Papers by Dimitris Kugiumtzis

Topics on Chaotic Systems
In the non-linear analysis of scalar time series the common practice is to reconstruct the state ... more In the non-linear analysis of scalar time series the common practice is to reconstruct the state space using time-delay embedding. When there are more than one observed quantities, one can reconstruct the state space using a time-delay embedding scheme specifying embedding parameters for each quantity. In this study we investigate the state space reconstruction from multiple time series derived from continuous systems and propose a method for building the embedding vector progressively using information measure criteria. The proposed method is compared to other methods with simulations on known chaotic systems, such as individual and coupled Lorenz and Rössler systems. Our analysis showed that multivariate attractor reconstruction preserves better the dynamics of a system and our proposed method gives a parsimonious alternative to the simple extension of the univariate case.
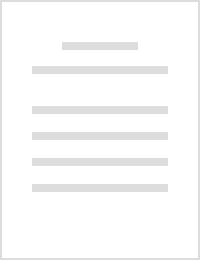
Comparison of Causality Network Estimation in the Sensor and Source Space: Simulation and Application on EEG
Frontiers in Network Physiology
The usage of methods for the estimation of the true underlying connectivity among the observed va... more The usage of methods for the estimation of the true underlying connectivity among the observed variables of a system is increasing, especially in the domain of neuroscience. Granger causality and similar concepts are employed for the estimation of the brain network from electroencephalogram (EEG) data. Also source localization techniques, such as the standardized low resolution electromagnetic tomography (sLORETA), are widely used for obtaining more reliable data in the source space. In this work, connectivity structures are estimated in the sensor and in the source space making use of the sLORETA transformation for simulated and for EEG data with episodes of spontaneous epileptiform discharges (ED). From the comparative simulation study on high-dimensional coupled stochastic and deterministic systems originating in the sensor space, we conclude that the structure of the estimated causality networks differs in the sensor space and in the source space. Moreover, different network typ...
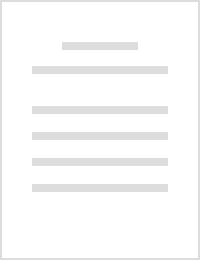
Surrogate Data Test on Time Series
Modelling and Forecasting Financial Data
ABSTRACT Given a real random-like time series, the first question to answer is whether the data c... more ABSTRACT Given a real random-like time series, the first question to answer is whether the data carry any information over time, i.e. whether the successive samples are correlated. Using standard statistical testing, the least interesting null hypothesis of white noise has to be rejected if the analysis of the time series should be of any use at all. Further, if nonlinear methods are to be used, e.g. a sophisticated nonlinear prediction method instead of a linear autoregressive model, the null hypothesis to be rejected is that the data involve only temporal linear correlations and are otherwise random. A statistically rigorous framework for such tests is provided by the method of surrogate data. The surrogate data, generated to represent the null hypothesis, are compared to the original data under a nonlinear discriminating statistic in order to reject or approve the null hypothesis. The surrogate data test for nonlinearity has become popular in the last years, especially with regard to the null hypothesis that the examined time series is generated by a Gaussian (linear) process undergoing a possibly nonlinear static transform. Properly designed surrogate data for this null hypothesis should possess the same autocorrelation and amplitude distribution as the original data and be otherwise random. However, the algorithms do not always provide surrogate data that preserve the original linear correlations, and this can lead to false rejections. The rejection of the null hypothesis may also depend on the applied nonlinear method and the choice of the method’s parameters. Also, different observed time series from the same system may give different test results. This chapter will describe the surrogate data test for the two hypotheses, i.e. white noise data and linear stochastic data. For the latter, some of the limitations and caveats of the test will be discussed and techniques to improve the robustness and reliability of the test will be reviewed. Finally, the tests will be applied to some financial data sets.

Brain Sciences
Aim: To investigate for the first time the brain network in the Alzheimer’s disease (AD) spectrum... more Aim: To investigate for the first time the brain network in the Alzheimer’s disease (AD) spectrum by implementing a high-density electroencephalography (HD-EEG - EGI GES 300) study with 256 channels in order to seek if the brain connectome can be effectively used to distinguish cognitive impairment in preclinical stages. Methods: Twenty participants with AD, 30 with mild cognitive impairment (MCI), 20 with subjective cognitive decline (SCD) and 22 healthy controls (HC) were examined with a detailed neuropsychological battery and 10 min resting state HD-EEG. We extracted correlation matrices by using Pearson correlation coefficients for each subject and constructed weighted undirected networks for calculating clustering coefficient (CC), strength (S) and betweenness centrality (BC) at global (256 electrodes) and local levels (29 parietal electrodes). Results: One-way ANOVA presented a statistically significant difference among the four groups at local level in CC [F (3, 88) = 4.76, p...

