access, 2024
Malaria is a mosquito-borne, life-threatening, and contagious disease that has caused thousands o... more Malaria is a mosquito-borne, life-threatening, and contagious disease that has caused thousands of fatalities in recent years. Due to inadequate detection, the inexperience of laboratory personnel, and lack of advanced point-of-care equipment, the malaria-induced mortality rate is increasing. In addition to the traditional detection mechanisms, researchers have recently been investigating microscopic malariainfected Red Blood Cells (RBC) image analysis based on deep learning models to detect malaria parasites as a general-purpose point-of-care solution. In this paper, we develop several hybrid data-driven models by combining a convolutional neural network (CNN) to extract the relevant features and two cascaded recurrent neural networks ( ) classifiers. Gated recurrent unit (GRU), long short-term memory (LSTM), and Bi-directional LSTM (BiLSTM) are considered candidate RNN classifiers. The models are compared in terms of accuracy, type-I & II error rates, and inference & training computation time. Experimental results demonstrate that the CNN-LSTM-BiLSTM model outperforms the other models with a significantly higher accuracy (96.20%), less type-I error rate (2.23%), and fewer combined type-I and type-II errors (3.80%). Also, we consider the model computation time (both training time per epoch and inference time per step)' as an important metric for emerging distributed learning paradigms where point-of-care devices with IoT (Internet of Things) capability can jointly contribute to global model accuracy. Thus, our findings demonstrate the practicality of cascading classifiers in resource-constrained point-of-care devices.

IEEE Access, Dec 31, 2023
This paper provides a study of the latest target (object) detection algorithms for underwater wir... more This paper provides a study of the latest target (object) detection algorithms for underwater wireless sensor networks (UWSNs). To ensure selection of the latest and state-of-the-art algorithms, only algorithms developed in the last seven years are taken into account that are not entirely addressed by the existing surveys. These algorithms are classified based on their architecture and methodologies of operation and their applications are described that are helpful in their selection in a diverse set of applications. The merits and demerits of the algorithms are also addressed that are helpful to improve their performance in future investigation. Moreover, a comparative analysis of the described algorithms is also given that further provides an insight to their selection in various applications and future enhancement. A depiction of the addressed algorithms in various applications based on publication count over the latest decade (2023-2013) is also given using the IEEE database that is helpful in knowing their future application trend. Finally, the challenges associated with the underwater target detection are highlighted and the future research paradigms are identified. The conducted study is helpful in providing a thorough analysis of the underwater target detection algorithms, their feasibility in various applications with future challenges and defined strategies for further investigation. INDEX TERMS Underwater target detection, deep learning, underwater object detection, YOLO, convolutional neural networks, ConVNNs.
Vehicles communications handover in 5G: A survey
ICT Express, 2022
Dynamic Modeling and Control of Hybrid AC/DC Microgrid With Green Hydrogen Energy Storage
Energy Management and Techno-Economic Optimization of an Isolated Hybrid AC/DC Microgrid with Green Hydrogen Storage System
AI-Aided Height Optimization for NOMA-UAV Networks

arXiv (Cornell University), Nov 8, 2019
As we are about to embark upon the highly hyped "Society 5.0", powered by the Internet of Things ... more As we are about to embark upon the highly hyped "Society 5.0", powered by the Internet of Things (IoT), traditional ways to monitor human heart signals for tracking cardio-vascular conditions are challenging, particularly in remote healthcare settings. On the merits of low power consumption, portability, and non-intrusiveness, there are no suitable IoT solutions that can provide information comparable to the conventional Electrocardiography (ECG). In this paper, we propose an IoT device utilizing a spintronic-technology-based ultra-sensitive Magnetic Tunnel Junction (MTJ) sensor that measures the magnetic fields produced by cardio-vascular electromagnetic activity, i.e. Magentocardiography (MCG). We treat the low-frequency noise generated by the sensor, which is also a challenge for most other sensors dealing with low-frequency bio-magnetic signals. Instead of relying on generic signal processing techniques such as moving average, we employ deep-learning training on biomagnetic signals. Using an existing dataset of ECG records, MCG signals are synthesized. A unique deep learning model, composed of a one-dimensional convolution layer, Gated Recurrent Unit (GRU) layer, and a fully-connected neural layer, is trained using the labeled data moving through a striding window, which is able to smartly capture and eliminate the noise features. Simulation results are reported to evaluate the effectiveness of the proposed method that demonstrates encouraging performance.

