Papers by Vikramrajkumar Thiyagarajan
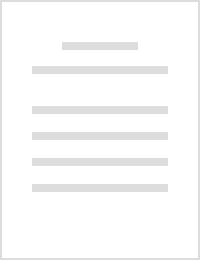
Reinforcement Learning for Financial Consolidation: A2C Model with Sentiment and Temporal Data
IEEE, 2024
Financial markets present inherent challenges due to their complex, non-linear dynamics and susce... more Financial markets present inherent challenges due to their complex, non-linear dynamics and susceptibility to various external factors, such as macroeconomic indicators, investor sentiment, and market volatility. Traditional stock trading models, relying heavily on historical price data, often fall short in capturing the complexities of market behavior. This paper proposes an advanced financial trading system based on the Advantage Actor-Critic (A2C) reinforcement learning framework. The model integrates Long Short-Term Memory (LSTM) networks to capture temporal dependencies in stock prices, as well as sentiment analysis to incorporate market psychology into trading decisions. Combining these elements, the model optimizes cumulative portfolio returns and effectively manages risk, as evidenced by enhanced Sharpe ratios and reduced volatility. A special reward function with built-in volatility penalties and entropy regularization also helps make sure that decisions are fair by encouraging exploration and stopping solutions that aren't the best. Numerous tests demonstrate that the proposed model outperforms the standard Deep Q-Network (DQN) and Proximal Policy Optimization (PPO) approaches in numerous financial domains. These results show that combining advanced reinforcement learning models with outside data sources could help people make better decisions in real-life financial markets. This implies that tasks such as combining finances, creating budgets, making predictions, and integrating ERP systems could utilize the model.

www.espjournals.org, 2023
In the current dynamic business environment, the use of AI as part of BI has emerged as a key det... more In the current dynamic business environment, the use of AI as part of BI has emerged as a key determinant of the competitiveness of a firm. This paper is aimed at discussing the possibilities of using AI for the modernization of traditional BI models to support more efficient data analysis, better forecasting, and faster decision-making in organizations. Reviewing the possibilities of AI in BI with a focus on the most essential technologies like machine learning, natural language processing, and predictive analytics, the results of this research demonstrate how the companies might improve operational performance, customer satisfaction, the overall organization's effectiveness implementing AI strategies as a part of BI initiatives. Finally, by the use of case studies and real-life BI applications, we show how radical AI has been in enhancing BI and how it can be implemented in organizations that want to achieve lasting growth and innovation.
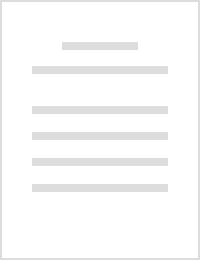
Enhanced Cancer Prediction Using Fine-Tuned CatBoost Model
IEEE, 2024
Cancer prediction using machine learning (ML) models has gained significant attention due to its ... more Cancer prediction using machine learning (ML) models has gained significant attention due to its potential to improve early diagnosis and treatment outcomes. Current models often face challenges such as imbalanced datasets, inadequate feature selection methods, and suboptimal hyperparameter tuning, limiting
their predictive accuracy and clinical applicability. To fill in these gaps, this study proposes a fine-tuned CatBoost model that is best for predicting cancer. It uses advanced data processing methods, such as ANOVA-based feature selection, to make sure that the input features are relevant. The proposed study is benchmarked
against multiple state-of-the-art ML models, such as Random Forest, XGBoost, and AdaBoost, providing a comprehensive performance comparison. By applying meticulous hyperparameter tuning, specifically optimizing parameters like learning
rate, depth, and iterations, the CatBoost model significantly improves accuracy, precision, recall, and AUC scores. Results indicate a 0.93 accuracy with a 3% to 5% improvement over traditional models like decision trees and K-nearest neighbors.
The CatBoost model exhibits enhanced interpretability through feature importance analysis, making it highly applicable in clinical settings. The fine-tuned CatBoost achieves a 4% increase in precision and a 6% increase in recall compared to baseline
models. This demonstrates its robustness and versatility across various datasets. The findings suggest that the proposed approach bridges the identified gaps and offers a reliable, high-performing solution for cancer prediction, outperforming existing models in terms of predictive power and clinical utility.
International Journal on Recent and Innovation Trends in Computing and Communication, 2020
This paper presents recent progress in machine learning techniques to extend integration between ... more This paper presents recent progress in machine learning techniques to extend integration between Oracle Enterprise Performance Management (EPM) and Enterprise Resource Planning (ERP) systems. Based on an extensive review of existing challenges in the current integration and newly developed technologies within ML, it proposes new strategies in automated data mapping and intelligent workflow orchestration and predictive analytics. Our findings clearly reflect better consistency in the process of integration for efficiency, precision, and scalability by implementing machine learning algorithms in schema matching, anomaly detection, and natural language processing related to data query operations.
International Journal on Recent and Innovation Trends in Computing and Communication, 2021
This research paper explores the integration of Artificial Intelligence (AI) and Machine Learning... more This research paper explores the integration of Artificial Intelligence (AI) and Machine Learning (ML) models into Oracle Planning and Budgeting Cloud (PBCS) system to enhance forecasting accuracy and optimize scenario planning. The study investigates how predictive analytics and real-time data processing can be leveraged to automate and improve financial planning processes. Through a comprehensive analysis of current methodologies and emerging AI technologies, this paper aims to bridge the research gap in understanding AI's impact on forecasting reliability, particularly in fluctuating market conditions. The findings suggest that AIdriven forecasting models can significantly improve prediction accuracy and enable more dynamic and responsive scenario planning in planning and budgeting systems.

