Papers by Pruthvi Manjunatha
Simulation Platform for Testing and Evaluation of CAV Trajectory Optimization and Signal Control Algorithm Integrated with Commercial Traffic Simulator
2022 Winter Simulation Conference (WSC)
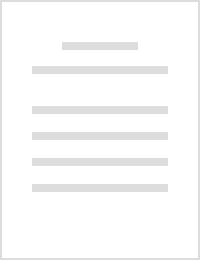
Evaluation of Arterial Corridor Improvements and Traffic Management Plans in Florida
University of Florida. Transportation Institute, Sep 1, 2019
US Transportation Collection2019PDFTech ReportElefteriadou, LilyManjunatha, PruthviHartley, TyDua... more US Transportation Collection2019PDFTech ReportElefteriadou, LilyManjunatha, PruthviHartley, TyDuan, XiUniversity of Florida. Transportation InstituteUniversity of Florida. Transportation InstituteFloridaUnited StatesAdaptive controlAlternatives analysisArterial highwaysEvaluation and assessmentPeriods of the dayTraffic delaysTraffic queuingTraffic signal control systemsTravel timeAdaptive Signal Control Technologies (ASCT)MobilitySafetyBDV31-977-44Final Report, Jun 2015 \u2013 Sep 2019The main goal of adaptive signal control technologies (ASCT) is to minimize the travel time experienced by travelers and to reduce the number of stops through arterial corridors. This project conducted an independent assessment of an ASCT system in Mayport, FL, and compared operating conditions when the system was \u201cOff\u201d to when the system was \u201cOn\u201d to determine its effectiveness. The mobility benefits of the ASCT implementation were assessed by comparing performance measures of time of the day (TOD) plans versus ASCT through field data collection. Two critical intersections were identified within the corridor, and performance measures such as corridor travel time, intersection delay, major and minor street queues, turning movement, etc. were collected. The changes in performance measures were used to report the effectiveness of ASCT.96
Evaluation of Advanced & Communication Technologies Through Traffic Microsimulation
Southeastern Transportation Research, Innovation, Development and Education Center (STRIDE), Aug 2, 2021
Simulation results
The folder contains results from the CAV simulation for STRIDE project D.
An Examination of Two Diverse Communities: Residents' Transportation Behaviors, Challenges, and Opportunities
Otjr-occupation Participation and Health, Apr 27, 2023
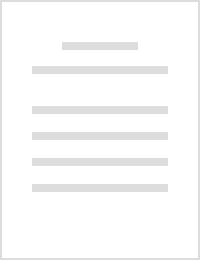
Evaluation of the Operational Effects of Autonomous and Connected Vehicles through Microsimulation
Transportation Research Record: Journal of the Transportation Research Board
Proper evaluation of traffic operations integrating connected and autonomous vehicles (CAVs) requ... more Proper evaluation of traffic operations integrating connected and autonomous vehicles (CAVs) requires accurate representation of these emerging technologies in microscopic simulation. This paper evaluates the ability of microscopic simulator PTV-VISSIM (Version 10.0) to simulate CAVs, and presents a comprehensive CAV model extension. In addition, emissions modeling is integrated with VISSIM to calculate real-time energy and emission estimates. The evaluation of VISSIM revealed that its internal CAV modeling has several limitations, such as modeling connectivity and complex vehicle behavior. For external modeling, there are two available VISSIM interfaces. The Component Object Model (COM) Application Programming Interface (API) is the superior approach for fetching data and modeling connectivity, whereas the External Driver Model (EDM) is a better tool for lateral and longitudinal control. The simulation extension developed leveraged both interfaces. An isolated signalized intersecti...
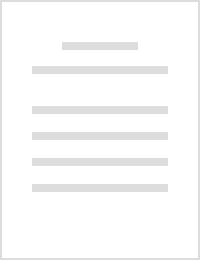
Methodology for the Calibration of VISSIM in Mixed Traffic
Mixed traffic, characterized by diverse vehicles, changing composition, lack of lane discipline, ... more Mixed traffic, characterized by diverse vehicles, changing composition, lack of lane discipline, etc. is best modeled by micro simulation. However, the majority of the leading micro simulation packages and their calibration methodologies have been developed considering less complex homogeneous traffic. Hence, a methodology for calibrating a micro simulation model for mixed traffic is proposed. Driver behavior in mixed traffic is observed and adjustments were made to represent in the simulation. Calibration parameters were identified using multi parameter sensitivity analysis, and the optimum values for these parameters were obtained by minimizing the error between the simulated and field delay using a genetic algorithm. Multiple criteria were included in the optimization formulation by constraint insertion. The proposed methodology is illustrated using VISSIM, a widely used micro simulation software. Signalized intersections with different traffic characteristics from Mumbai are tak...