IEEE Access, 2022
Recently, unmanned aerial vehicles (UAVs) communications gained significant concentration as a ta... more Recently, unmanned aerial vehicles (UAVs) communications gained significant concentration as a talented technology for future wireless communications using its remarkable advantages and broad applicability. Furthermore, UAV networks' high complex configurations and designs encourage researchers to leverage relevant artificial intelligence (AI) techniques for better beyond fifth-generation (B5G)/sixthgeneration (6G) services. This article summarizes AI-aided UAV solutions designated for forthcoming wireless networks. Besides, we deliver a comprehensive summary of machine learning (ML) approaches, including their applications and valuable contributions towards effective UAV network implementations, particularly advanced ML ones like bandits, federated learning (FL), meta-learning, etc. Finally, detailed UAV communication-related future research scopes and challenges is highlighted.

International Journal of Intelligent Systems, Jun 24, 2023
Pelvis fracture detection is vital for diagnosing patients and making treatment decisions for tra... more Pelvis fracture detection is vital for diagnosing patients and making treatment decisions for traumatic pelvis injuries. Computeraided diagnostic approaches have recently become popular for assisting doctors in disease diagnosis, making their conclusions more trustworthy and error-free. Inspecting X-ray images with fractures needs a lot of time from experienced physicians. However, there is a lack of inexperienced radiologists in many hospitals to deal with these images. Terefore, this study presents an accurate computer-aided-diagnosing system based on deep learning for detecting pelvis fractures. In this research, we construct an explainable artifcial intelligence (XAI) framework for pelvis fracture classifcation. We used a dataset containing 876 X-ray images (472 pelvis fractures and 404 normal images) to train the model. Te obtained results are 98.5%, 98.5%, 98.5%, and 98.5% for accuracy, sensitivity, specifcity, and precision.

arXiv (Cornell University), Dec 2, 2020
In the advanced metering infrastructure (AMI) network of the smart power grid, smart meters (SMs)... more In the advanced metering infrastructure (AMI) network of the smart power grid, smart meters (SMs) are installed at the customers' premises to report their fine-grained power consumption readings to the utility for billing and load monitoring purposes. Moreover, to create a clean power system, customers install solar panels on their rooftops to generate power and sell it to the utility. However, malicious customers may compromise their SMs to report false readings to achieve financial gains illegally. Reporting false readings not only causes hefty financial losses to the utility but may also degrade the grid performance because the reported readings are used for energy management. This paper is the first work that investigates this problem in the net-metering system, in which one SM is used to report the difference between the power consumed and the power generated. First, we prepare a benign dataset for the net-metering system by processing a real power consumption and generation dataset. Then, we propose a new set of attacks tailored for the net-metering system to create malicious dataset. After that, we analyze the data and we found time correlations between the net meter readings and correlations between the readings and relevant data obtained from trustworthy sources such as the solar irradiance and temperature. Based on the data analysis, we propose a general multi-data-source deep hybrid learning-based detector to identify the false-reading attacks. Our detector is trained on net meter readings of all customers besides data from the trustworthy sources to enhance the detector performance by learning the correlations between them. The rationale here is that although an attacker can report false readings, he cannot manipulate the solar irradiance and temperature values because they are beyond his control. Extensive experiments have been conducted, and the results indicate that our detector can identify the false-reading attacks with high detection rate and low false alarm.