Entropy
Granger causality and variants of this concept allow the study of complex dynamical systems as ne... more Granger causality and variants of this concept allow the study of complex dynamical systems as networks constructed from multivariate time series. In this work, a large number of Granger causality measures used to form causality networks from multivariate time series are assessed. These measures are in the time domain, such as model-based and information measures, the frequency domain, and the phase domain. The study aims also to compare bivariate and multivariate measures, linear and nonlinear measures, as well as the use of dimension reduction in linear model-based measures and information measures. The latter is particular relevant in the study of high-dimensional time series. For the performance of the multivariate causality measures, low and high dimensional coupled dynamical systems are considered in discrete and continuous time, as well as deterministic and stochastic. The measures are evaluated and ranked according to their ability to provide causality networks that match th...

Medical & Biological Engineering & Computing
Transcranial magnetic stimulation combined with electroencephalography (TMS-EEG) is widely used t... more Transcranial magnetic stimulation combined with electroencephalography (TMS-EEG) is widely used to study the reactivity and connectivity of brain regions for clinical or research purposes. The electromagnetic pulse of the TMS device generates at the instant of administration an artifact of large amplitude and a duration up to tens of milliseconds that overlaps with brain activity. Methods for TMS artifact correction have been developed to remove the artifact and recover the underlying, immediate response of the cerebral cortex to the magnetic stimulus. In this study, three such algorithms are evaluated. Since there is no ground truth for the masked brain activity, pilot data formed from the superposition of the isolated TMS artifact on the EEG brain activity are used to evaluate the performance of the algorithms. Different scenarios of TMS-EEG experiments are considered for the evaluation: TMS at resting state, TMS inducing epileptiform discharges and TMS administered during epileptiform discharges. We show that a proposed gap filling method is able to reproduce qualitative characteristics and in many cases closely resemble the hidden EEG signal. Finally, shortcomings of the TMS correction algorithms as well as the pilot data approach are discussed.
Testing the randomness of correlation networks from multivariate time series
Journal of Complex Networks

Computational intelligence and neuroscience, 2018
Brain-Computer Interface (BCI) is a rapidly developing technology that aims to support individual... more Brain-Computer Interface (BCI) is a rapidly developing technology that aims to support individuals suffering from various disabilities and, ultimately, improve everyday quality of life. Sensorimotor rhythm-based BCIs have demonstrated remarkable results in controlling virtual or physical external devices but they still face a number of challenges and limitations. Main challenges include multiple degrees-of-freedom control, accuracy, and robustness. In this work, we develop a multiclass BCI decoding algorithm that uses electroencephalography (EEG) source imaging, a technique that maps scalp potentials to cortical activations, to compensate for low spatial resolution of EEG. Spatial features were extracted using Common Spatial Pattern (CSP) filters in the cortical source space from a number of selected Regions of Interest (ROIs). Classification was performed through an ensemble model, based on individual ROI classification models. The evaluation was performed on the BCI Competition IV...
Financial networks based on Granger causality: A case study
Physica A: Statistical Mechanics and its Applications
Discrimination of coupling structures using causality networks from multivariate time series
Chaos: An Interdisciplinary Journal of Nonlinear Science
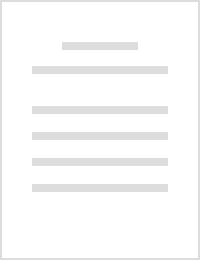
The Concept of Effective Inflow: Application to Interictal Localization of the Epileptogenic Focus from iEEG
IEEE transactions on bio-medical engineering, Sep 29, 2016
Accurate determination of the epileptogenic focus is of paramount diagnostic and therapeutic impo... more Accurate determination of the epileptogenic focus is of paramount diagnostic and therapeutic importance in epilepsy. The current gold standard for focus localization is from ictal (seizure) onset and thus requires the occurrence and recording of multiple typical seizures of a patient. Localization of the focus from seizure-free (interictal) periods remains a challenging problem, especially in the absence of interictal epileptiform activity. By exploring the concept of effective inflow, we developed a focus localization algorithm (FLA) based on directed connectivity between brain sites. Subsequently, using the measure of generalized partial directed coherence (GPDC) over a broad frequency band in FLA for the analysis of interictal periods from long-term (days) intracranial electroencephalographic (iEEG) signals, we identified the brain region that is the most frequent receiver of maximal effective inflow from other brain regions. In six out of nine patients with temporal lobe epileps...