Empirical Assessment of Adaptive Signal Control Technologies in Florida
Analysis of Wiedemann Car Following Thresholds Using Driving Simulator Observations: (Extended Abstract Only)
How Has Driver Behavior Been Considered in Traffic Microsimulation and How Can we Use Cognitive Sciences and Psychology Studies to Enhance Them

Mixed traffic, characterized by diverse vehicles, changing composition, lack of lane discipline, ... more Mixed traffic, characterized by diverse vehicles, changing composition, lack of lane discipline, etc. is best modeled by micro simulation. However, the majority of the leading micro simulation packages and their calibration methodologies have been developed considering less complex homogeneous traffic. Hence, a methodology for calibrating a micro simulation model for mixed traffic is proposed. Driver behavior in mixed traffic is observed and adjustments were made to represent in the simulation. Calibration parameters were identified using multi parameter sensitivity analysis, and the optimum values for these parameters were obtained by minimizing the error between the simulated and field delay using a genetic algorithm. Multiple criteria were included in the optimization formulation by constraint insertion. The proposed methodology is illustrated using VISSIM, a widely used micro simulation software. Signalized intersections with different traffic characteristics from Mumbai are tak...
Conference Presentations by Pruthvi Manjunatha

98th Annual Meeting of the Transportation Research Board, 2019
Human factors have significant influence over traffic operations. To understand situations such a... more Human factors have significant influence over traffic operations. To understand situations such as traffic breakdowns, capacity drop, etc., it is essential that human factors are measured and modeled accurately. While existing microsimulation models use stochastic distributions to represent human variability, there is lack of literature in traffic engineering that links these distributions to specific driver behaviors.
At the same time, considerable work has been reported in the field of psychology on modeling and predicting human behavior and drivers’ intended actions through driver cognitive states represented by constructs such as Workload (WL) and Situational Awareness (SA).
We present a framework for incorporating human factors in traffic microsimulation. “Driver States” such as WL, SA are the cognitive condition of drivers that can change dynamically over time and space depending on the internal emotional factors and external environmental factors. We propose that changes in Driver Behavior (Car Following (CF), Lane Changing (LC)) is the resultant physical manifestation of drivers’ cognitive response to changes in Driver States.
A driving simulator experiment was devised to measure Driver States (WL, SA) and Driver Behavior (CF, LC) concurrently for different traffic densities and at different freeway segments. Based on the driver cognitive states measured during a driving simulator experiment with a set of forty drivers, groups of drivers were identified using cluster analysis. When the driving trajectories were analyzed, conforming to the basis of our framework, the driver groups identified showed distinctly different driving behavior. The results of the work can be used to improve microsimulation models.
Uploads
Papers by Pruthvi Manjunatha
Conference Presentations by Pruthvi Manjunatha
At the same time, considerable work has been reported in the field of psychology on modeling and predicting human behavior and drivers’ intended actions through driver cognitive states represented by constructs such as Workload (WL) and Situational Awareness (SA).
We present a framework for incorporating human factors in traffic microsimulation. “Driver States” such as WL, SA are the cognitive condition of drivers that can change dynamically over time and space depending on the internal emotional factors and external environmental factors. We propose that changes in Driver Behavior (Car Following (CF), Lane Changing (LC)) is the resultant physical manifestation of drivers’ cognitive response to changes in Driver States.
A driving simulator experiment was devised to measure Driver States (WL, SA) and Driver Behavior (CF, LC) concurrently for different traffic densities and at different freeway segments. Based on the driver cognitive states measured during a driving simulator experiment with a set of forty drivers, groups of drivers were identified using cluster analysis. When the driving trajectories were analyzed, conforming to the basis of our framework, the driver groups identified showed distinctly different driving behavior. The results of the work can be used to improve microsimulation models.
At the same time, considerable work has been reported in the field of psychology on modeling and predicting human behavior and drivers’ intended actions through driver cognitive states represented by constructs such as Workload (WL) and Situational Awareness (SA).
We present a framework for incorporating human factors in traffic microsimulation. “Driver States” such as WL, SA are the cognitive condition of drivers that can change dynamically over time and space depending on the internal emotional factors and external environmental factors. We propose that changes in Driver Behavior (Car Following (CF), Lane Changing (LC)) is the resultant physical manifestation of drivers’ cognitive response to changes in Driver States.
A driving simulator experiment was devised to measure Driver States (WL, SA) and Driver Behavior (CF, LC) concurrently for different traffic densities and at different freeway segments. Based on the driver cognitive states measured during a driving simulator experiment with a set of forty drivers, groups of drivers were identified using cluster analysis. When the driving trajectories were analyzed, conforming to the basis of our framework, the driver groups identified showed distinctly different driving behavior. The results of the work can be used to improve microsimulation models.