Benha Journal of Applied Sciences, Mar 1, 2017
Massive multiple-input multiple-output (MIMO) is the technology of the future, because it has the... more Massive multiple-input multiple-output (MIMO) is the technology of the future, because it has the capability to increase a wireless system capacity without costing the system to purchase an additional bandwidth. It also has the capability to decrease the energy consumption of the system. All these capabilities have promoted the massive MIMO to be a promising technology for the fifth generation (5G) wireless communication systems. However, it has an important issue that limits the benefits which can be acquired by deploying it in 5G. This issue is the pilot contamination, which means the interference between pilot sequences. This occurs when the same pilot sequence is used by more than one terminal in the channel estimation phase at the same time and this causes interference between data symbols later. In this paper, we propose an enhanced fractional frequency reuse (FFR) scenario to mitigate the effects of pilot contamination by following an algorithm in assigning the pilot sequences to the system terminals instead of the random assignment. Simulation results show that our proposed scenario outperforms the traditional FFR in terms of signal to interference and noise ratio (SINR) and capacity.
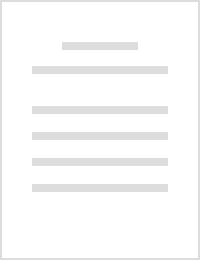
A spatiotemporal scenario to mitigate pilot contamination in 5G massive MIMO systems
Massive multiple-input multiple-output (MIMO) or large-scale MIMO is the magical technology that ... more Massive multiple-input multiple-output (MIMO) or large-scale MIMO is the magical technology that can provide the required large system capacity in the future fifth generation (5G) cellular networks. Massive MIMO is characterized by a large number of base station (BS) antennas, which makes it less susceptible to noise and fast fading. Unfortunately, this large antennas number does not enable the massive MIMO to get rid of the inter-cell interference originated from the problem of pilot contamination. In this article, we propose a spatiotemporal scenario that can mitigate the pilot contamination effects by avoiding synchronous pilot transmission in the time domain and following a pilot allocation algorithm in the spatial domain. We show that our proposed scenario provides a reduced level of inter-cell interference, which eventually gives it the ability to enhance the minimum and the mean achievable capacities per terminal.
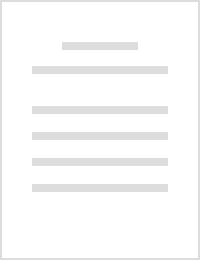
A novel vision to mitigate pilot contamination in massive MIMO-based 5G networks
Massive multiple-input multiple-output (MIMO) is one of the promising technologies to be used in ... more Massive multiple-input multiple-output (MIMO) is one of the promising technologies to be used in the future fifth generation (5G) wireless networks for its capability to increase the system throughput, improve spectral efficiency and energy efficiency. To achieve these benefits of the massive MIMO, there are many challenges that need to be handled. One of these challenges is the pilot contamination problem that arises from reusing pilot sequences among the system cells. Since this problem is considered as a bottleneck of the system performance, it attracted many researchers who proposed schemes to solve it. In this paper, we propose a new scheme that mitigates its effect by adopting both asynchronous pilot transmission (APT) and fractional pilot reuse (FPR). We compare the performance of our proposed scheme with other schemes using computer simulations and show that our proposed scheme enhances the performance of the time division duplex (TDD) multi-cell massive MIMO systems in terms of the signal to interference and noise ratio (SINR) and capacity.

IEEE Access, 2022
Visible light communication (VLC) is a relatively new wireless communication technology that allo... more Visible light communication (VLC) is a relatively new wireless communication technology that allows for high data rate transfer. Because of its capability to enable high-speed transmission and eliminate inter-symbol interference, orthogonal frequency division multiplexing (OFDM) is widely employed in VLC. Peak to average power ratio (PAPR) is an issue that impacts the effectiveness of OFDM systems, particularly in VLC systems, because the signal is distorted by the nonlinearity of light-emitting diodes (LEDs). The proposed method Long Short Term Memory-Autoencoder (LSTM-AE) uses an autoencoder as well as an LSTM to learn a compact representation of an input, allowing the model to handle variable length input sequences as well as predict or produce variable length output sequences. This study compares the suggested model with various PAPR reduction strategies to demonstrate that it offers a superior improvement in PAPR reduction of the transmitted signal while maintaining BER. Also, this model provides a flexible compromisation between PAPR and BER.

