Papers by Djamel Bouchaffra
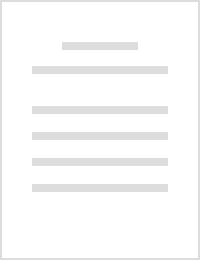
Postprocessing of Recognized Strings Using
This paper presents Nonstationary Markovian Models and their application to recognition of string... more This paper presents Nonstationary Markovian Models and their application to recognition of strings of tokens. Domain specific knowledge is brought to bear on the application of recognizing zip Codes in the U.S. mailstream by the use of postal directory files. These files provide a wealth of information on the delivery points (mailstops) corresponding to each zip code. This data feeds into the models as n-grams, statistics that are seamlessly integrated with recognition scores of digit images. An especially interesting facet of the model is its ability to excite and inhibit certain positions in the n-grams leading to the familiar area of Markov Random Fields. The authors have previously described elsewhere (2) a methodology for deriving probability values from recognizer scores. These probability measures allow the Markov chain to be constructed in a truly Bayesian framework. We empirically illustrate the success of Markovian modeling in postprocessing applications of string recognition. We present the recognition accuracy of the different models on a set of 20,000 zip codes. The performance is superior to the present system which ignores all contextual information and simply relies on the recognition scores of the digit recognizers. Index Terms—Nonstationary hidden Markov models, zip code recognition, postprocessing, class conditional probability, Markov random fields.
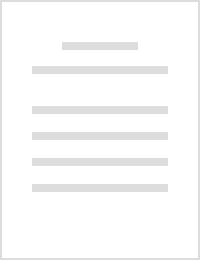
Towards Building an Emotional Speech Corpus of Algerian Dialect: Criteria and Preliminary Assessment Results
2019 International Conference on Advanced Electrical Engineering (ICAEE), Nov 1, 2019
This paper presents the preliminary results of an ongoing project whose mission is to build the f... more This paper presents the preliminary results of an ongoing project whose mission is to build the first acted Emotional Speech Corpus of Algerian Dialect named ESCAD. The corpus is being recorded by nonprofessional native speakers to simulate four emotional states, namely: (i) neutral, (ii) angry, (iii) happy, and (iv) disgust. We introduce the first version of the corpus that is limited to angry and neutral states. A group of 53 male and female speakers participated in the recording of the first version of the corpus. The purpose of this contribution consists of (i) outlining the criteria for building acted corpora, (ii) introducing the framework of ESCAD, and (iii) identifying unskilled speakers. The recorded corpus has been assessed using subjective test lead by 11 referees. An objective test for data separability has also been conducted. The obtained results are exploited thereafter to identify unskilled speakers among the initial volunteers. These speakers will not be requested to participate in the recording of the remaining emotions. The recorded data can be shared upon request.
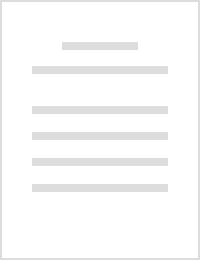
Induced Subgraph Game for Ensemble Selection
Ensemble methodology has proved to be one of the strongest machine learning techniques. In spite ... more Ensemble methodology has proved to be one of the strongest machine learning techniques. In spite of its huge success, most ensemble methods tend to generate unnecessary large number of classifiers, which entails an increase in memory storage, computational cost, and even a reduction of the generalization performance of the ensemble. Ensemble selection addresses these shortcomings by searching for a fraction of individual classifiers that performs as good as, or better than the entire ensemble. In this paper, we formulate the problem of ensemble selection as a coalitional game in a graph form, and name the approach ISCG-Ranking. The proposed game aims at capturing two crucial concepts that affect the performance of an ensemble: accuracy and diversity. Most importantly, it ranks every classifier based on its contribution in keeping a proper balance between these two notions using Shapley value. To demonstrate the validity and the effectiveness of the proposed approach, we carried out experimental comparisons with several state-of-the-art techniques on 26 UCI benchmark datasets. The results reveal that ISCG-Ranking significantly improves the original ensemble and performs better than other methods in most cases.