ESP International Journal of Advancements in Computational Technology, 2023
In the current dynamic business environment, the use of AI as part of BI has emerged as a key det... more In the current dynamic business environment, the use of AI as part of BI has emerged as a key determinant of the competitiveness of a firm. This paper is aimed at discussing the possibilities of using AI for the modernization of traditional BI models to support more efficient data analysis, better forecasting, and faster decision-making in organizations. Reviewing the possibilities of AI in BI with a focus on the most essential technologies like machine learning, natural language processing, and predictive analytics, the results of this research demonstrate how the companies might improve operational performance, customer satisfaction, the overall organization's effectiveness implementing AI strategies as a part of BI initiatives. Finally, by the use of case studies and real-life BI applications, we show how radical AI has been in enhancing BI and how it can be implemented in organizations that want to achieve lasting growth and innovation.

ESP International Journal of Advancements in Computational Technology , 2023
In today's world, where the business environment changes every day, AI, together with BI, changes... more In today's world, where the business environment changes every day, AI, together with BI, changes the decisionmaking process. This paper delves into the role and impact AI is having on traditional business analytics through BI that is driven by AI and what new concepts it brings to traditional business analytics, including accurate and real-time insight provision, enabler of predictive analysis and automation of data-intensive tasks. We explore some of the crucial technologies that have enabled this change, such as machine learning, natural language processing and more contemporary data mining methodologies. Also, the paper establishes the advantages of AI-integrated BI, which include efficiency in operations, business advantage, and the fact that they can reveal concealed patterns and trends that are hard to observe using traditional techniques. In this paper, we show how, with the help of AI-driven BI, companies can harness data better, make smarter decisions and attain their goals through case studies and real-life examples. Due to the progressive nature of the entrepreneurship world relying more on data, AI-driven business intelligence is well poised to help drive the emphatic future direction and success.

International Journal of Innovation Research of Science, Engineering and Technology, 2024
The nature of the contemporary financial environment suggests that there has been a relevant nece... more The nature of the contemporary financial environment suggests that there has been a relevant necessity for increasing efficiency and enhancing the accuracy and timeliness of consolidation processes. Some of the conventional consolidation techniques involve several manual steps and different systems for managing the information and thus cannot meet the needs of today's businesses. Oracle Financial Consolidation and Close Cloud Service (FCCS) is presented as a revolutionary tool which can help organizations innovate financial integration procedures, increase the quality of the achieved data, as well as improve the decision-making process. In this paper, the author focuses on the importance of Oracle FCCS in changing the traditional consolidation functions with a look at the challenges that traditional functions have, the main functions of Oracle FCCS, and how the Oracle FCCS helps to overcome those challenges. FCCS can manage multi-GAAP and multi-currency consolidations, actively integrate with other financial systems, and include the financial intelligence subsystem; thus, it complies with the accounting standards that international organizations have developed for the modern enterprise. In addition, this paper provides detailed descriptions of numerous examples of organizations that applied FCCS and obtained enhanced consolidation outcomes. Finally, it looks at what the future holds for financial consolidation and how FCCS is placed to deliver for businesses in a world that will increasingly embrace technology, Artificial Intelligence, machine learning and other related technologies that are bound to define the financial environments of the future more closely.