IEEE Access, 2022
Worldwide, Nuclear Power Plants (NPPs) must have higher security protection and precise fault det... more Worldwide, Nuclear Power Plants (NPPs) must have higher security protection and precise fault detection systems, especially underground power cable faults, to avoid causing national disasters and keep on safe national ratios of electricity production. Hence, this paper proposes an automatic, effective, and accurate Deep Learning (DL)-based fault classification and location technique for these cables via a One-dimensional Convolutional Neural Network (1D-CNN) and a Binary Support Vector Machine (BSVM). The proposed approach includes four main steps: data collection, feature extraction and reduction, fault detection, and fault classification and location. Signal collection from the underground cable's sending end is performed via the Alternating Transient Program/Electromagnetic Transient Program (ATP/EMTP). Feature extraction and reduction are performed via Fractional Discrete Cosine Transform (FrDCT) and Singular Value Decomposition (SVD) methods. Fault detection is performed through leveraging BSVM with the linear Kernel method in the third step. Finally, this permits 1D-CNN to classify the fault type and locate it. Simulation results confirmed the efficiency of our proposed method, especially for 11kV underground cable faults, including different fault resistances and inception angles. Moreover, the proposed technique is applicable in real-time scenarios with a 99.6% accuracy rate, 0.15sec lowest execution time, and 0.095% maximum error rate for fault location at fractional factor (α) equals to 0.8.

IEEE Access
This paper surveys the short-term road traffic forecast algorithms based on the long-short term m... more This paper surveys the short-term road traffic forecast algorithms based on the long-short term memory (LSTM) model of deep learning. The algorithms developed in the last three years are studied and analyzed. This provides an in-depth and thorough description of the algorithms rather than their marginal description as performed in the existing surveys that focus on general deep learning algorithms. The chosen algorithms are classified depending upon the use of LSTM in combination with other techniques for processing input data features towards a final traffic forecast. The operational strategies of the algorithms are described with merits and limitations. Moreover, a comparative analysis of the compared classes of algorithms is also provided. These strategies are helpful in selection of the right algorithms and their classes for the diverse traffic conditions and their future investigation for improvement. Besides, the applications of these classes of algorithms to traffic forecast in various networks for the latest decade is graphically depicted. Moreover, the applications of the LSTM in other fields involving a forecast are provided. Finally, the challenges associated with the short-term traffic forecast using the LSTM are described and strategies are highlighted for their future investigation.
Energies
This Special Issue on “Secure and Efficient Communication in Smart Grids” received a total of 11 ... more This Special Issue on “Secure and Efficient Communication in Smart Grids” received a total of 11 submitted articles, of which 5 were accepted and published after each passing an independent peer-review process [...]

Drones
In this work, we design an intelligent reflecting surface (IRS)-assisted Internet of Things (IoT)... more In this work, we design an intelligent reflecting surface (IRS)-assisted Internet of Things (IoT) by enabling non-orthogonal multiple access (NOMA) and unmanned aerial vehicles (UAV) approaches. We pay attention to studying the achievable rates for the ground users. A practical system model takes into account the presence of hardware impairment when Rayleigh and Rician channels are adopted for the IRS–NOMA–UAV system. Our main findings are presented to showcase the exact expressions for achievable rates, and then we derive their simple approximations for a more insightful performance evaluation. The validity of these approximations is demonstrated using extensive Monte Carlo simulations. We confirm the achievable rate improvement decided by main parameters such as the average signal to noise ratio at source, the position of IRS with respect to the source and destination and the number of IRS elements. As a suggestion for the deployment of a low-cost IoT system, the double-IRS model ...

Energies
An earthquake early warning system (EEWS) should be included in smart cities to preserve human li... more An earthquake early warning system (EEWS) should be included in smart cities to preserve human lives by providing a reliable and efficient disaster management system. This system can alter how different entities communicate with one another using an Internet of Things (IoT) network where observed data are handled based on machine learning (ML) technology. On one hand, IoT is employed in observing the different measures of EEWS entities. On the other hand, ML can be exploited to analyze these measures to reach the best action to be taken for disaster management and risk mitigation in smart cities. This paper provides a survey on the different aspects required for that EEWS. First, the IoT system is generally discussed to provide the role it can play for EEWS. Second, ML models are classified into linear and non-linear ones. Third, the evaluation metrics of ML models are addressed by focusing on seismology. Fourth, this paper exhibits a taxonomy that includes the emerging ML and IoT e...
Uploads
Papers by Mostafa M. Fouda