Embedding HMM’s-based models in a Euclidean space: The topological hidden Markov models
International Conference on Pattern Recognition, Dec 1, 2008
One of the major limitations of HMM-based models is the inability to cope with topology: When app... more One of the major limitations of HMM-based models is the inability to cope with topology: When applied to a visible observation (VO) sequence, HMM-based techniques have difficulty predicting the n-dimensional shape formed by the symbols of the VO sequence. To fulfill this need, we propose a novel paradigm named "topological hidden Markov models" (THMM's) that classifies VO sequences by embedding
Long Short-Term Memory Neural Network, Bottleneck Distance, and Their Combination for Topological Facial Expression Recognition
CRC Press eBooks, Dec 19, 2022
A Comparative Performance Study of Feature Selection Techniques for the Detection of Parkinson's Disease from Speech
CRC Press eBooks, Dec 19, 2022
Lombard effect in Algerian Dialect Speech: Investigation of boosting and bypass strategies in narrowband noisy environment
Applied Acoustics
Investigations of the potential of acoustic speech regions for detecting hostile talking in Algerian Dialect and German language
Applied Acoustics
IMECS 2011 - International MultiConference of Engineers and Computer Scientists 2011: Preface
International MultiConference of Engineers and Computer Scientists, Jul 26, 2011
ABSTRACT Export Date: 29 August 2012, Source: Scopus
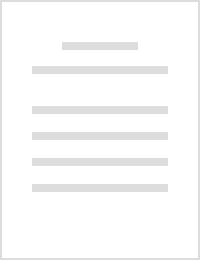
Persistent Homology for Land Cover Change Detection
Oxford Research Encyclopedia of Natural Hazard Science, Feb 23, 2021
The need for environmental protection, monitoring, and security is increasing, and land cover cha... more The need for environmental protection, monitoring, and security is increasing, and land cover change detection (LCCD) can aid in the valuation of burned areas, the study of shifting cultivation, the monitoring of pollution, the assessment of deforestation, and the analysis of desertification, urban growth, and climate change. Because of the imminent need and the availability of data repositories, numerous mathematical models have been devised for change detection. Given a sample of remotely sensed images from the same region acquired at different dates, the models investigate if a region has undergone change. Even if there is no substantial advantage to using pixel-based classification over object-based classification, a pixel-based change detection approach is often adopted. A pixel can encompass a large region, and it is imperative to determine whether this pixel (input) has changed or not. A changed image is compared to the available ground truth image for pixel-based performance evaluation. Some existing change detection systems do not take into account reversible changes due to seasonal weather effects. In other words, when snow falls in a region, the land cover is not considered as a change because it is seasonal (reversible). Some approaches exploit time series of Landsat images, which are based on the Normalized Difference Vegetation Index technique. Others evaluate built-up expansion to assess urban morphology changes using an unsupervised approach that relies on labels clustering. Change detection methods have also been applied to the field of disaster management using object-oriented image classification. Some methodologies are based on spectral mixture analysis. Other techniques invoke a similarity measure based on the evolution of the local statistics of the image between two dates for vegetation LCCD. Probabilistic approaches based on maximum entropy have been applied to vegetation and forest areas, such as Hustai National Park in Mongolia. Researchers in this field have proposed an LCCD scheme based on a feed-forward neural network using backpropagation for training. This paper invokes the new concept of homology theory, a subfield of algebraic topology. Homology theory is incorporated within a Structural Hidden Markov Model.
Feature Extraction Based on Wavelet Transform for Classification of Stress Level
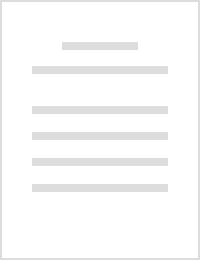
Land Cover Change Detection based on Homology Theory
2019 6th International Conference on Image and Signal Processing and their Applications (ISPA), 2019
We exploit homology theory (HT) for land cover change detection in remote sensing. Shape disclosu... more We exploit homology theory (HT) for land cover change detection in remote sensing. Shape disclosure is achieved through the Vietoris-Rips simplicial complex constructor. We propose to use persistent homology to overcome the land cover change detection problem in remote sensing. Qualitative Features (Betti numbers) are computed from a series of simplicial complexes at each level of resolution. Local structures are unfolded gradually throughout the observation sequence generated from a pixel in the input image at two different points in time. The weighted Bottleneck distance is used to detect the change satellite images. Experimental results demonstrate the efficiency of our methodology when compared to some major state-of-the-art approaches.

The monitoring of dangerous audio events is very important in surveillance systems. One of the mo... more The monitoring of dangerous audio events is very important in surveillance systems. One of the most significant phase in audio surveillance is the detection of impulsive sounds (IS). It is considered as a preprocessing stage prior to the recognition phase. We propose in this paper an indoor audio monitoring software to detect IS in real-time. It is composed of three main stages: (i) audio acquisition, (ii) preprocessing module and (iii) sound detector. We have used MEMS microphone to acquire the audio data. The preprocessing stage aims at tuning the microphone sensitivity. It is used to mask the non-desired frequency components of the environment by adding white noise. The detection of IS is conducted using a thresholding scheme based on normalized form of power sequences. The proposed prototype is running under Windows 7 on an ordinary laptop. The results we have obtained are very promising.
Abstract—This paper presents an evaluation of three classi-fiers using the discrete wavelet trans... more Abstract—This paper presents an evaluation of three classi-fiers using the discrete wavelet transform (DWT) as a feature extractor. The thrust is to investigate the impact of DWT with its various filter banks on the HMM, PCA and SHMM classifiers. In addtion, we have developed a novel approach that combines the multiresolution feature of the discrete wavelet transform with the local interactions of the facial structures expressed through the structural hidden Markov model (SHMM). Tests have been carried out on the AT&T and Essex face databases, which show that DWT/SHMM outperforms both the DWT/HMM and DWT/PCA with an 8 % increase in accuracy. I.