International Journal of Innovation Research of Science, Engineering and Technology, 2024
Revenue forecasting is a critical function for organizations, facilitating strategic planning and... more Revenue forecasting is a critical function for organizations, facilitating strategic planning and informed decision-making. Traditional forecasting methods often fall short in capturing complex patterns within financial data. Oracle Enterprise Planning and Budgeting Cloud Service (EPBCS) offers robust planning and budgeting capabilities, yet its predictive accuracy can be significantly enhanced by integrating advanced machine learning (ML) techniques. This research investigates the incorporation of ML algorithms into EPBCS to improve revenue forecasting accuracy. We conduct a comprehensive literature review to examine existing methodologies and identify gaps. Based on this, we propose a detailed framework for embedding ML-based predictive models within EPBCS. Our approach includes data preprocessing, feature engineering, model selection, and seamless integration with EPBCS. Preliminary results demonstrate that ML models, particularly ensemble methods, outperform traditional statistical approaches, leading to more accurate and reliable revenue forecasts. This integration promises enhanced decision support and strategic planning capabilities for businesses using Oracle EPBCS.
Conference Presentations by Vikramrajkumar Thiyagarajan
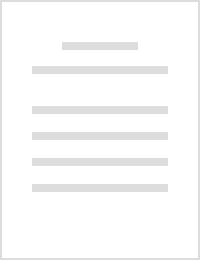
Enhanced Cancer Prediction Using Fine-Tuned CatBoost Model
IEEE, 2024
Cancer prediction using machine learning (ML) models has gained significant attention due to its ... more Cancer prediction using machine learning (ML) models has gained significant attention due to its potential to improve early diagnosis and treatment outcomes. Current models often face challenges such as imbalanced datasets, inadequate feature selection methods, and suboptimal hyperparameter tuning, limiting their predictive accuracy and clinical applicability. To fill in these gaps, this study proposes a fine-tuned CatBoost model that is best for predicting cancer. It uses advanced data processing methods, such as ANOVA-based feature selection, to make sure that the input features are relevant. We benchmark the proposed study against multiple state-of-the-art ML models, including Random Forest, XGBoost, and AdaBoost, to provide a comprehensive performance comparison. By applying meticulous hyperparameter tuning, specifically optimizing parameters like learning rate, depth, and iterations, the CatBoost model significantly improves accuracy, precision, recall, and AUC scores. Results indicate a 0.93 accuracy with a 3% to 5% improvement over traditional models like decision trees and K-nearest neighbors. The CatBoost model exhibits enhanced interpretability through feature importance analysis, making it highly applicable in clinical settings. The fine-tuned CatBoost achieves a 4% increase in precision and a 6% increase in recall compared to baseline models. This demonstrates its robustness and versatility across various datasets. The findings suggest that the proposed approach bridges the identified gaps and offers a reliable, high-performing solution for cancer prediction, outperforming existing models in terms of predictive power and clinical utility.
Uploads
Papers by Vikramrajkumar Thiyagarajan
their predictive accuracy and clinical applicability. To fill in these gaps, this study proposes a fine-tuned CatBoost model that is best for predicting cancer. It uses advanced data processing methods, such as ANOVA-based feature selection, to make sure that the input features are relevant. The proposed study is benchmarked
against multiple state-of-the-art ML models, such as Random Forest, XGBoost, and AdaBoost, providing a comprehensive performance comparison. By applying meticulous hyperparameter tuning, specifically optimizing parameters like learning
rate, depth, and iterations, the CatBoost model significantly improves accuracy, precision, recall, and AUC scores. Results indicate a 0.93 accuracy with a 3% to 5% improvement over traditional models like decision trees and K-nearest neighbors.
The CatBoost model exhibits enhanced interpretability through feature importance analysis, making it highly applicable in clinical settings. The fine-tuned CatBoost achieves a 4% increase in precision and a 6% increase in recall compared to baseline
models. This demonstrates its robustness and versatility across various datasets. The findings suggest that the proposed approach bridges the identified gaps and offers a reliable, high-performing solution for cancer prediction, outperforming existing models in terms of predictive power and clinical utility.
Conference Presentations by Vikramrajkumar Thiyagarajan
their predictive accuracy and clinical applicability. To fill in these gaps, this study proposes a fine-tuned CatBoost model that is best for predicting cancer. It uses advanced data processing methods, such as ANOVA-based feature selection, to make sure that the input features are relevant. The proposed study is benchmarked
against multiple state-of-the-art ML models, such as Random Forest, XGBoost, and AdaBoost, providing a comprehensive performance comparison. By applying meticulous hyperparameter tuning, specifically optimizing parameters like learning
rate, depth, and iterations, the CatBoost model significantly improves accuracy, precision, recall, and AUC scores. Results indicate a 0.93 accuracy with a 3% to 5% improvement over traditional models like decision trees and K-nearest neighbors.
The CatBoost model exhibits enhanced interpretability through feature importance analysis, making it highly applicable in clinical settings. The fine-tuned CatBoost achieves a 4% increase in precision and a 6% increase in recall compared to baseline
models. This demonstrates its robustness and versatility across various datasets. The findings suggest that the proposed approach bridges the identified gaps and offers a reliable, high-performing solution for cancer prediction, outperforming existing models in terms of predictive power and clinical utility.