Frontiers in Physiology, 2016
Removable complete and partial dentures are supported by the residual alveolar ridges consisting ... more Removable complete and partial dentures are supported by the residual alveolar ridges consisting of mucosa, submucosa, periosteum, and bone. An understanding of the biomechanical behavior of the oral mucosa is essential in order to improve the denture-bearing foundations for complete and partially edentulous patients. The purpose of this paper was to examine the biomechanical behavior of the soft tissues supporting a removable denture and develop a model for that reason. Keratinized oral mucosa blocks with their underlying bone were harvested from the maxillary palatal area adjacent to the edentulous ridges of a cadaver. The compressive response of the oral mucosa was tested by using atomic force microscopy. The specimens were first scanned in order their topography to be obtained. The mechanical properties of the specimens were tested using a single crystal silicon pyramidal tip, which traversed toward the keratinized oral mucosa specimens. Loading-unloading cycles were registered and four mathematical models were tested using MATLAB to note which one approximates the force-displacement curve as close as possible: a. spherical, b. conical, c. third order polynomial, d. Murphy (fourth order polynomial, non-linear Hertzian based). The third order polynomial model showed the best accuracy in representing the force-displacement data of the tested specimens. A model was developed in order to analyze the biomechanical behavior of the human oral keratinized mucosa and obtain information about its mechanical properties.

Physica D: Nonlinear Phenomena, 1997
Many methods dealing with the analysis of multivariate data involve computations of point interdi... more Many methods dealing with the analysis of multivariate data involve computations of point interdistances in state space. In time series analysis, the points are reconstructed from scalar data, most commonly using the method of delay coordinates. While, theoretically, all norms are considered equivalent in measuring interdistances, they perform differently in the presence of noise. The statistical description of three of the most popular norms, L 1, L2, and L~, revealed certain shortcomings of each, depending on the corresponding noise-free interdistances. For chaotic time series, the effect of noise on the different measures also varies with the reconstruction. Estimating the correlation dimension for simulated noisy data using the three norms confirmed the statistical analysis. Generally, the L2 norm turns out to be the most robust over a range of time series types and reconstructions, whereas the L1 and L~c norms seem to deteriorate markedly for specific types of systems or reconstructions. Furthermore, the investigation gave some more insight into the role of the reconstruction parameters, showing in particular, the importance of the time window length rw.
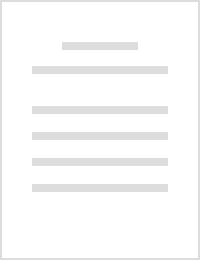
Directed coupling measures for interhemisheric information flow from epileptic EEG
ABSTRACT The aim of this work is to evaluate two directed coupling measures in determining interh... more ABSTRACT The aim of this work is to evaluate two directed coupling measures in determining interhemisheric information flow from electroencephalograms (EEG) of epileptic patients. In particular, the frontal, central and parietal cortices are considered. The first measure is the directed coherence, a linear measure defined in the frequency domain, and the second the transfer entropy, a nonlinear information measure defined in the time domain. The two measures are computed on consecutive EEG segments from each pair of channels at the different brain areas over a time period that covers the preictal, ictal and postictal state. The profiles of the two measures over the recording period are obtained for 8 extracranial epileptic EEG records, 7 of general tonic clonic type and one of temporal lobe type, all from different patients. Discrimination of the preictal state from postictal state could be established in almost all episodes by transfer entropy and in only few with the directed coherence. The results for the direction of the causal effects were not conclusive as the measures indicated both similar and opposite causal effects.

In the nonlinear prediction of scalar time series, the common practice is to reconstruct the stat... more In the nonlinear prediction of scalar time series, the common practice is to reconstruct the state space using time-delay embedding and apply a local model on neighborhoods of the reconstructed space. The method of false nearest neighbors is often used to estimate the embedding dimension. For prediction purposes, the optimal embedding dimension can also be estimated by some prediction error minimization criterion. We investigate the proper state space reconstruction for multivariate time series and modify the two abovementioned criteria to search for optimal embedding in the set of the variables and their delays. We pinpoint the problems that can arise in each case and compare the state space reconstructions (suggested by each of the two methods) on the predictive ability of the local model that uses each of them. Results obtained from Monte Carlo simulations on known chaotic maps revealed the non-uniqueness of optimum reconstruction in the multivariate case and showed that prediction criteria perform better when the task is prediction.
In the prediction of oscillating time series, the interest is in the turning points of successive... more In the prediction of oscillating time series, the interest is in the turning points of successive oscillations rather than the samples themselves. For this purpose a scheme has been proposed; the state space reconstruction is limited to the turning points and the local (nearest neighbor) model is modified in order to predict the turning point magnitudes and times. This approach
Small-world property and distinct evolution of complex networks from historical earthquakes in Greece
ABSTRACT
Testing the randomness of causality networks from multivariate time series
ABSTRACT
ΕΛΕΓΧΟΣ ΤΥΧΑΙΟΤΗΤΑΣ ΔΙΚΤΥΩΝ ΣΥΣΧΕΤΙΣΗΣ ΑΠΟ ΠΟΛΥΜΕΤΑΒΛΗΤΕΣ ΧΡΟΝΟΣΕΙΡΕΣ
ABSTRACT
Causality networks from multivariate time series and application to epilepsy
2015 37th Annual International Conference of the IEEE Engineering in Medicine and Biology Society (EMBC), 2015
Uploads
Papers by Dimitris Kugiumtzis