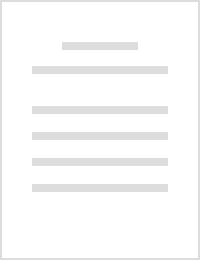
Because the need to classify objects with respect to uncertain properties is increasing, it becom... more Because the need to classify objects with respect to uncertain properties is increasing, it becomes important to seek an appropriate generalization of a Galois lattice structure. We aim to analyze an uncertain (probabilistic) Galois lattice structure. One of our interests consists to determine a complete (total) order within the objects set generated by the uncertain Galois lattice structure. Afterwards, we classify the objects according to the generation power criterion. This classification is set up using a Markov Mesh (MM) model when considering the uncertain Galois lattice as a neighborhood system. As an application,we hierarchically classify textual fragments according to their lexical properties. This operation produces a set of structured contexts (or fragments) that enables us to weight and find out the presence of different contexts of use assigned to each term. This set of contexts may aid the terminologist to capture the meaning of the terms studied.
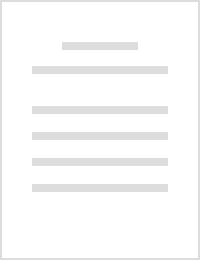
A k-Nearest Neighbor approach to improve change detection from remote sensing: Application to optical aerial images
2015 15th International Conference on Intelligent Systems Design and Applications (ISDA), 2015
This paper proposes a k-Nearest Neighbor (k-NN) based scheme in order to update a change detectio... more This paper proposes a k-Nearest Neighbor (k-NN) based scheme in order to update a change detection decision from a Feed-Forward Neural Network (FFNN). Change and no-change detection is treated as a context-free binary classification problem, using a FFNN fed by pixel spectral intensity data. The particularity of this method, compared to the existing and established ones, is that it takes into account the result of change/no-change decision of neighboring pixels during the detection process. A first stage FFNN pixel-based classification is conducted and the output change/no-change label assigned to a pixel is updated via information from neighboring pixel labels. A majority vote strategy is adopted within the k-nearest neighbors' labels. Experiments are performed on real optical aerial images with large time differences. We show that the proposed system produces an overall performance detection improvement of around 13% and 4% of F-measure and G-mean values, respectively, over the FFNN baseline system.
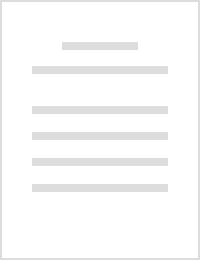
Selection of Optimal Sub-ensembles of Classifiers through Evolutionary Game Theory
2019 IEEE International Conference on Systems, Man and Cybernetics (SMC)
Ensemble methodology combines multiple learning schemes in order to improve the classification pe... more Ensemble methodology combines multiple learning schemes in order to improve the classification performance. An ensemble made of a large number of classifiers entails an increase in the computational cost, memory storage, and even a reduction in the predictive performance. Ensemble pruning has become an important task that lives up to these challenges. The thrust consists of constructing a subset that maintains or improves the accuracy of the original set of classifiers while reducing the number of members that constitute the ensemble. Inspired by evolutionary game theory, we formulate ensemble pruning as a dynamical system. Our approach simulates the evolution of an ensemble according to the replicator dynamics. The selection mechanism is defined based on strategic interactions that favor complementary members. The survivors of the evolutionary process compose the final ensemble. In order to evaluate the proposed technique, we performed comparisons with some major state-of-the-art methods such as semi-definite programming, genetic algorithm, and orientation ordering, based on 30 UCI benchmark datasets. The results demonstrate the effectiveness of our approach in terms of accuracy performance, pruning ratio, and computational cost.
Echantillonnage multivarié de textes pour les processus de Markov et introduction au raisonnement incertain dans le traitement de la langue naturelle
ABSTRACT
Editorial: Feature generation and machine learning for robust multimodal biometrics
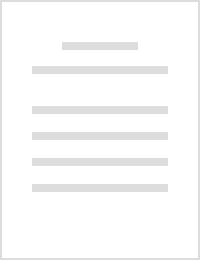
Structural hidden Markov models: An application to handwritten numeral recognition
Intelligent Data Analysis
ABSTRACT We introduce in this paper a generalization of the widely used hidden Markov models (HMM... more ABSTRACT We introduce in this paper a generalization of the widely used hidden Markov models (HMM's), which we name "structural hidden Markov models" (SHMM). Our approach is motivated by the need of modeling complex structures which are encountered in many natural sequences pertaining to areas such as computational molecular biology, speech/handwriting recognition and content-based information retrieval. We consider observations as strings that produce the structures derived by an unsupervised learning process. These observations are related in the sense they all contribute to produce a particular structure. Four basic problems are assigned to a structural hidden Markov model: (1) probability evaluation, (2) state decoding, (3) structural decoding, and (4) parameter re-estimation. We have applied our methodology to recognize handwritten numerals. The results reported in this application show that the structural hidden Markov model outperforms the traditional hidden Markov model with a 23.9% error-rate reduction.
Uploads
Papers by Djamel Bouchaffra